Multilevel Decomposition Time Aggregation Graph Convolution Networks for Skeleton-Based Action Recognition
2023 IEEE International Conference on Mechatronics and Automation (ICMA)(2023)
摘要
Skeleton-based human action recognition has become a popular topic among researchers. This is because using skeletal data provides a robust solution to problems encountered in complex environments, such as changes in perspective and background interference. The robustness of skeletal data enables recognition methods to focus on more specific and relevant features. We propose a model called multilevel decomposition time aggregation graph convolution network (MDT-GCN), which utilizes a multilevel graph convolution kernel to capture higher-order spatial dependence relationships between joints. This is achieved by decomposing a human topology graph into smaller graphs, each of which has its own graph convolution kernel. To further enhance the performance of our model, we employ a two-flow framework and channel topology refinement strategy. Our experiments on the NTU-RGB+D60 and NTU-RGB+D120 datasets demonstrate that our MDT-GCN network outperforms the previous algorithm and significantly improves the accuracy of action recognition.
更多查看译文
关键词
Skeleton-based data,Action recognition,Spatio-temporal graph,Temporal attention
AI 理解论文
溯源树
样例
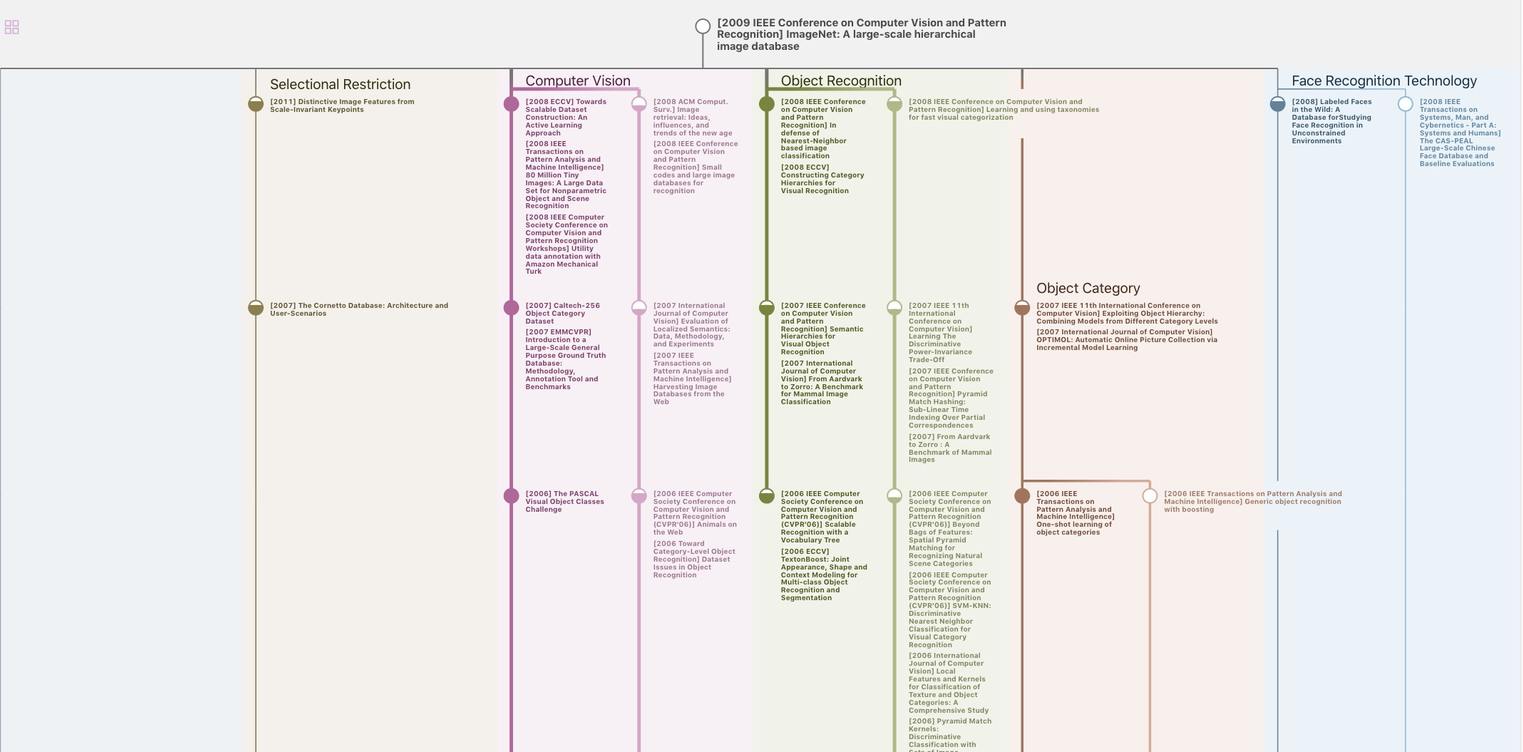
生成溯源树,研究论文发展脉络
Chat Paper
正在生成论文摘要