A Dynamic Feature Regression Network for Industrial Soft Sensor Modeling
2023 42nd Chinese Control Conference (CCC)(2023)
摘要
Soft sensor has been extensively applied for online estimation of the key quality variables in modern industrial processes, which is extremely important for the process to achieve efficient monitoring and smooth control. To build an accurate data-driven model, the dynamic correlation and strong nonlinearity of process sequential data must be considered in soft sensor modeling. Therefore, a dynamic feature regression network (DFR) is proposed in this paper for industrial soft sensor modeling, consisting of a dynamic feature extraction network and a feature regression network to explore different industrial data features. First, the dynamic feature extraction network maps time series samples to a set of hidden dynamic features, while the feature regression network performs deeper feature extraction and output regression on key quality variables. Furthermore, since both networks consist of unsupervised feature extraction and supervised feature regression, a large scale of unlabeled samples can be utilized in the semisupervised learning of model parameters. Finally, the feasibility and efficacy of the proposed model are verified through the coal-to-ethylene glycol process data to predict the carbon monoxide content.
更多查看译文
关键词
Autoencoder network (AE),long short-term memory network (LSTM),Convolutional neural network (CNN),feature extraction,semisupervised learning,soft sensor
AI 理解论文
溯源树
样例
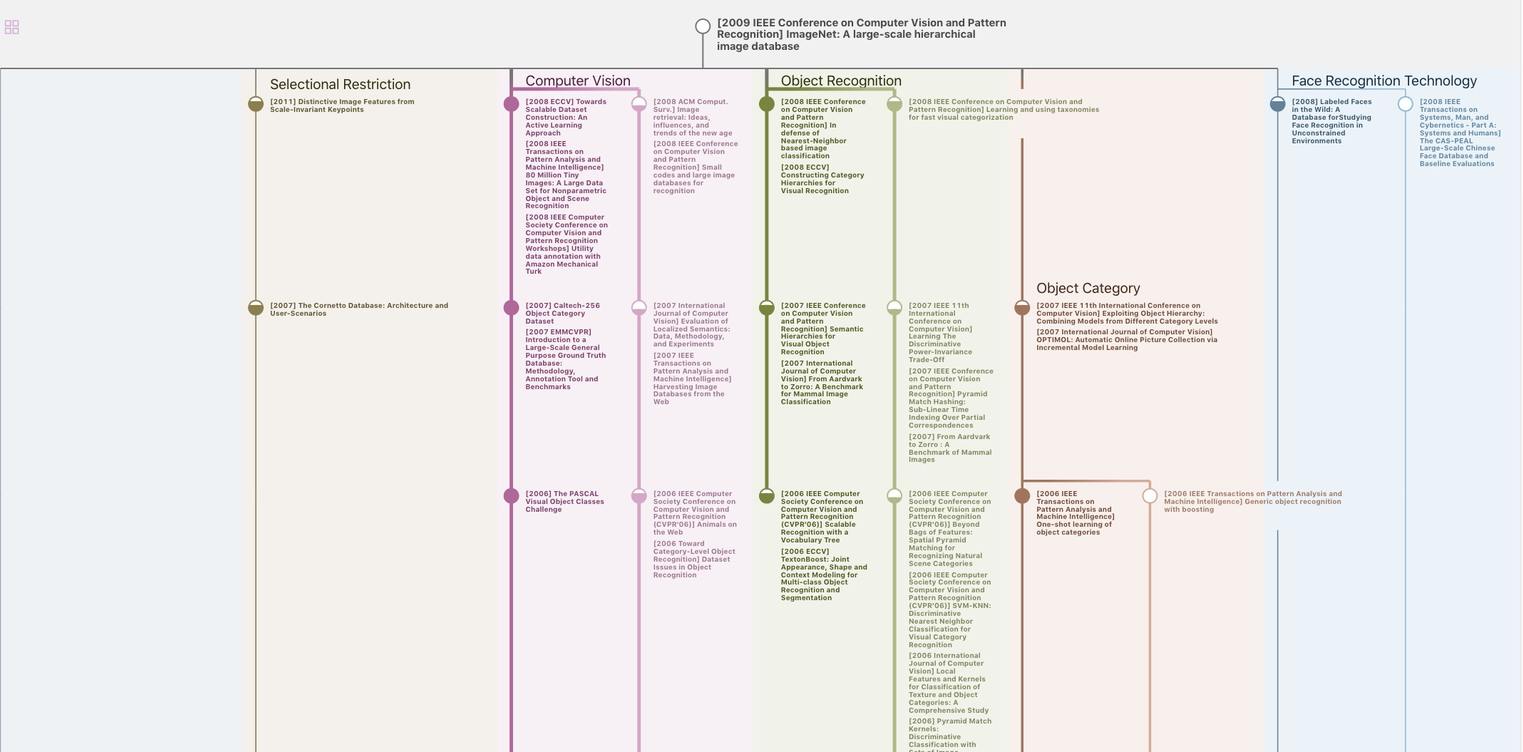
生成溯源树,研究论文发展脉络
Chat Paper
正在生成论文摘要