Affective Commonsense Knowledge Enhanced Dependency Graph for aspect sentiment triplet extraction
JOURNAL OF SUPERCOMPUTING(2023)
摘要
Most existing aspect sentiment triplet extraction models emphasize the adoption of novel tagging scheme to jointly extract three elements of sentiment triplets, but they overlook the intrinsic information of individual words, including the implicit relationships between words, which results in the inaccurate triplet extraction. In this paper, we propose a novel method named Affective Commonsense Knowledge Enhanced Dependency Graph, which considers the sentiment information contained in each word and the relative positional distance relationships between them. Specifically, a dependency graph is generated, incorporating the sentiment information obtained through an external knowledge base called SenticNet. This dependency graph is integrated into a multi-layer graph convolutional network to enhance the sentiment dependency relationships between words. Additionally, an attention mechanism with relative position embeddings is utilized to acquire word representations which incorporate contextual and syntactic information. Furthermore, an Expanded Grid Tagging Scheme is employed to provide more accurate description of the relationships between words. In terms of the F1 metric, on the four SemEval datasets, 14res, 14lap, 15res, and 16res, our model improvements were 1.64, 1.66, 2.01, and 2.56 when compared to the baselines, respectively. On the four datasets of ASTE-Data-V2, our model improvements were 0.41, 1.03, 1.12, and 0.34, respectively. Experimental results demonstrate the superior performance of our method.
更多查看译文
关键词
Sentiment analysis,Aspect sentiment triplet extraction,Affective commonsense knowledge
AI 理解论文
溯源树
样例
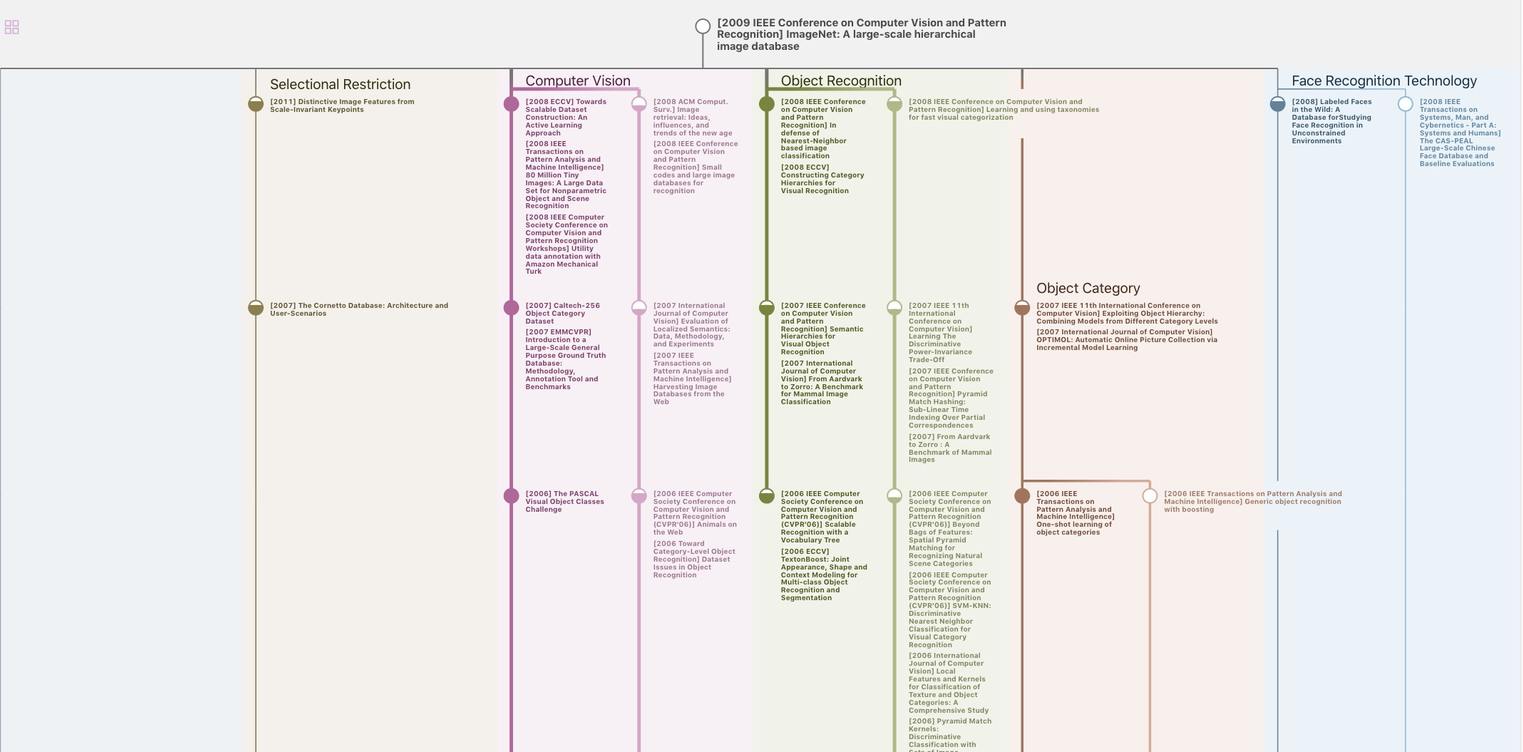
生成溯源树,研究论文发展脉络
Chat Paper
正在生成论文摘要