MedAttacker: Exploring Black-Box Adversarial Attacks on Risk Prediction Models in Healthcare
2022 IEEE International Conference on Bioinformatics and Biomedicine (BIBM)(2022)
摘要
Researchers have conduct adversarial attacks against deep neural networks (DNNs) for health risk prediction in the white/gray-box setting to evaluate their robustness. However, since most real-world solutions are trained by private data and released as black-box services on the cloud, we should investigate their robustness in the black-box setting. Unfortunately, existing work ignores to consider the uniqueness of electronic health records (EHRs). To fill this gap, we propose the first black-box adversarial attack method against health risk prediction models named MedAttacker to investigate their vulnerability. It addresses the challenges brought by EHRs via two steps: hierarchical position selection which selects the attacked positions in a reinforcement learning (RL) framework and substitute selection which identifies substitutes with a score-based principle. Particularly, by considering the temporal context inside EHRs, MedAttacker initializes its RL position selection policy by using the contribution score of each visit and the saliency score of each code, which can be well integrated with the deterministic substitute selection process decided by the score changes. We evaluate MedAttacker by attacking three advanced risk prediction models in the black-box setting across multiple real-world datasets, and MedAttacker consistently achieves the highest average success rate and even outperforms a recent white-box EHR adversarial attack technique in certain cases.
更多查看译文
关键词
advanced risk prediction models,black-box adversarial attacks,black-box services,deep neural networks,deterministic substitute selection process,electronic health records,health care,health risk prediction models,hierarchical position selection,MedAttacker,reinforcement learning,RL position selection policy,score-based principle,white-box EHR adversarial attack technique
AI 理解论文
溯源树
样例
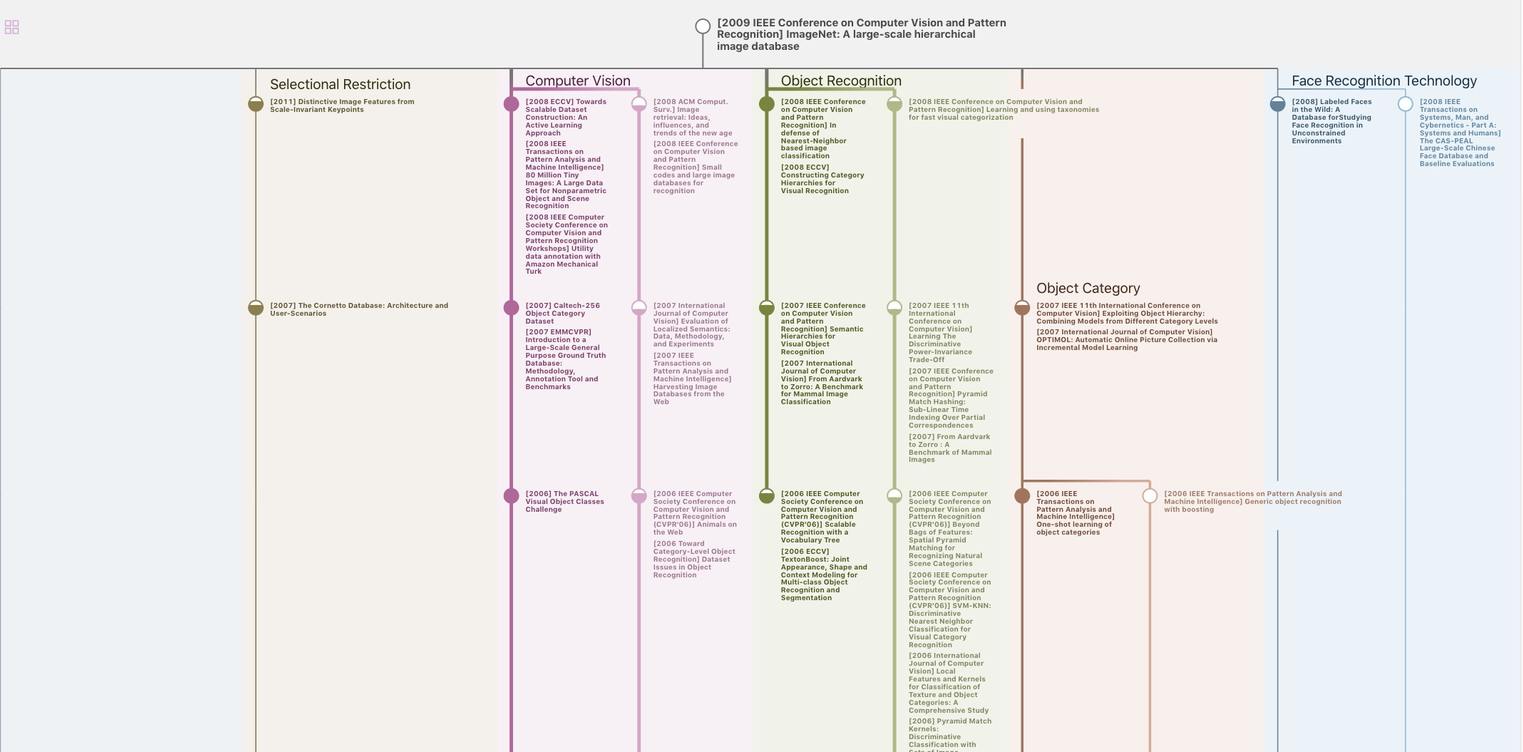
生成溯源树,研究论文发展脉络
Chat Paper
正在生成论文摘要