Detection of Smartphone Permissioned Security Breaches using Machine Learning Techniques
2023 World Conference on Communication & Computing (WCONF)(2023)
摘要
Attackers may target cell phones by taking advantage of the rights given to installed applications. Users frequently have to provide application permission when they download and install it on their smartphones. This experimental study concentrates on the identification of permissioned malware assaults in smartphone security breaches using machine learning approaches. In order to uncover security flaws in smartphones, the study compares the effectiveness of three widely used machine learning algorithms: Naive Bayes, K-nearest neighbor, and Decision Tree. Each method was trained and evaluated using the pre-processed data and then divided into training, and testing sets. The study evaluated trained and tested algorithm’s accuracy, precision, recall, and F1 score so that machine learning techniques can be deployed for detecting permissioned malware attack. The results prove that the technique Decision Tree Classifier is performing overall better in comparison with other chosen techniques for spotting security flaws in cellphones. Performance indicators for Naive Bayes were lower than those for the other two algorithms. This study offers insightful information about how well machine learning algorithms can execute early detection of permissioned malware attacks on smartphones, and it can be utilized as a model for creating secure smartphone defenses.
更多查看译文
关键词
Permissioned Malware Detection,Random Forest,Malicious Attack,Decision Tree,K-Nearest Neighbors,Smartphones,Security
AI 理解论文
溯源树
样例
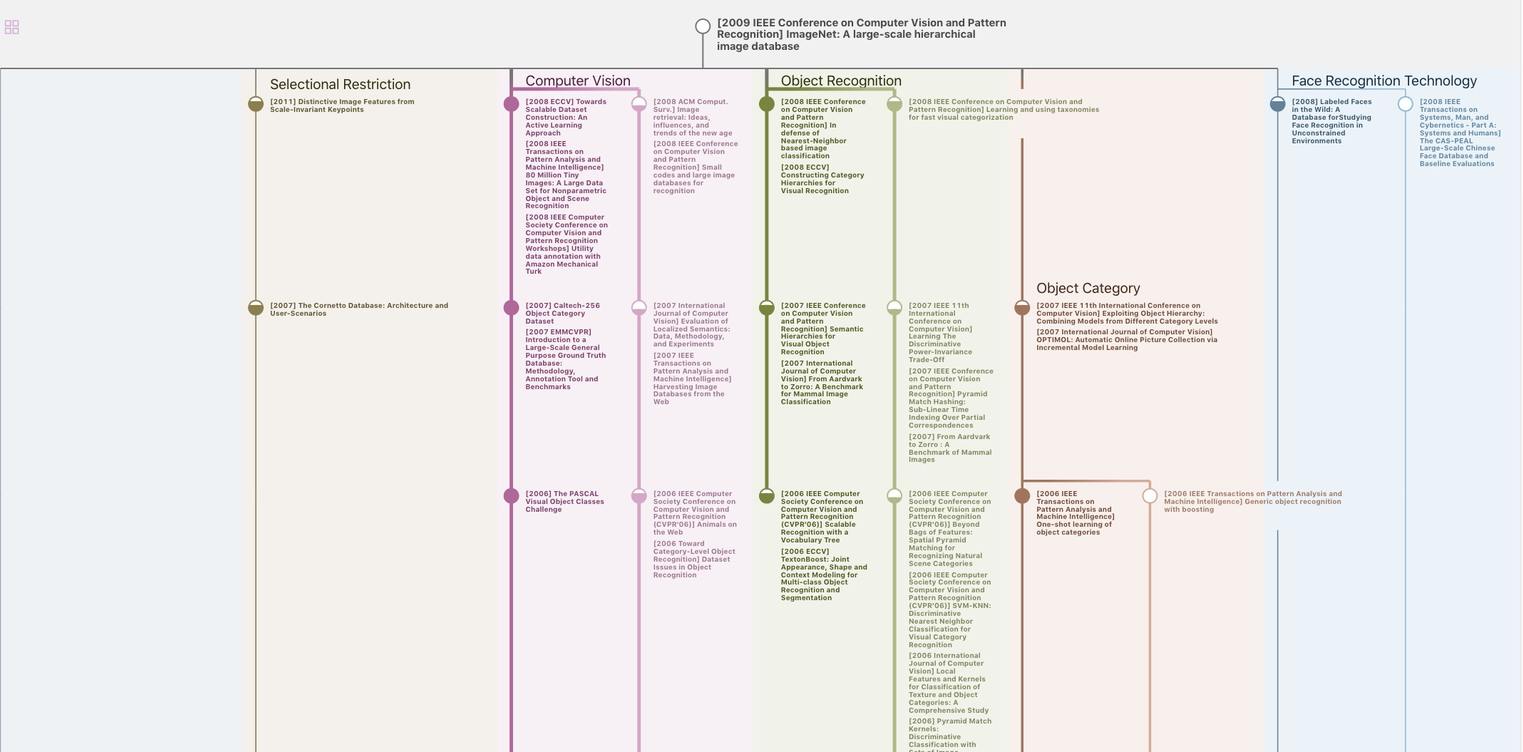
生成溯源树,研究论文发展脉络
Chat Paper
正在生成论文摘要