Discovering the Optimal Setup for Speech Emotion Recognition Model Incorporating Different CNN Architectures
2022 IEEE 14th International Conference on Humanoid, Nanotechnology, Information Technology, Communication and Control, Environment, and Management (HNICEM)(2022)
摘要
Grown in recent decades, emotion recognition from speech data has been a research topic in human-machine communication technologies. Different approaches, structures, algorithms, and models have been designed to represent emotions from speech signals. In practice, however, speech data utilized is acquired from existing speech databases as natural speech database requires a lot of effort and time to acquire. In addition, an optimal setup and architecture must be considered to determine the speech emotion model’s performance. To solve these problems, this paper proposed a speech emotion recognition model with the natural database as an input. The said model will employ Mel-Frequency Cepstral Coefficients (MFCC) to extract the complementary features to be used by the speech emotion recognition model and will utilize the capabilities of different CNN deep learning methods namely; 1D CNN, 2D CNN, and CNN Long Short Term Memory (LSTM) and compare the results in order to determine which method suits the capturing of emotional features of a natural database among five (5) emotion categories: confusion, disappointment, excitement, happiness and neutral. The classification performance is based on extracted features. The experimental evaluation displays the optimal set-up for each deep learning method and its respective performances in terms of the correct emotion recognition rate of the proposed SER model. As a result, the researchers found out that the optimal architecture in working with Natural Speech Database is the 2D CNN and the optimal epochs depends on the classification subject.
更多查看译文
关键词
Emotion Recognition,Deep Learning,1D CNN,2D CNN,CNN-LSTM,Natural Database,MFCC
AI 理解论文
溯源树
样例
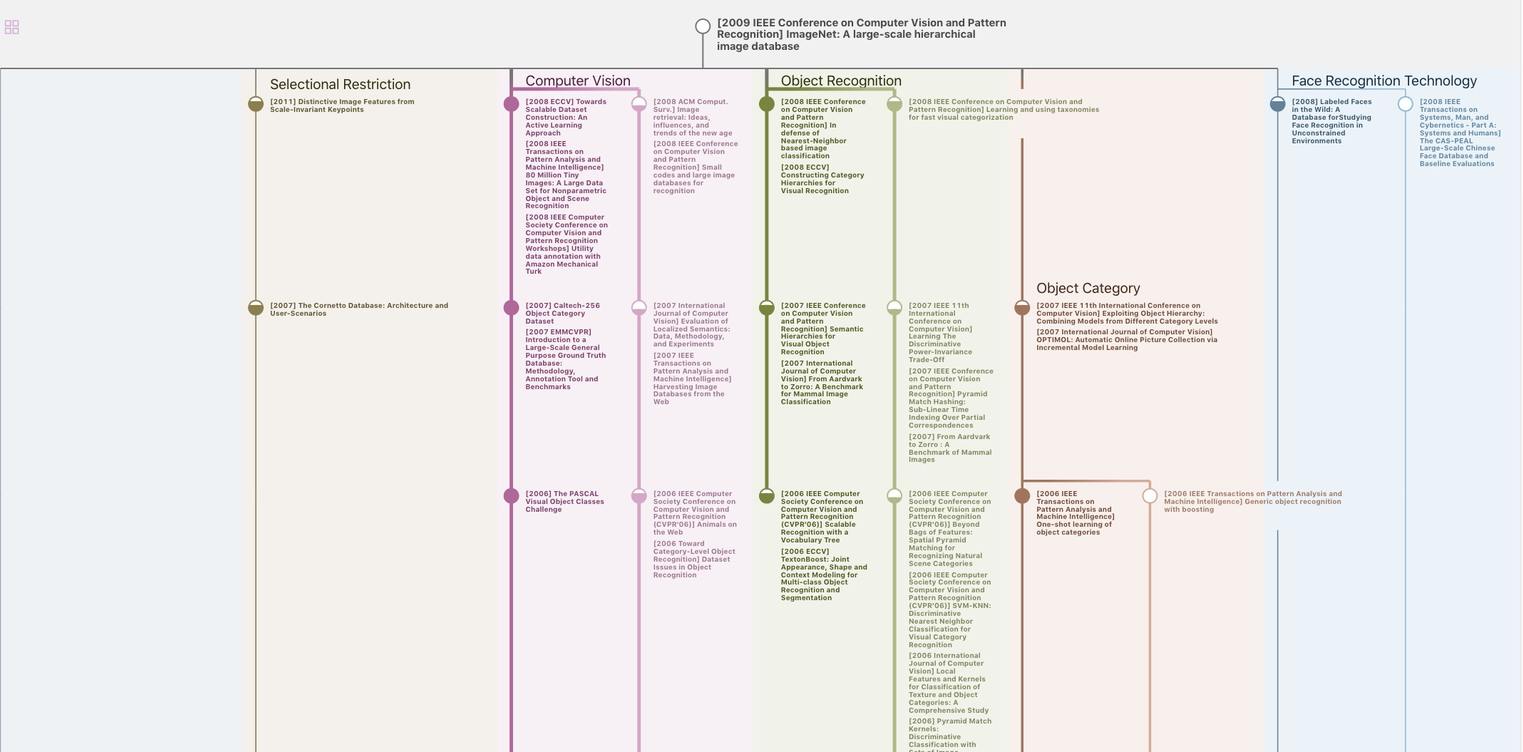
生成溯源树,研究论文发展脉络
Chat Paper
正在生成论文摘要