Anomaly detection using Federated Learning: A Performance Based Parameter Aggregation Approach
2023 3rd International Conference on Intelligent Technologies (CONIT)(2023)
摘要
Anomaly detection is a significant challenge that exists in multiple industries, including healthcare, finance, and manufacturing. Machine Learning techniques are extensively being used to solve these problems. However, data privacy concerns and regulations are making acquiring the necessary data for model training increasingly challenging, particularly in sensitive sectors. Federated learning has emerged as a promising solution, allowing local models to be trained on edge devices using local datasets and model parameters to be shared with a central server for aggregation. This allows the models to reap the benefits of the distributed data without actually sharing any data. However, the current aggregation method used in federated learning is susceptible to models trained on bad data, which can impact the accuracy and reliability of the resulting model. To address this issue, the study proposes a new filtered aggregation algorithm that greatly mitigates the effect of models trained on bad data. The proposed algorithm accounts for models that cannot detect anomalous patterns and gives them much less representation in the final model, resulting in improved accuracy and reliability.
更多查看译文
关键词
Federated learning,Autoencoder,Edge Computing,Anomaly detection
AI 理解论文
溯源树
样例
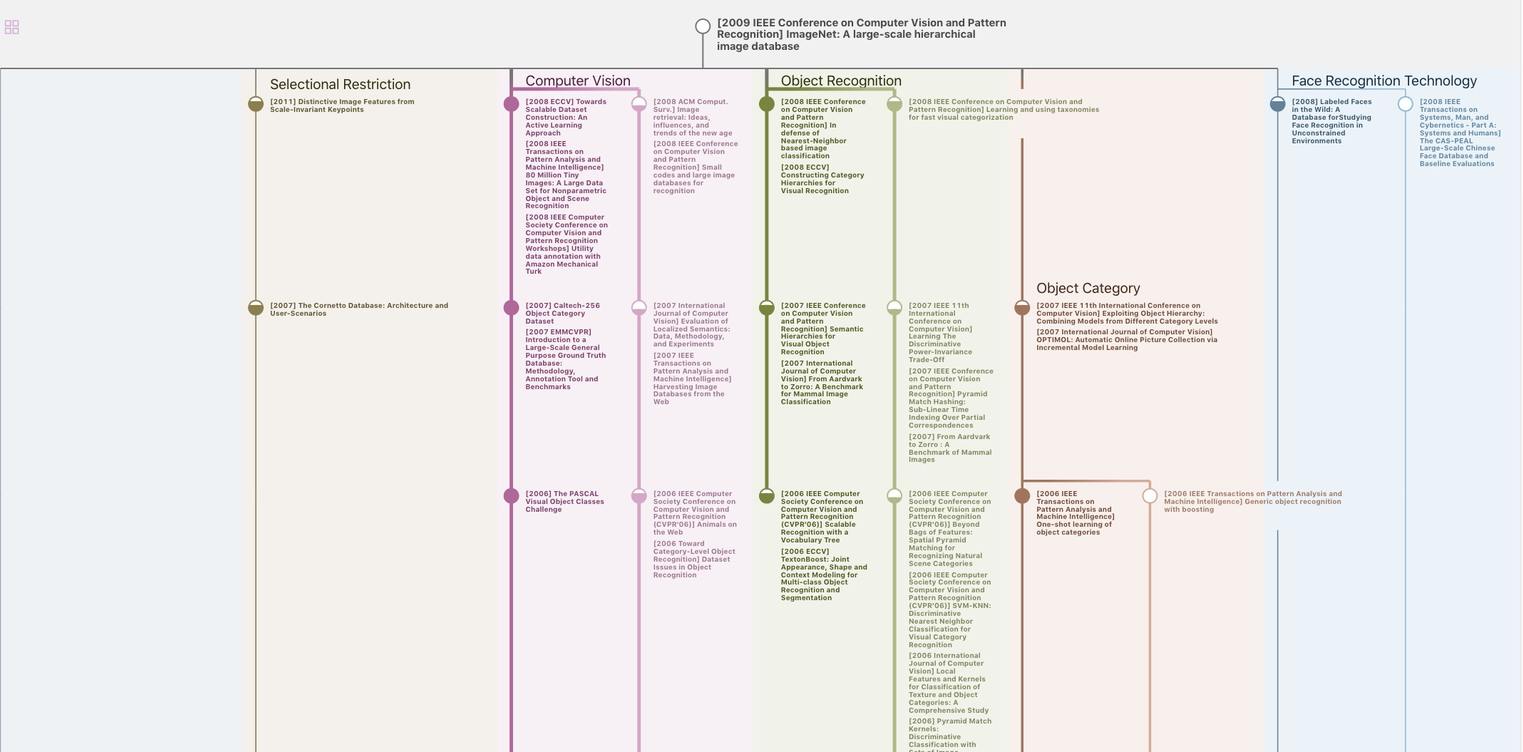
生成溯源树,研究论文发展脉络
Chat Paper
正在生成论文摘要