A Generic Postmethod for Blind Source Separation to Purify Abundance Map
IEEE SENSORS JOURNAL(2023)
摘要
There are many mixed pixels in a hyperspectral image (HI), and blind hyperspectral unmixing (BHU) is employed to decompose these mixed pixels into spectra and corresponding fractional coefficients, respectively, termed endmembers and abundances. Although the de facto applications usually need pure abundances, the existing methods often fail to provide pure abundance maps due to the natural distribution of the earth's surface substances; moreover, HI is easily affected by different interferences. These detrimental factors lead to a generation of redundancy for the extracted feature (endmember/abundance). Because of the redundancy, the substances are difficult to distinguish, and some parts of an abundance map are inclined to disappear or erroneously appear in the other abundance map. As a result, the accuracy of the estimated abundances could be compromised. To improve the discriminations among different substances, we design a BHU method and exploit an additional independent redundancy-removing method, which can punish the redundancy among the extracted endmembers. Different from other BHU methods, the presented method can considerably reduce the redundancy among the reconstructions from the global perspective and significantly improve the discriminations among different substances, especially, the exact endmembers and purer abundances are expected to be obtained. A wide range of experiments are conducted on both synthetic and benchmark real datasets to demonstrate the superiority of the advocated frameworks.
更多查看译文
关键词
Adverse factors,correlation,hyperspectral unmixing,remove redundancy,statistical distribution
AI 理解论文
溯源树
样例
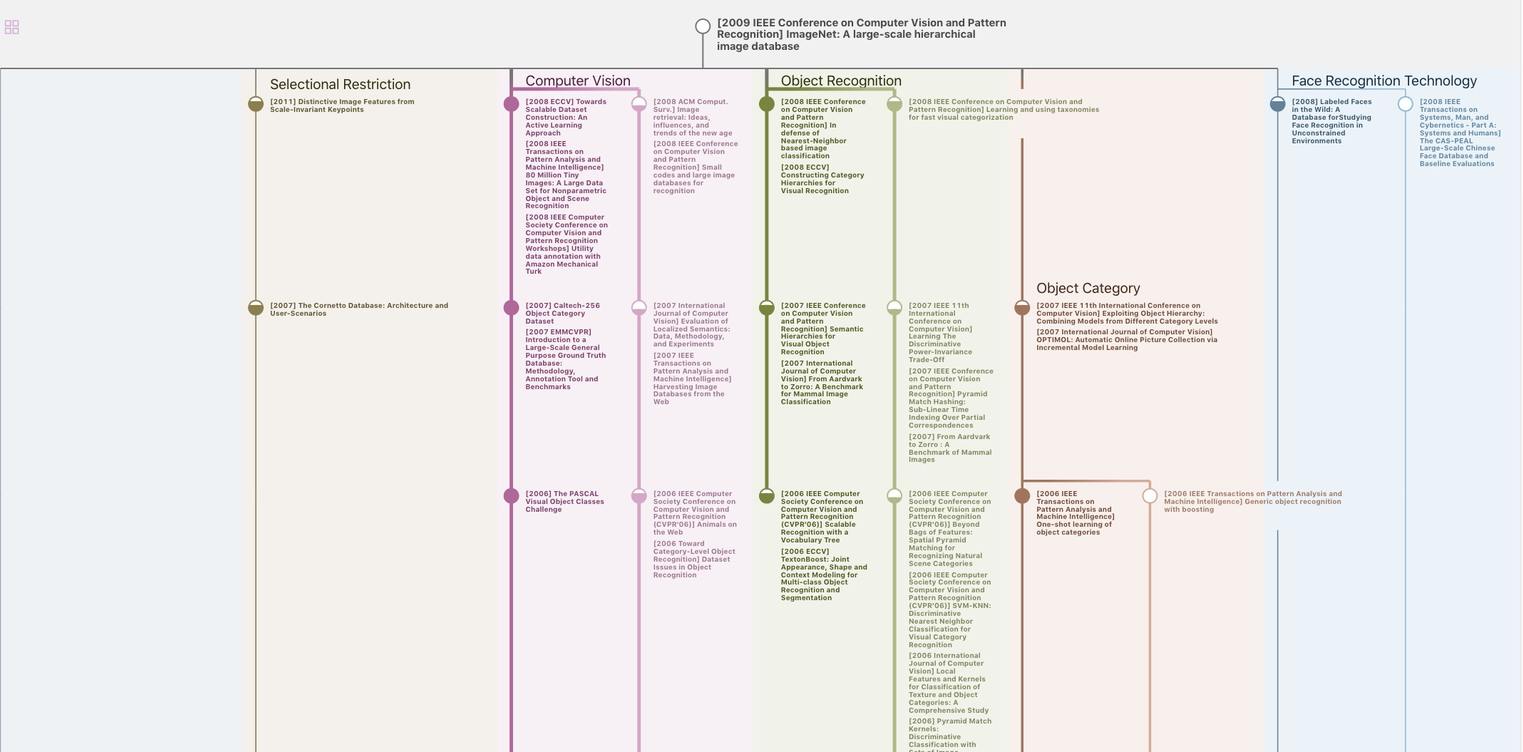
生成溯源树,研究论文发展脉络
Chat Paper
正在生成论文摘要