Can Pretrained Language Models Reason on Sparse Commonsense Knowledge Graph?
2022 IEEE 8th International Conference on Computer and Communications (ICCC)(2022)
摘要
Commonsense knowledge is the knowledge shared by most humans, which is always stored in commonsense knowledge graph (CKG) as triplets. In this paper, we focus on the task of CKG competition, whose target is to predict the tail (head) target given the head (tail) entity and the relation. Most existing works employ the graph-based models, which aggregate information from neighboring entities on CKG. Despite their effectiveness, they still suffer from two main weaknesses. Firstly, the semantic relations between head and tail entities are neglected. Secondly, due to the sparsity of CKG, they rely on the graph densification that it will bring unexpected noises. To solve these problems, we propose a unified framework for COmmonSense knowledge graph completion based on BERT, namely COS-BERT. Firstly, we transfer each triplet into a natural sentence. Then, we fine-tune the pretrained language model using the transformed sentences. Finally, we rank the candidates based on the output representation of sentences. Furthermore, we add a pre-filter to obtain a subset of candidates on the inference stage to save unnecessary computation costs. Comprehensive experiments have demonstrated the superiority of COS-BERT over the state-of-the-arts.
更多查看译文
关键词
commonsense reasoning,pretrained language model
AI 理解论文
溯源树
样例
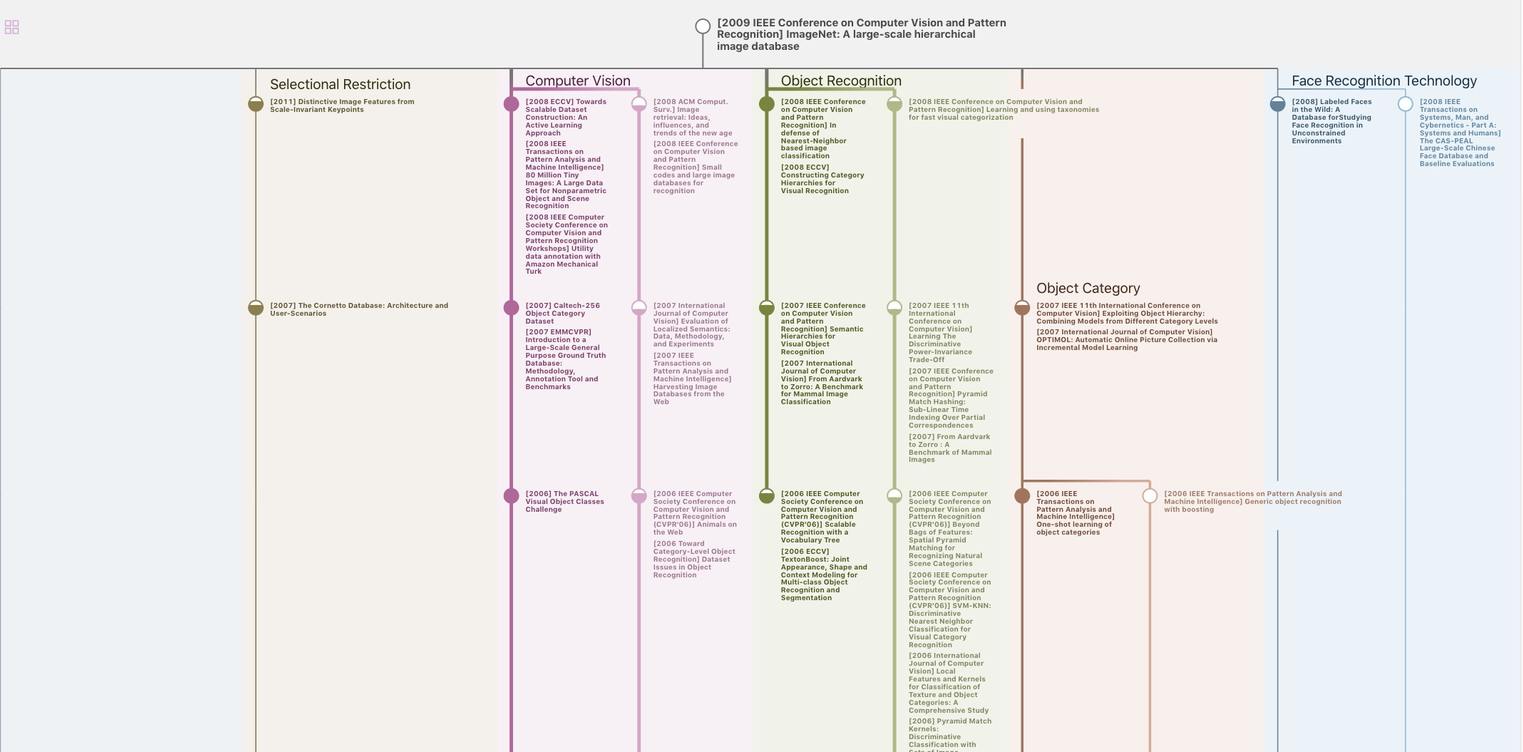
生成溯源树,研究论文发展脉络
Chat Paper
正在生成论文摘要