MOC: Multi-Objective Mobile CPU-GPU Co-optimization for Power-efficient DNN Inference
2023 IEEE/ACM INTERNATIONAL CONFERENCE ON COMPUTER AIDED DESIGN, ICCAD(2023)
Abstract
With the emergence of DNN applications on mobile devices, plenty of attention has been attracted to their optimization. However, the impact of DNN inference tasks on device power consumption is still a lack of comprehensive study. In this work, we propose MOC, a Multi-Objective deep reinforcement learning-assisted DNN inference stage-adaptive CPU-GPU Co-optimization approach. We find through experiments that CPU-GPU parameters, including CPU core, CPU, and GPU frequency, could significantly impact the speed and power consumption of DNN inference. We empirically analyze various stages of DNN inference, including pre/post-processing and feed-forward calculating stages. Based on the analysis, a DNN demand-resource matching model is proposed to classify the DNNs into various categories. Next, a multi-objective deep reinforcement learning (MODRL)-assisted framework is proposed, which considers both the DNN type and hardware environment, to make decisions on DNN inference stage-adaptive CPU/GPU parameter tuning. Finally, a rule-based action refinement technique is introduced to tailor the search space of MOC. Extensive experiments show that, compared with existing works, MOC could substantially reduce the power consumption of DNN inference tasks by up to 74.4%, meanwhile delivering an excellent speed on mobile devices.
MoreTranslated text
AI Read Science
Must-Reading Tree
Example
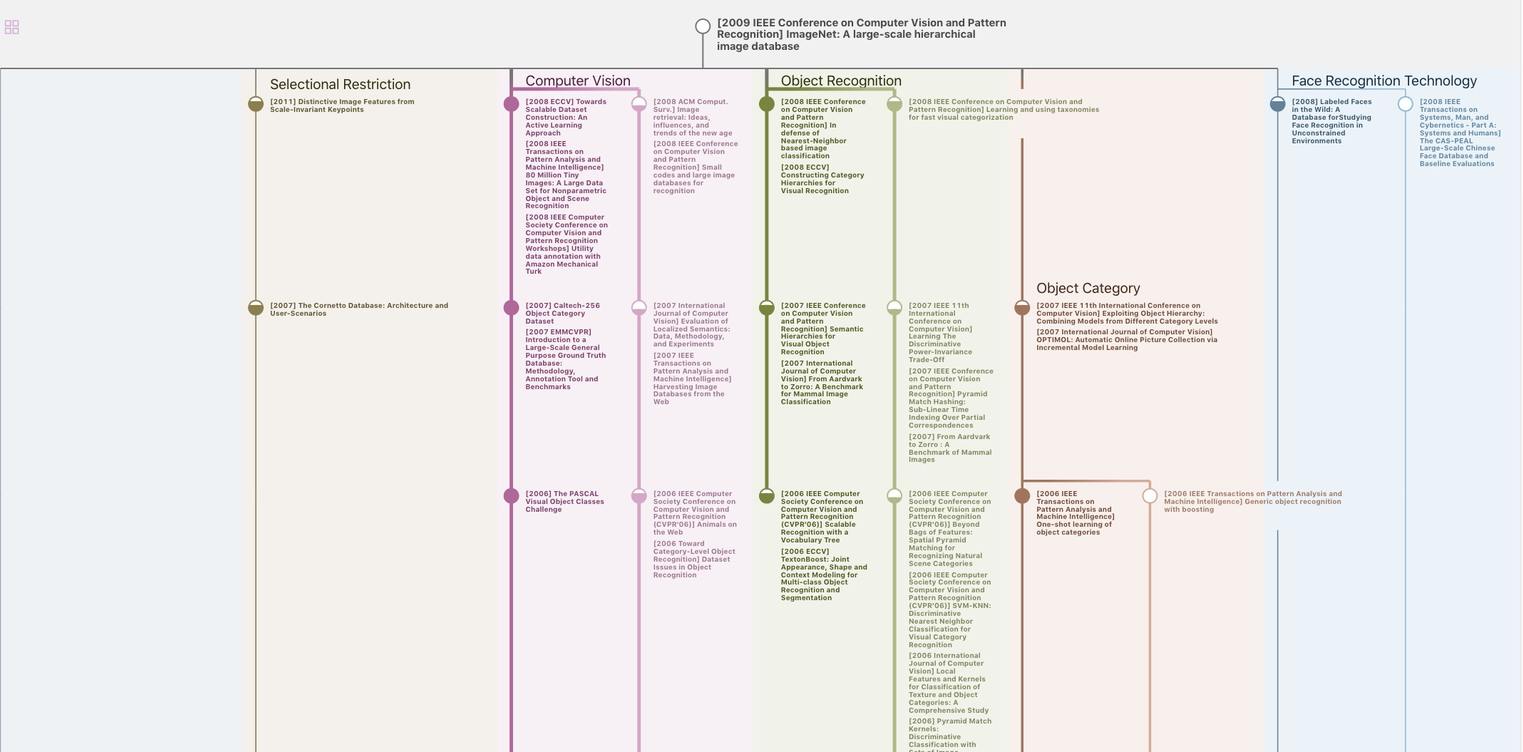
Generate MRT to find the research sequence of this paper
Chat Paper
Summary is being generated by the instructions you defined