A Stable and Scalable Digital Composite Neurocognitive Test for Early Dementia Screening Based on Machine Learning: Model Development and Validation Study
JOURNAL OF MEDICAL INTERNET RESEARCH(2023)
摘要
Background: Dementia has become a major public health concern due to its heavy disease burden. Mild cognitive impairment (MCI) is a transitional stage between healthy aging and dementia. Early identification of MCI is an essential step in dementia prevention. Objective: Based on machine learning (ML) methods, this study aimed to develop and validate a stable and scalable panel of cognitive tests for the early detection of MCI and dementia based on the Chinese Neuropsychological Consensus Battery (CNCB) in the Chinese Neuropsychological Normative Project (CN-NORM) cohort. Methods: CN-NORM was a nationwide, multicenter study conducted in China with 871 participants, including an MCI group (n=327, 37.5%), a dementia group (n=186, 21.4%), and a cognitively normal (CN) group (n=358, 41.1%). We used the following 4 algorithms to select candidate variables: the F-score according to the SelectKBest method, the area under the curve (AUC) from logistic regression (LR), P values from the logit method, and backward stepwise elimination. Different models were constructed after considering the administration duration and complexity of combinations of various tests. Receiver operating characteristic curve and AUC metrics were used to evaluate the discriminative ability of the models via stratified sampling cross-validation and LR and support vector classification (SVC) algorithms. This model was further validated in the Alzheimer'sDisease Neuroimaging Initiative phase 3 (ADNI-3) cohort (N=743), which included 416 (56%) CN subjects, 237 (31.9%) patients with MCI, and 90 (12.1%) patients with dementia. Results: Except for social cognition, all other domains in the CNCB differed between the MCI and CN groups (P<.008). In feature selection results regarding discrimination between the MCI and CN groups, the Hopkins Verbal Learning Test-5 minutes Recall had the best performance, with the highest mean AUC of up to 0.80 (SD 0.02) and an F-score of up to 258.70. The scalability of model 5 (Hopkins Verbal Learning Test-5 minutes Recall and Trail Making Test-B) was the lowest. Model 5 achieved a higher level of discrimination than the Hong Kong Brief Cognitive test score in distinguishing between the MCI and CN groups (P<.05). Model 5 also provided the highest sensitivity of up to 0.82 (range 0.72-0.92) and 0.83 (range 0.75-0.91) according to LR and SVC, respectively. This model yielded a similar robust discriminative performance in the ADNI-3 cohort regarding differentiation between the MCI and CN groups, with a mean AUC of up to 0.81 (SD 0) according to both LR and SVC algorithms. Conclusions: We developed a stable and scalable composite neurocognitive test based on ML that could differentiate not only between patients with MCI and controls but also between patients with different stages of cognitive impairment. This composite neurocognitive test is a feasible and practical digital biomarker that can potentially be used in large-scale cognitive screening and intervention studies.
更多查看译文
关键词
mild cognitive impairment,digital cognitive assessment,machine learning,neurocognitive test,cognitive screening,dementia
AI 理解论文
溯源树
样例
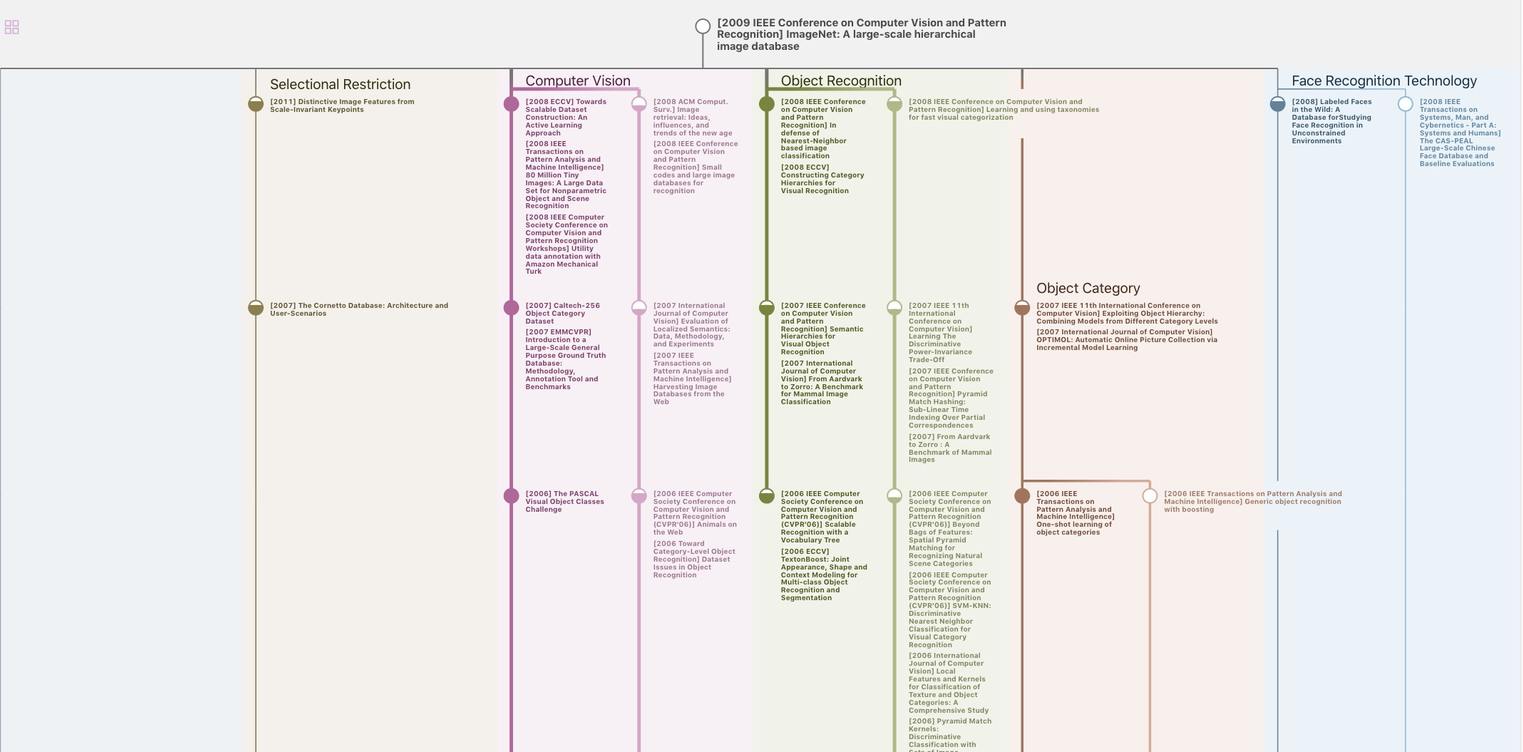
生成溯源树,研究论文发展脉络
Chat Paper
正在生成论文摘要