A mutation-based modified binary fruit fly optimization for feature selection to predict CD4+/CD8+ T-cells epitopes
Soft Computing(2023)
摘要
The healthcare sector is advancing with emerging technologies that help to detect new diseases or viruses worldwide. Generally, virus detection is based on various symptomatic tests and analysis of data samples that contain raw data (blood sample, protein sequence, etc.). Raw data cannot be directly processed by any machine learning (ML) technique. Therefore, feature extraction (FE) techniques are required to generate feature vectors. FE methods yield high dimensional data. Also, ML alone cannot provide accurate results due to large feature vectors. Thus, the feature selection (FS) technique is apt to solve this problem. The paper proposes a feature engineering module in which the FE module is developed and a new FS technique is proposed. The feature engineering module can help in the vaccine development process as accurate disease diagnosis is critical as any vaccine's success depends on precise disease detection. For the first time, this paper uses a metaheuristic method for FS in T-cell prediction. A new variant of the original fruit fly optimization algorithm (FFOA) is proposed in which the problem of local optima and slow convergence of FFOA is solved. The proposed variant of FFOA is named modified binary fruit fly optimization algorithm (MBFFOA) in which the mutation operator is updated. MBFFOA is first tested on benchmark functions and then tested on dengue and Zika virus datasets to predict CD4+ and CD8+ T-cells epitopes. The performance of MBFFOA is compared with that of competing metaheuristic algorithms. The performance of MBFFOA is statistically analyzed by Bonferroni–Dunn post-hoc test.
更多查看译文
关键词
CD4+ T-cell,CD8+ T-cell,Fruit fly optimization,Feature selection,Vaccine,Mutation,DENV,ZIKAV
AI 理解论文
溯源树
样例
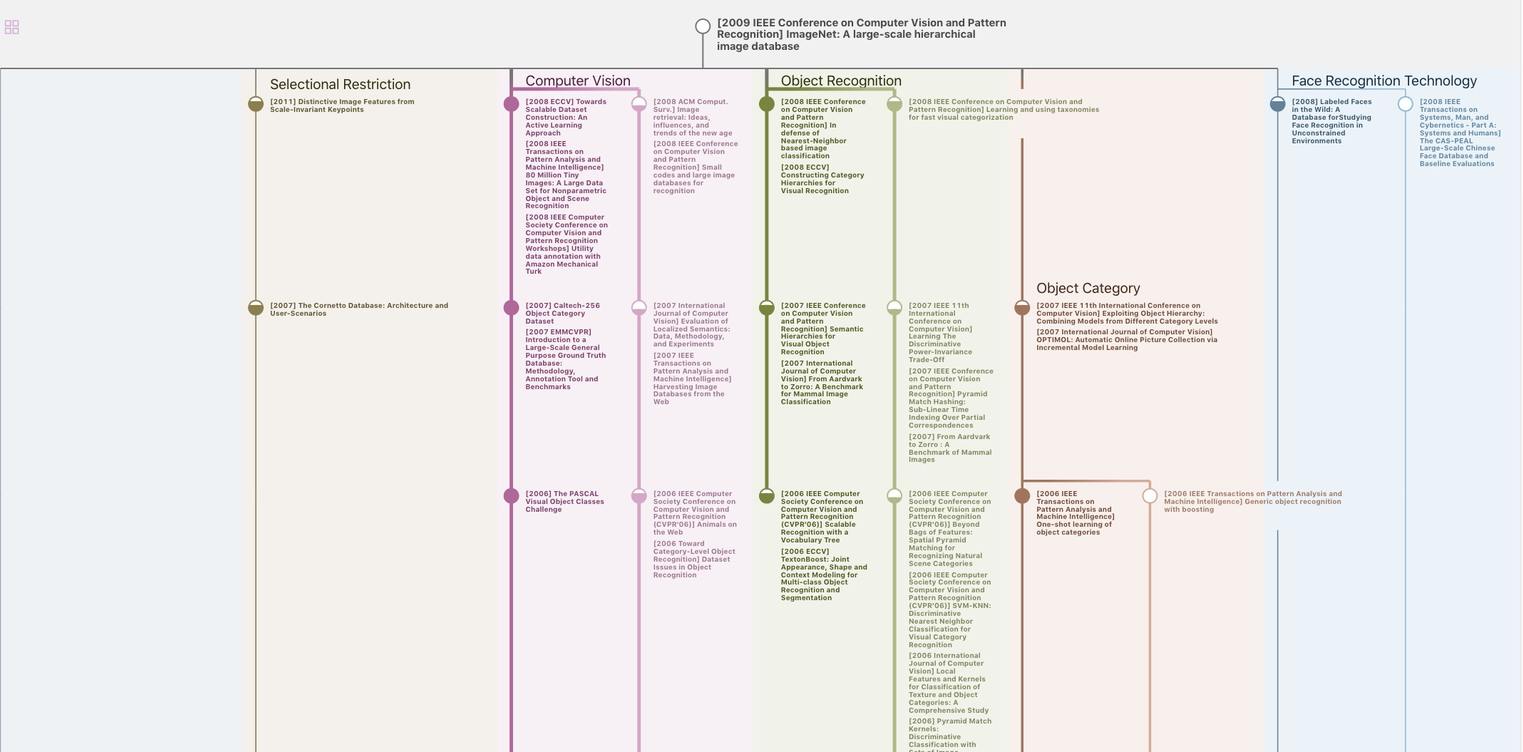
生成溯源树,研究论文发展脉络
Chat Paper
正在生成论文摘要