Self-Supervised Accent Learning for Under-Resourced Accents Using Native Language Data
ICASSP 2023 - 2023 IEEE International Conference on Acoustics, Speech and Signal Processing (ICASSP)(2023)
摘要
In this paper, we propose a novel method to improve the accuracy of an English speech recognizer for a target accent using the corresponding native language data. Collecting labeled data for all accents of English to train an end-to-end neural speech recognizer for English is a difficult and expensive task. Also, finding a pool of representative English speakers for any arbitrary accent to collect unlabeled data can be a difficult task. However, collecting unlabeled speech data for any native language is a much simpler task. It is important to note that the accents of most non-native English speakers are heavily biased by the co-articulation of sounds in their own native language. In view of this, we propose to use unlabeled native language data to learn self-supervised representations during the pre-training stage. The pre-trained model is then fine-tuned using limited labeled English data for the target accent. Experiments using native language data to pre-train an English recognizer followed by fine-tuning using target accented English show significant improvements in word error rates on four different accents (Great Britain, Korean, Chinese, Spanish).
更多查看译文
关键词
E2E ASR,Accent Adaptation,Self-supervised Training,Low Resource
AI 理解论文
溯源树
样例
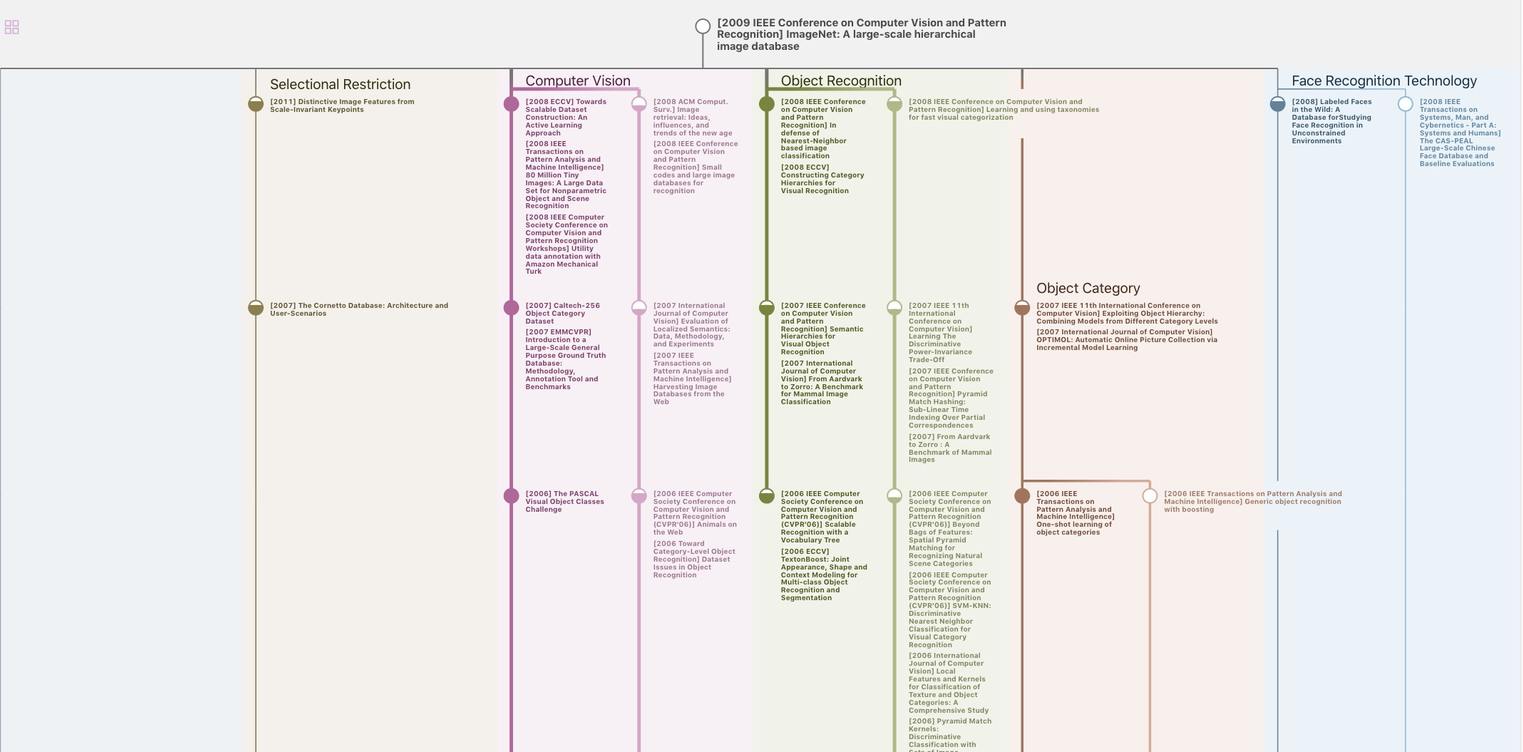
生成溯源树,研究论文发展脉络
Chat Paper
正在生成论文摘要