Evaluation of Different Machine Learning Framework in Daily 1 Km Resolution Gridded Pm2.5 Prediction
IGARSS 2023 - 2023 IEEE International Geoscience and Remote Sensing Symposium(2023)
摘要
Atmospheric fine particles (PM
2.5
) poses a significant threat to human health and several studies have been conducted to predict the PM
2.5
concentrations in time series at monitoring stations. Gridded PM
2.5
concentration prediction is relatively scarce. In this study, we evaluated representative ML-Based framework in daily 1 km gridded PM
2.5
prediction to identify suitable prediction methods. To achieve this, random forest, convolutional neural network, and transformer were selected for evaluation. The results showed that the transformers model slightly outperformed the other two models in both qualitative and quantitative evaluations. However, all three models showed poor performance during winter, as the PM
2.5
concentration is inherently higher and more variable. It is precisely such situation that people are most concerned about. Our research suggests that resolving "class imbalance" and "concept drift" are two potential approaches to address this issue.
更多查看译文
关键词
PM2.5,Air pollution,Machine learning,Spatiotemporal prediction
AI 理解论文
溯源树
样例
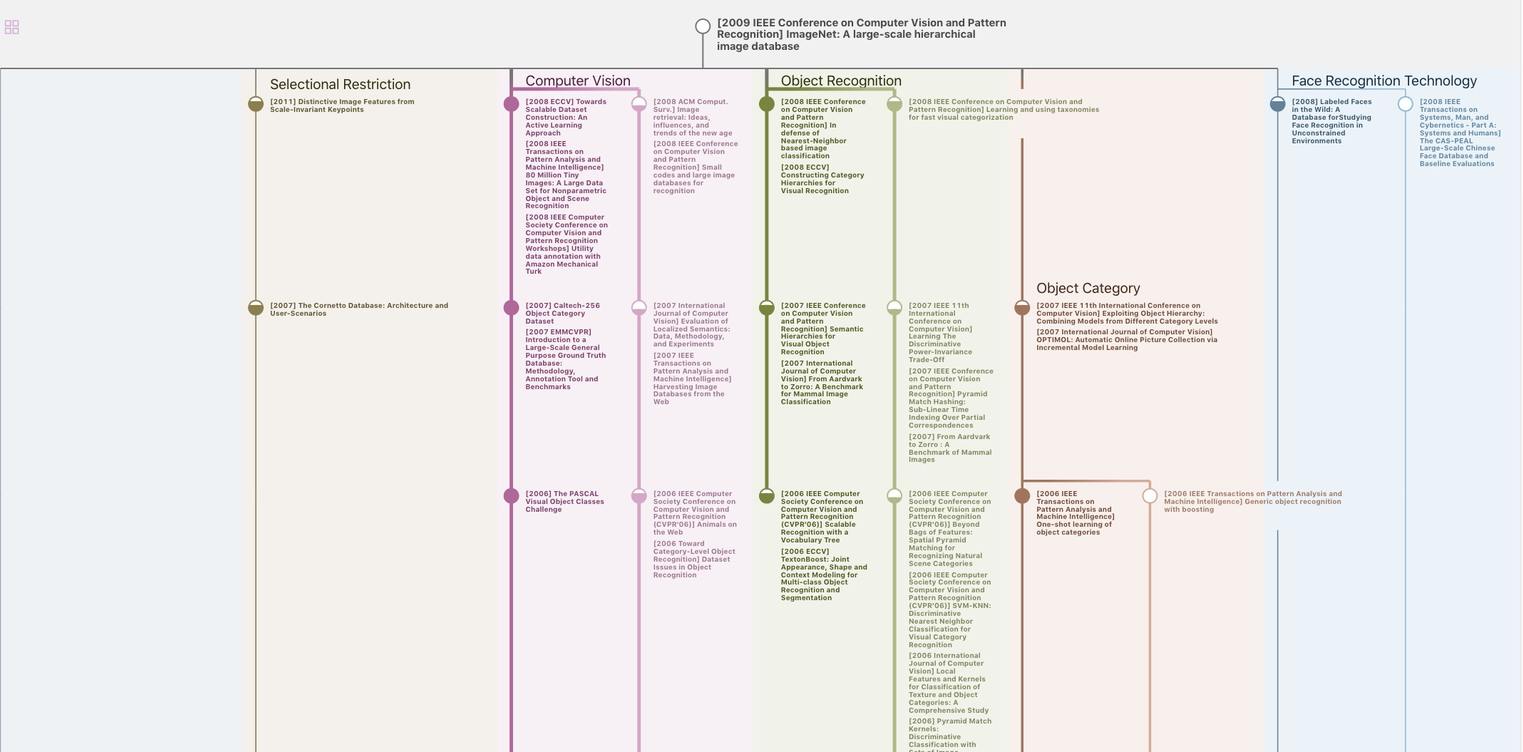
生成溯源树,研究论文发展脉络
Chat Paper
正在生成论文摘要