Adaptive Lazily Aggregation Based on Error Accumulation
2023 4th International Conference on Electronic Communication and Artificial Intelligence (ICECAI)(2023)
摘要
Federated Learning (FL) enables multiple clients to collaboratively train models without exposing their local data. FL is an effective approach to utilizing localized data while preserving clients’ data privacy, but it also brings significant communication overhead. To reduce communication overhead of FL, this paper proposes the Adaptive Lazily Aggregation based on Error Accumulation (EA-ALA) algorithm. It uses adaptive constraints to determine whether a client can skip a communication round with the server so as to diminish communication cost. It also adopts error accumulation to improve model accuracy. The experimental results on CIFAR10 and Fashion-MNIST datasets show that compared to vanilla FL, EA-ALA consumes only 52% and 61% of communication rounds to achieve higher model accuracy.
更多查看译文
关键词
Federated Learning,Adaptive,Lazily Aggregation,Error Accumulation
AI 理解论文
溯源树
样例
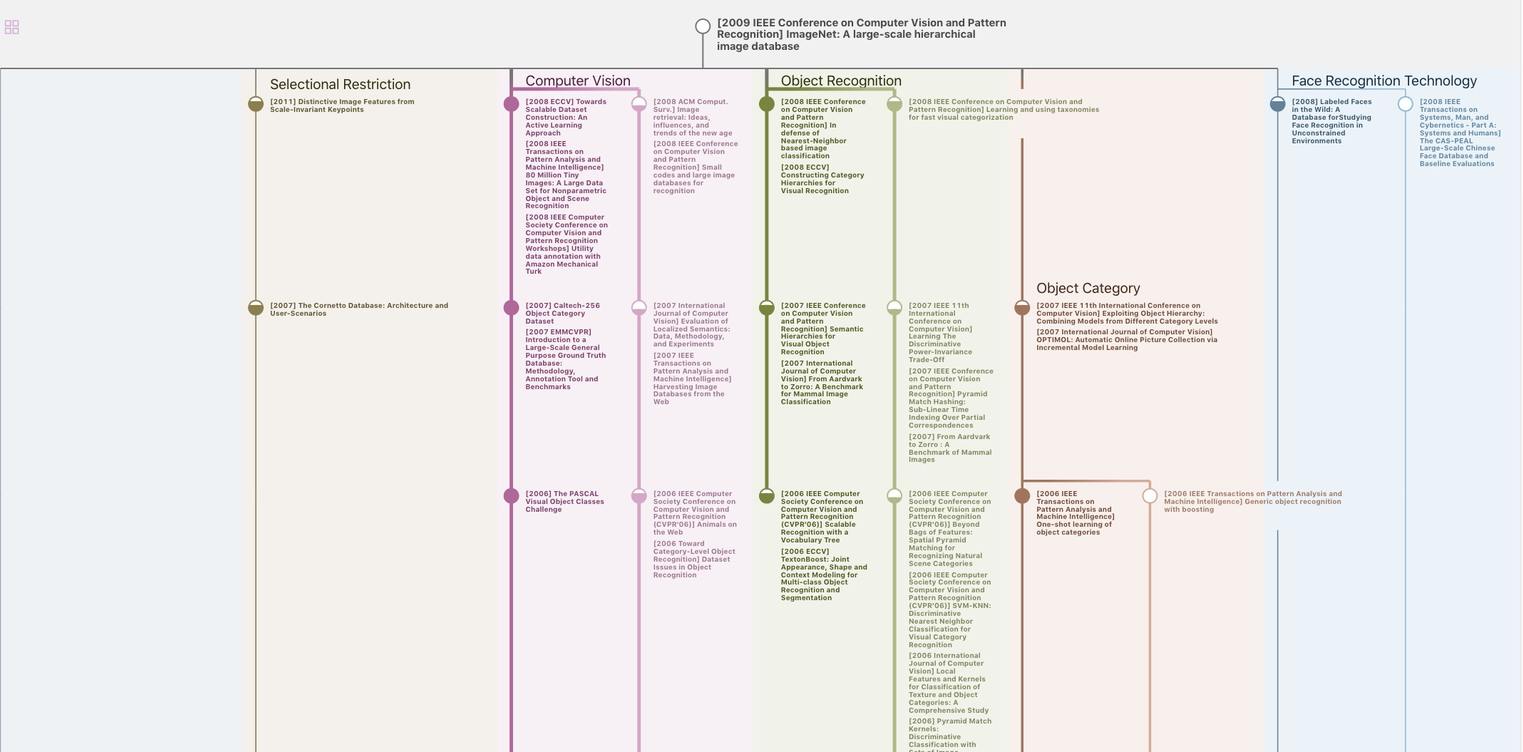
生成溯源树,研究论文发展脉络
Chat Paper
正在生成论文摘要