CoAM-Net: coordinate asymmetric multi-scale fusion strategy for polyp segmentation
Applied Intelligence(2023)
摘要
Automatic segmentation of colorectal polyps can help physicians effectively identify the exact location and size of polyps. Current methods are rapidly developing and demonstrating achievements in colonoscopic image segmentation. However, the effectiveness is limited by two aspects. 1) A changeable intestinal environment causes image variability. 2) The convolutional neural network has limitations in extracting long-distance relationships. We propose a coordinate asymmetric multi-scale fusion network (CoAM-Net) to manage the above problems. Transformer and CNN are combined to improve automatic polyp segmentation accuracy. The proposed method employs a coordinate asymmetric module (CA-Module), a multi-scale fusion decoder (MSD), and a multi-scale spatial attention mechanism (MSA) to utilize global and local features. CA-Module is a component of the CNN feature extractor that makes intensive use of contextual information. By extracting multi-scale features and attention mechanisms, MSA effectively enriches CNN spatial features and highlights the importance of relevant features. The designed MSD effectively exploits long-distance relationships and local features to build a more accurate decoding structure. We compare our method with twelve other state-of-the-art works on the Kvasir-SEG and CVC-ClinicDB datasets. Experiments show that our method performs optimally in most metrics. We also performed generalization experiments on the ETIS-Larib Polyp DB, CVC-T, and CVC-ColonDB datasets to validate that CoAM-Net can achieve good results. The model is available at https://github.com/wangyuanyuan0/CoAM-Net .
更多查看译文
关键词
Multi-scale,Feature fusion,Polyp segmentation,Long-distance relationship
AI 理解论文
溯源树
样例
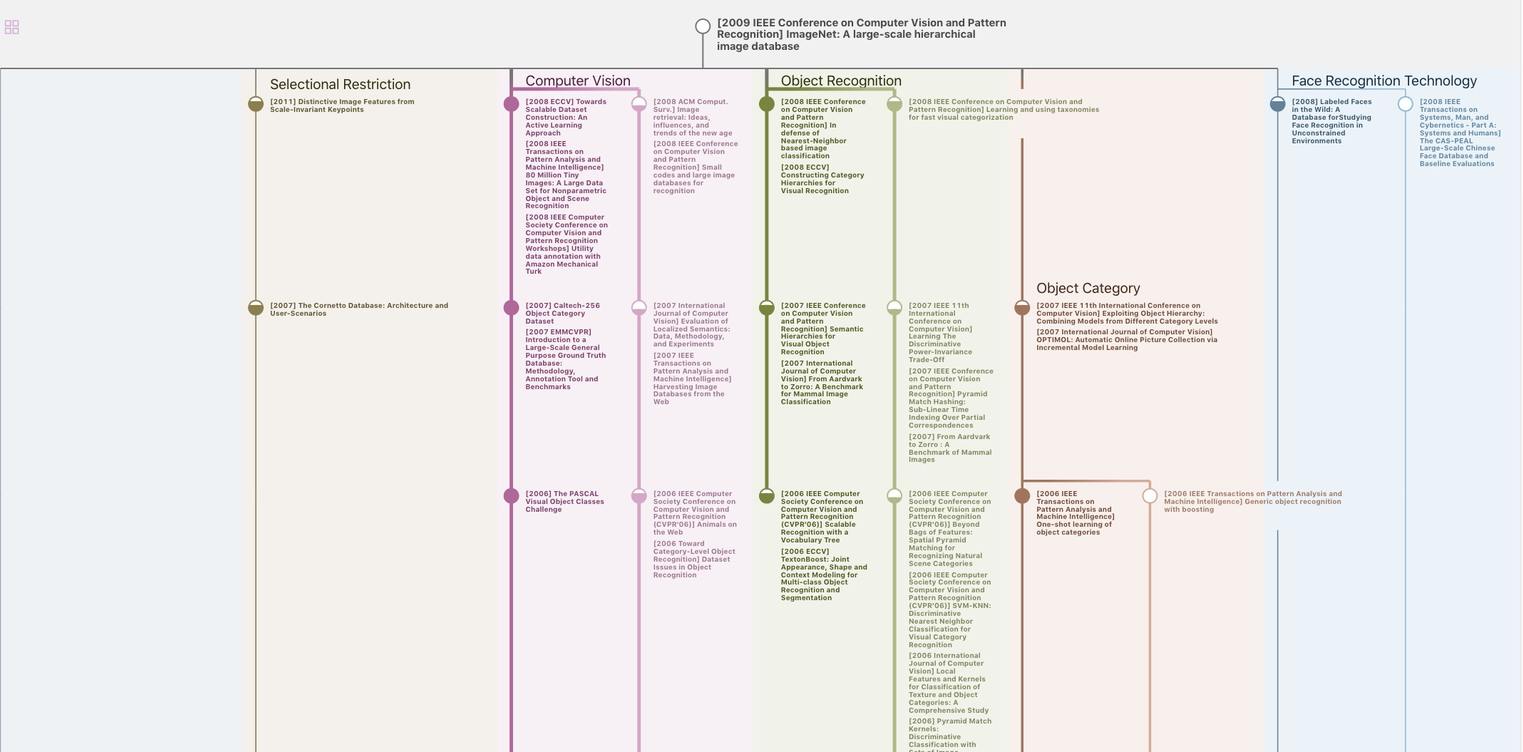
生成溯源树,研究论文发展脉络
Chat Paper
正在生成论文摘要