An In2 O3 Nanotubes based Gas Sensor Array combined with Machine Learning Algorithms for Trimethylamine Detection
2021 IEEE 16th International Conference on Nano/Micro Engineered and Molecular Systems (NEMS)(2021)
摘要
This work focuses on developing an electronic nose system with machine learning algorithm for detection of trimethylamine (TMA). Pure and Ga-doped In
2
O
3
nanotubes are synthesized by a simple electrospinning method, and four kinds of gas sensors (pristine, 1% Ga, 10% Ga, and 20% Ga-doped In
2
O
3
) are fabricated to form a sensor array. Results show that the sensor array can classify TMA effectively from interference gases (xylene, ethanol, hydrogen sulfide) by a support vector machine (SVM) algorithm. Several algorithms, including radial basis function neural network (RBFNN), back propagation neural network (BPNN) and principal component analysis combined with linear regression (PCA-LR), are used to predict the concentration level of each gas. For TMA gas, the trained algorithms can predict its concentration with average relative errors of 1.22% for RBFNN, 2.5% for BPNN and 13.34% for PCA-LR. Furthermore, the binary mixtures of TMA and ethanol are measured and used to train the above algorithms, and the lowest average relative error of 1.74% is achieved in the case of RBFNN algorithm.
更多查看译文
关键词
trimethylamine detection,electronic nose system,machine learning algorithm,electrospinning method,hydrogen sulfide,support vector machine algorithm,radial basis function neural network,principal component analysis,PCA-LR,trained algorithms,RBFNN algorithm,TMA gas sensor array,linear regression analysis,backpropagation neural network,SVM algorithm,xylene,ethanol,In2O3:Ga
AI 理解论文
溯源树
样例
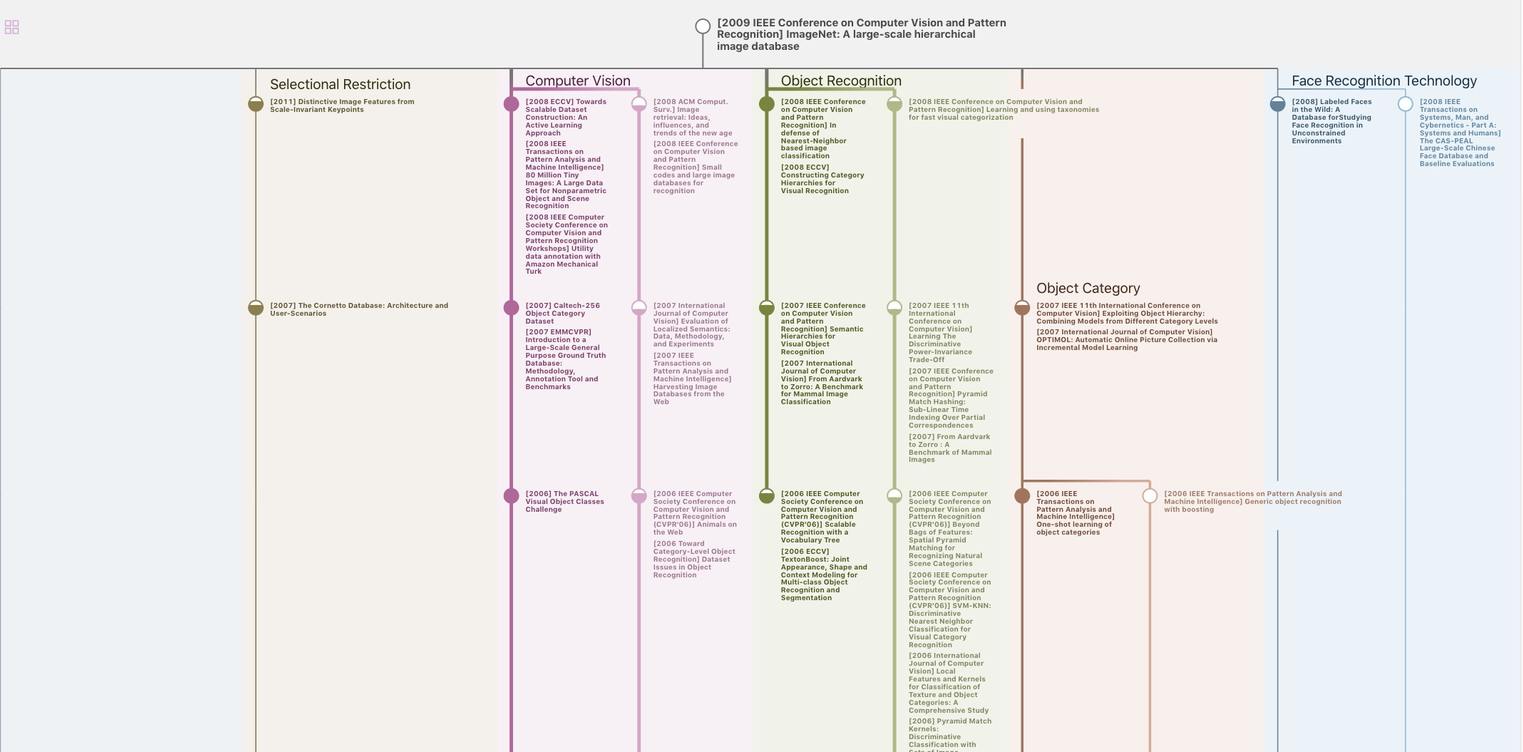
生成溯源树,研究论文发展脉络
Chat Paper
正在生成论文摘要