Resource Allocation in IoT-based Edge Computing using Deep Learning with Optimization Model
2023 Second International Conference on Electronics and Renewable Systems (ICEARS)(2023)
摘要
This study examines the problem of allocating resources for edge computing in IoT networks. In this model, each end device acts independently in deciding whether or not to offload compute chores to itself. As network states, the received signal from end nodes and the point of entry, the computation job column, and the leftover information processing source of the end nodes are considered in order to lessen the long-term summation cost, which encompasses the power requirements and the total completion latency. Cloud computing is the foundation of edge computing, which simply relocates processing, archiving, and connecting nodes closer to the data itself. The IoT architecture is akin to cloud computing in every respect. Limiting latency while making optimal use of energy is a major challenge in edge computing when processing activities provided by IoT devices. Deep learning technology is used to the issue of a chain of choices having to be made at the end devices. Starting with the challenges of resource management in cellular IoT and low-power IoT networks, the usual resource management solutions for these systems justify DL approaches. In future, deep learning research will be recommended for IoT network resource organization.
更多查看译文
关键词
Edge computing,Internet of Things (IoT),resource allocation,deep learning,computational task
AI 理解论文
溯源树
样例
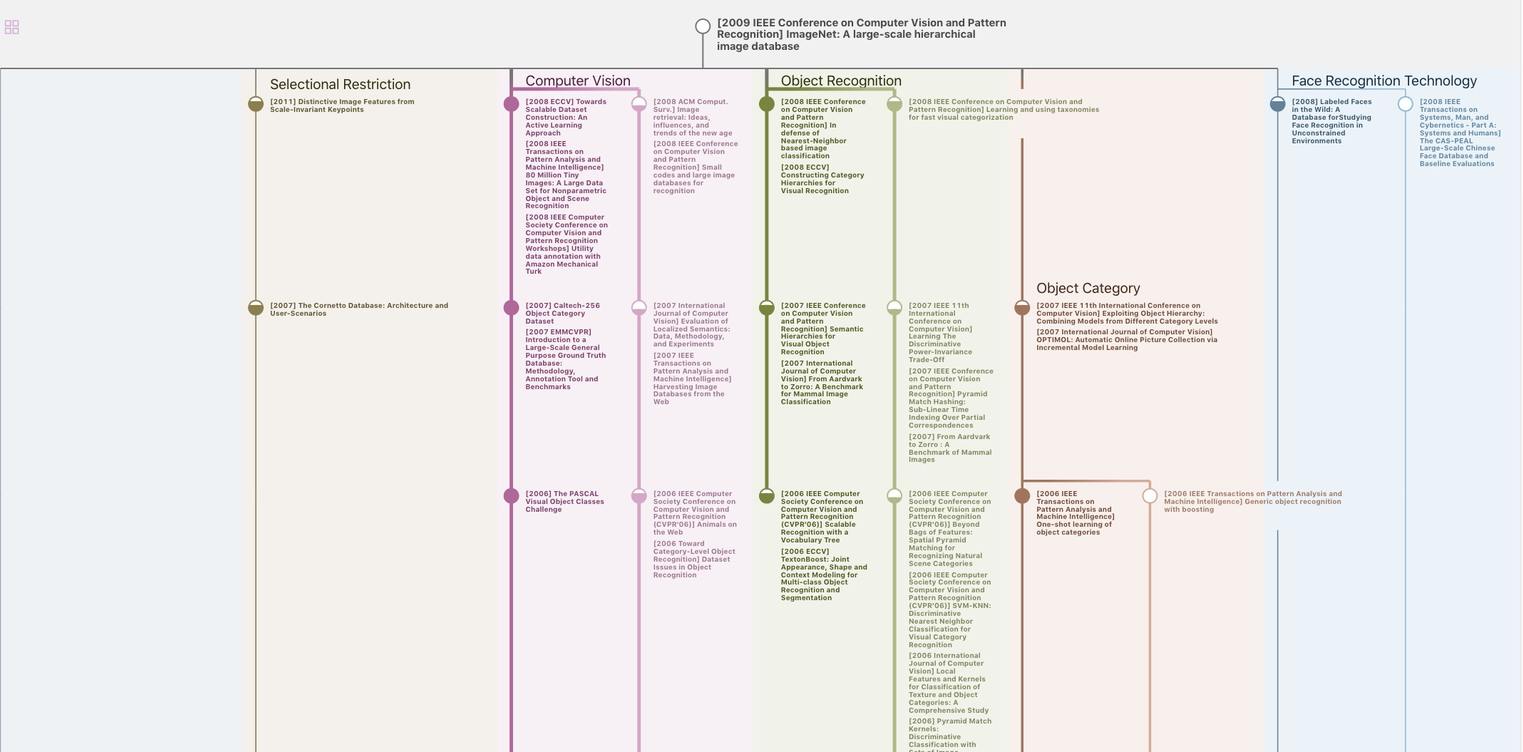
生成溯源树,研究论文发展脉络
Chat Paper
正在生成论文摘要