Deep Learning-Based Real-time Anomaly Detection for Power Electronics-Dominated Grid
2023 IEEE Power and Energy Conference at Illinois (PECI)(2023)
摘要
This paper presents a type of recurrent neural network (RNN), namely, long short-term memory (LSTM) for real-time anomaly detection and classification at power electronics dominated grids (PEDG) inverters network. The proposed approach classifies signal sequences representative of voltage, frequency and rate of change of frequency (ROCOF) sensed at inverters point of common coupling (PCC), to normal or anomalous types. This paper addresses the challenges of training the network for real-time detection of anomalies in power grid due to large context of events. Numerous disturbances were included in the simulated PEDG for creating an effective database to avoid the neural network algorithm overfitting. The neural network classification accuracy and loss proved its capability of effectively detecting anomalous grid clusters with abnormal voltage and frequency behavior resulting from compromised inverters and their sensors. The preliminary case studies validate the performance and functionality of proposed real-time anomaly detection method for PEDG.
更多查看译文
关键词
Anomaly detection,anomaly classification,power electronics dominated grid,cybersecurity,LSTM neural networks
AI 理解论文
溯源树
样例
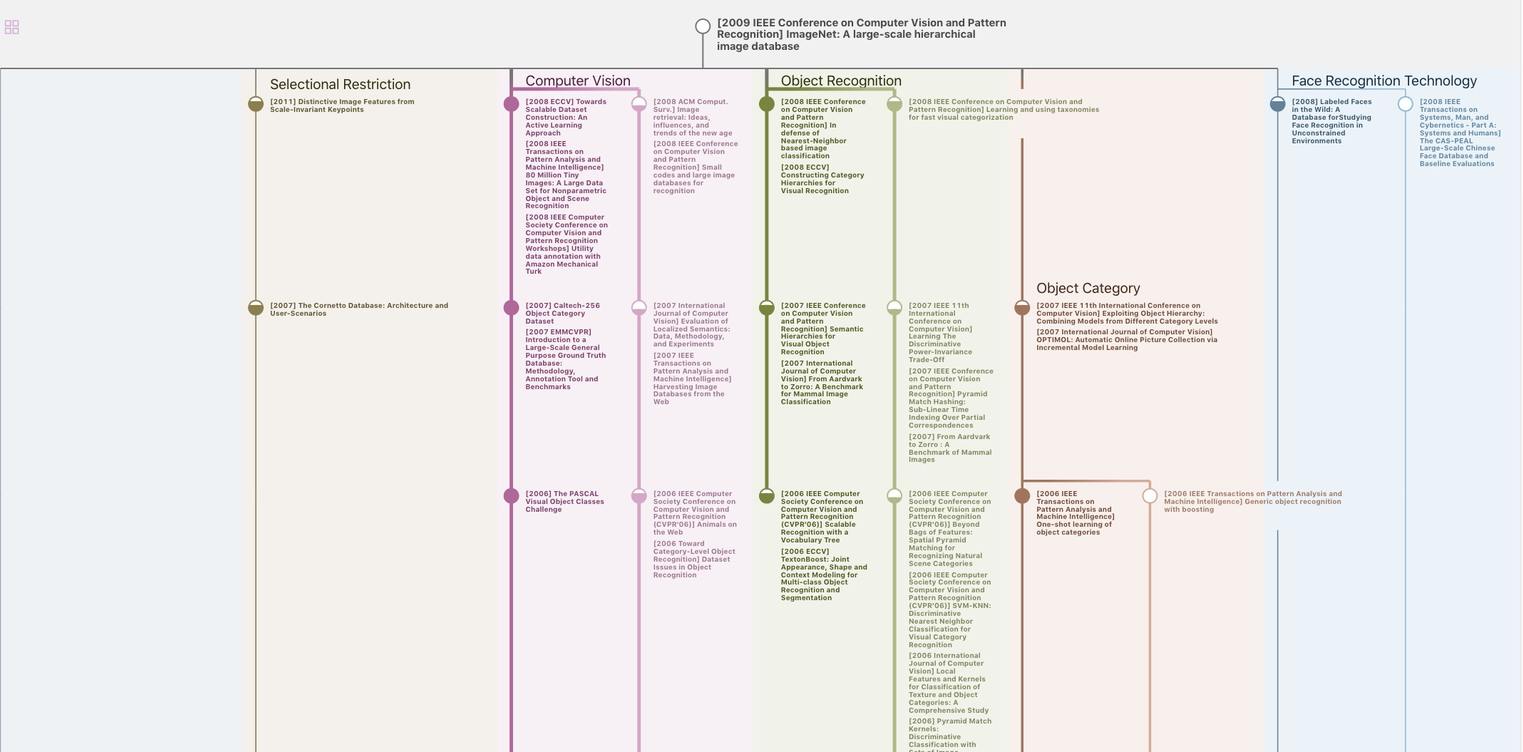
生成溯源树,研究论文发展脉络
Chat Paper
正在生成论文摘要