A Technique for Early Identification of Network Separation to Enhance Frequency Resilience
2023 International Conference for Advancement in Technology (ICONAT)(2023)
摘要
Wind and solar photovoltaic (PV) energy have transformed power systems in the last decade. Integration of renewable energy into grids has resulted in cleaner and greener energy generation and distribution than the conventional fossil-fuel-based grids. However, the variability and asynchrony associated with the renewable-based generation pose security threats such as blackouts following major generation contingency events. Early identification of such serious network separation events is crucial for grid operators to take prompt action and avoid blackouts. To this end, this paper proposes a Long-Short Term Memory (LSTM) based artificial neural network model to forecast network bottlenecks in an early stage. The proposed model is further enhanced by the inclusion of stacked LSTM with more hidden layers than the conventional LSTM. The model is then implemented on a modified Southeast Australian (SA) Power System model with substantial renewable integration. All the dynamic simulations are conducted in the PSSE platform. This platform is also used for time series data mining. The model is then trained and validated on the constructed dataset concerning the real power system. In addition, several performance metrics are evaluated to check the performance of forecasting. The findings show that the model successfully detects and anticipates the tie line overflow during a major synchronous generator loss occurrence every time. Furthermore, all of the performance evaluation metrics validate the proposed model’s goodness of fit. Therefore, the proposed technique utilizing a generalized approach has the potential to be deployed as an effective tool for forecasting network separation events in low-inertia power grids.
更多查看译文
关键词
sustainable energy,LSTM,forecasting,blackout,ANN,frequency resilience,photovoltaic,wind
AI 理解论文
溯源树
样例
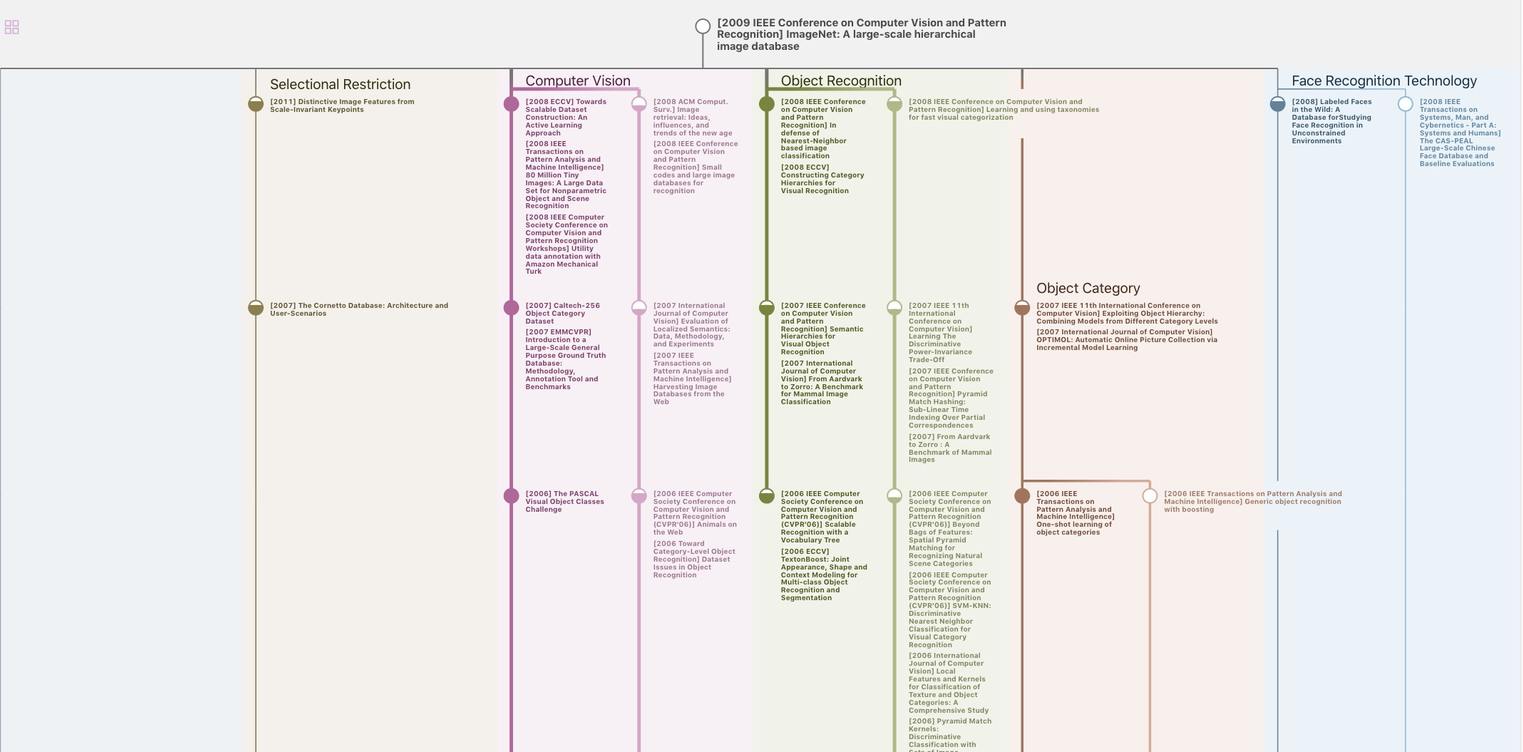
生成溯源树,研究论文发展脉络
Chat Paper
正在生成论文摘要