Vehicle Trajectory Prediction With Interaction Regions and Spatial-Temporal Attention
IEEE ACCESS(2023)
摘要
Vehicle trajectory prediction is a key technology in autonomous driving, one of the aims of which is to ensure the safety of vehicle travelling and lane changing. Especially in complex traffic scenarios, it is critical to accurately predict the vehicle trajectory so that the automated driving system can make appropriate response actions to help the vehicle driver avoid accidents to the greatest extent possible. Some studies on vehicle trajectory prediction have used recurrent networks (LSTMs) to extract temporal correlations and additional convolutional neural networks (CNNs) to capture spatial correlations. This hybrid model can be added more operations, so we propose a new CNN-LSTM hybrid model containing multi-module, interaction regions and spatial-temporal attention mechanisms (IA-CSTM). In this paper, the traffic scene is divided into multiple interaction regions, and vehicles in different regions have different impacts on the predicted vehicles. The model has multiple modules that deal with vehicle information in different interaction regions. The model will cross-fuse vehicle information in important interaction regions to enhance the model's perception of vehicle information. Multiple modules are linked through a spatial-temporal attention mechanism and capture the relevance of the vehicle in the interaction region to predicted vehicle.
更多查看译文
关键词
Trajectory,Correlation,Tensors,Predictive models,Data models,Vehicle dynamics,Space vehicles,Spatiotemporal phenomena,Vehicle trajectory prediction,interaction regions,multi-module,spatial-temporal attention mechanism,IA-CSTM
AI 理解论文
溯源树
样例
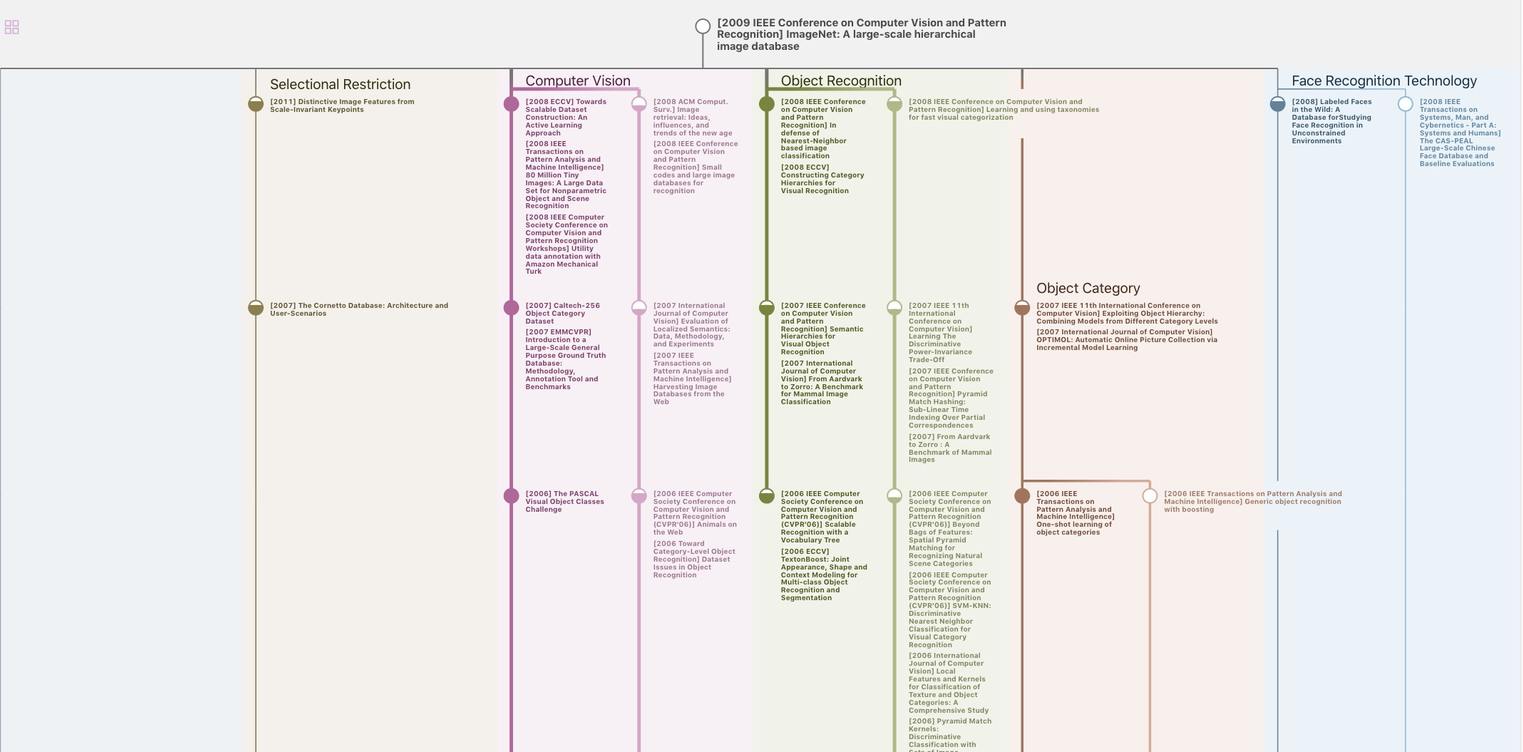
生成溯源树,研究论文发展脉络
Chat Paper
正在生成论文摘要