Multi-Scale Variational Graph AutoEncoder for Graph Disentanglement Learning
2023 IEEE 18th Conference on Industrial Electronics and Applications (ICIEA)(2023)
摘要
Graph Convolutional Neural Networks are serving as a common network to learn complex relationships and extract deep features for downstream tasks. The existing graph-based autoencoders take an encoder with fixed adjacency matrix to learn latent representations in the real graph and adopt a decoder to reconstruct the adjacency matrix for self-supervised learning. However, the relations are heterogeneous in the real world, but entangled together because of many latent factors. In this paper, we propose a novel graph framework, termed multi-scale variational graph autoencoder for graph disentanglement learning, which disentangles the input graph into several factor graphs and learns latent representations. Then, we present a decoder to infer mixed relations from latent representations. In addition, we introduce β-VAE framework to maintain diversity of latent representations. Generally, different metrics demonstrate our model’s advantaged performance on both downstream tasks and graph-level disentanglement tasks. Our model outperforms the state-of-the-art methods, especially on the ZINC dataset and other datasets, for example those related to bioinformatics graphs, which are featured by practical node representations.
更多查看译文
关键词
graph convolutional neural network,graph autoencoder,self-supervised learning,graph disentangled learning
AI 理解论文
溯源树
样例
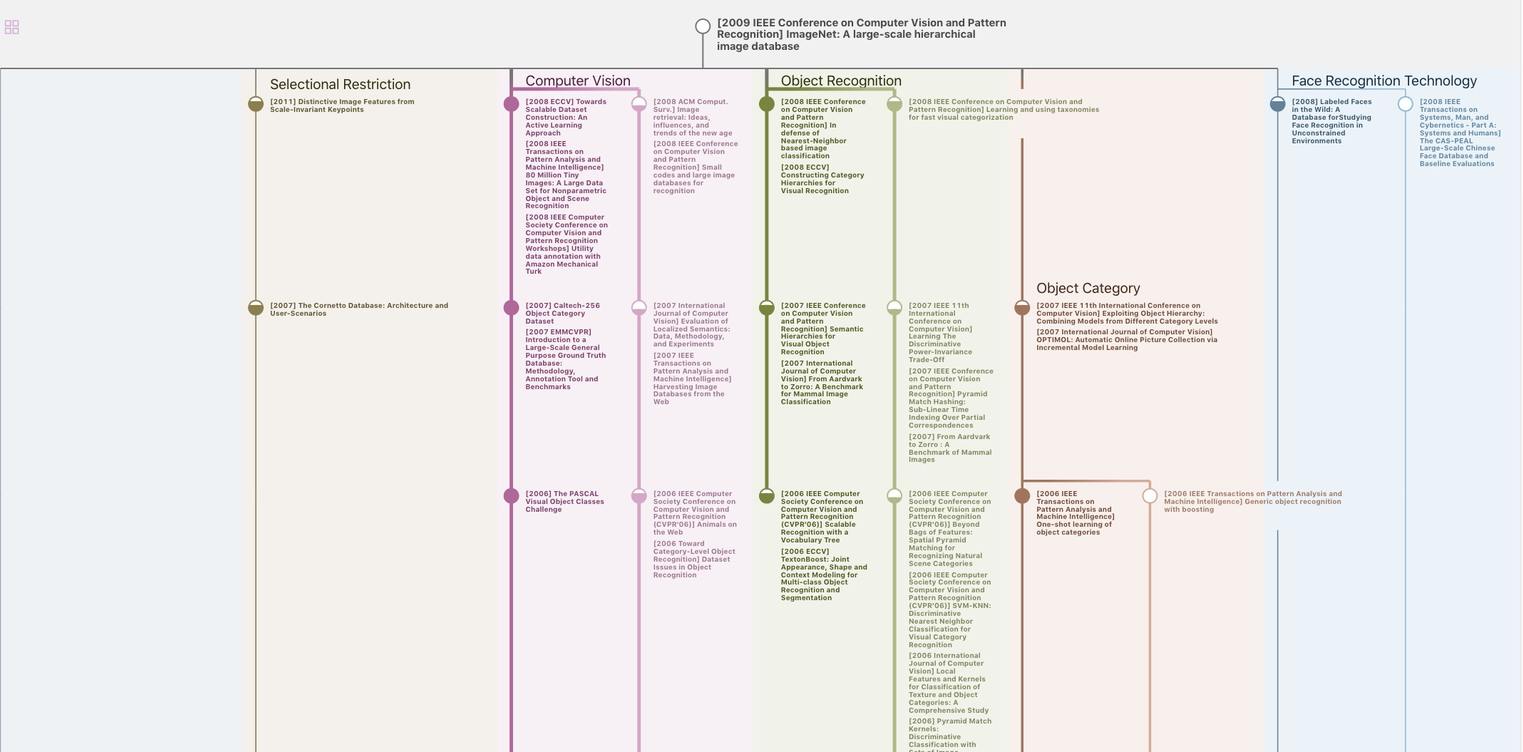
生成溯源树,研究论文发展脉络
Chat Paper
正在生成论文摘要