Investigating Individual- and Group-Level Model Adaptation for Self-Reported Runner Exertion Prediction from Biomechanics
2022 E-Health and Bioengineering Conference (EHB)(2022)
摘要
Despite the many positive effects of sports, it also carries the risk of overuse injuries if done excessively. Early detection of a runner's exertion is therefore highly relevant for preventing and increasing the well-being of individuals. Recent machine learning methods have given little to no attention to the impact of individual characteristics such as age or sex on exertion recognition. We tackle this issue by proposing a personalised machine learning system. To achieve this, we first train a model using a transfer learning approach with shared common layers as well as individual layers per runner. Subsequently, to account for different subgroups, we train separate models for male and female runners. In addition, we improve our results using mutual information estimation for selection of features extracted from biomechanical data. Finally, we explore how personalisation strategies affect the fairness of these models. Our experiments show that the best result is obtained using sex-based group-level adapted models, leading to an MAE of 2.227 for the RPE prediction (scale: [6-20]) compared to the non-adapted baseline with an MAE of 3.009. Furthermore, both individual- and group-level adaptations reduce the variance of the individual results per runner and subgroup, leading to an overall fairer model prediction.
更多查看译文
关键词
Machine Learning,Running,Exertion Prediction,Personalised Model,Model Fairness
AI 理解论文
溯源树
样例
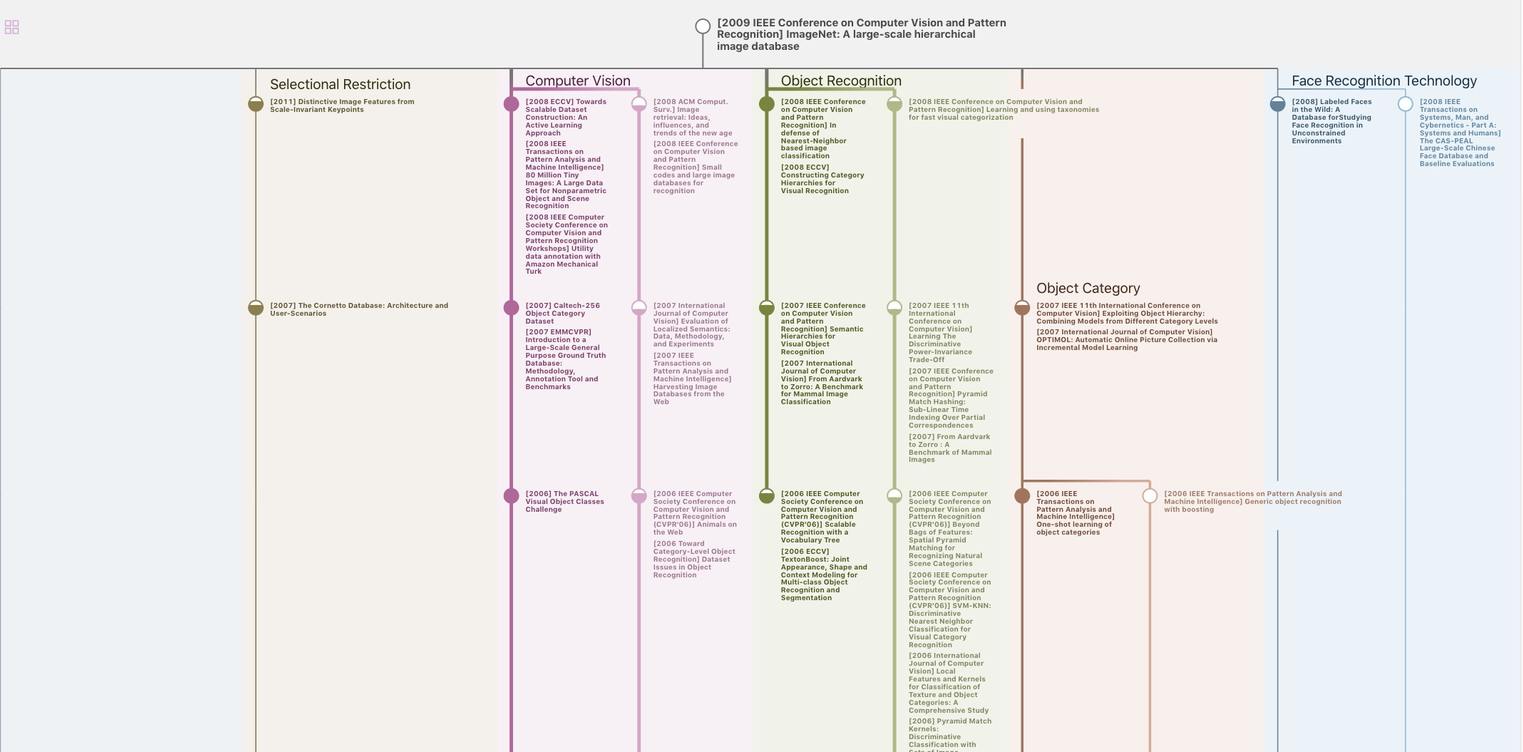
生成溯源树,研究论文发展脉络
Chat Paper
正在生成论文摘要