Energy Value Estimation of Acoustic Emission Signal Using Advanced Machine Learning Algorithms within Composite Specimen Application
2022 International Conference on Electrical, Computer, Communications and Mechatronics Engineering (ICECCME)(2022)
摘要
Composites, in the industry, are substituting metal parts in order to reduce the weight of the structures and due to their properties such as stiffness, strength, and others. Acoustic emission (AE) is a well-known and appropriate method for monitoring structures for defects, and it can be successfully applied to composite parts of a structure. Machine learning can be employed in order to automate the process of defect detection based on different metrics. The signal energy is a vital metric that can be utilised to show whether defects exist in composite specimens. In this paper, a dataset is analysed into features and it gets proliferated using a synthesis method based on differential privacy. Thereafter, the dataset is fed into two advanced machine learning algorithms, namely the Convolutional Neural Networks (CNN) and Long Short-Term Memory (LSTM) neural networks. The results of the CNN show the test Mean-Squared Error (MSE) and Training MSE, which are calculated, showing satisfactory results for the defect and energy, respectively. Moreover, the energy values can be quite accurately predicted using LSTM; the process indicates that defects can be identified in a composite specimen using only the energy of the signal.
更多查看译文
关键词
acoustic emission signal,advanced machine learning algorithms,acoustic emission,composite specimen application,machine learning
AI 理解论文
溯源树
样例
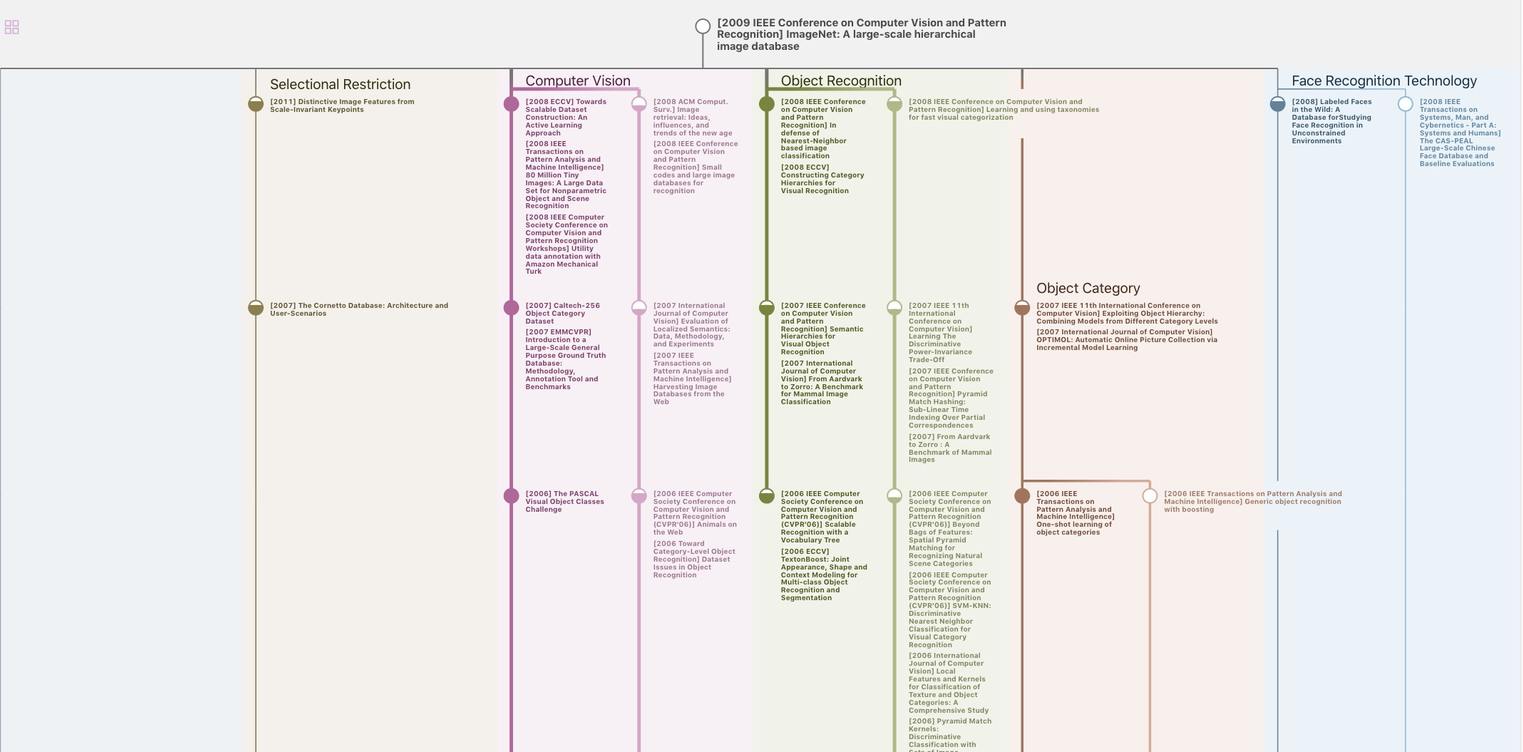
生成溯源树,研究论文发展脉络
Chat Paper
正在生成论文摘要