A Machine Learning-Powered Web Application for Accident Severity Detection
2023 5th International Conference on Inventive Research in Computing Applications (ICIRCA)(2023)
摘要
The severity of road accidents has been a major concern for transportation safety. Despite various efforts to reduce accident rates, the inability to accurately predict and detect the severity of accidents has impeded progress. In recent years, machine learning (ML) techniques have been explored to detect the severity of accidents, enabling timely intervention by emergency services and reducing the mortality rate. This paper presents a comprehensive review of the application of ML techniques in detecting accident severity. Five different machine learning algorithms were used: Random forest classifier, XGBoost, Logistic regression, CatBoost and Support vector machines. Two deep learning models, the Multi-layer Perceptron (MLP) and Convolutional Neural Network (CNN), were utilized to compare their performances. A dataset on road accidents published by the Leeds City Council was used for training the models. This dataset consists of 2605 data points categorized under three classes: slight, severe, and fatal. The chi-squared test and mutual information feature selection techniques were used to determine which features have the highest influence on prediction. A comparison was made between the accuracies obtained from using the dataset with feature selection and the dataset without feature selection. A web application was developed that can be used by the user to predict the severity of the accident in real time. This web application would help the emergency workers to get an early knowledge about the severity of the accident and to plan their course of actions accordingly. It was observed that XGBoost had the highest accuracy with 95.036%.
更多查看译文
关键词
Machine learning,Accident severity,Prediction,Web application,Feature selection,Deep learning
AI 理解论文
溯源树
样例
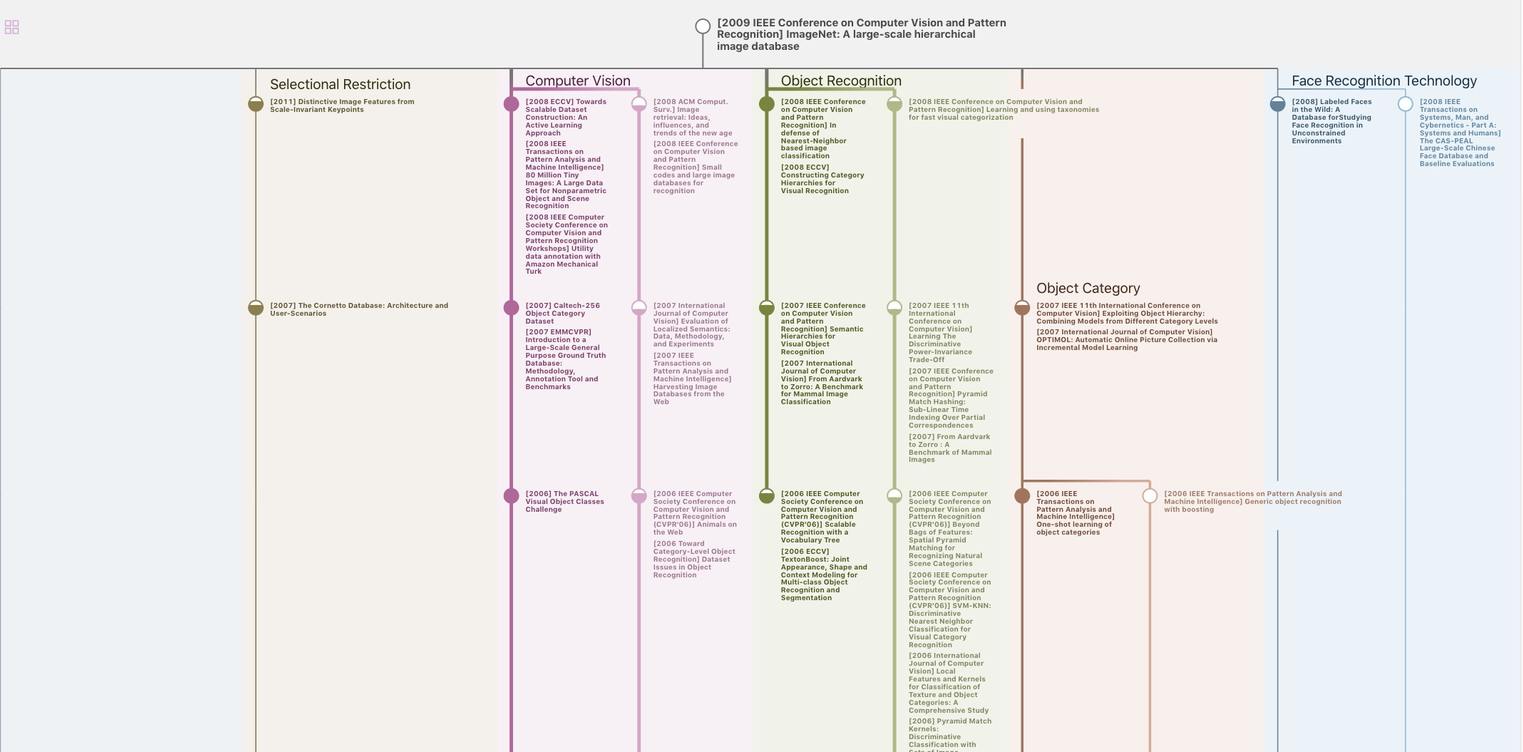
生成溯源树,研究论文发展脉络
Chat Paper
正在生成论文摘要