Clustering-Based Supervised Contrastive Learning for Identifying Risk Items on Heterogeneous Graph
ICASSP 2023 - 2023 IEEE International Conference on Acoustics, Speech and Signal Processing (ICASSP)(2023)
摘要
Risk item identification is vital for protecting the health of ecommerce trades. Existing solutions prefer to model structure information besides item attributes and optimize parameters in cross-entropy (CE) manners. However, the few labeled and imbalanced supervision in real-world scenarios usually results in poor generalization of CE optimization. More seriously, the pattern-level difference of risk items is often neglected in binary supervised learning, leading to limited performance. In this paper, we propose a novel Clustering-based Supervised Contrastive Learning (CSCL) to address the two challenges. CSCL first devises a contrastive heterogeneous graph neural network that fully exploits multiple risk relations in contrastive learning, keeping generalization performance. It then designs a clustering-based reweighted sampling strategy to search informative positive and negative training instances for effective pattern-level optimization. We test the performance on Xianyu Platform, and experimental results demonstrate that CSCL outperforms all baselines.
更多查看译文
关键词
Risk item identification,multiple subcategory problem,contrastive learning,graph neural network
AI 理解论文
溯源树
样例
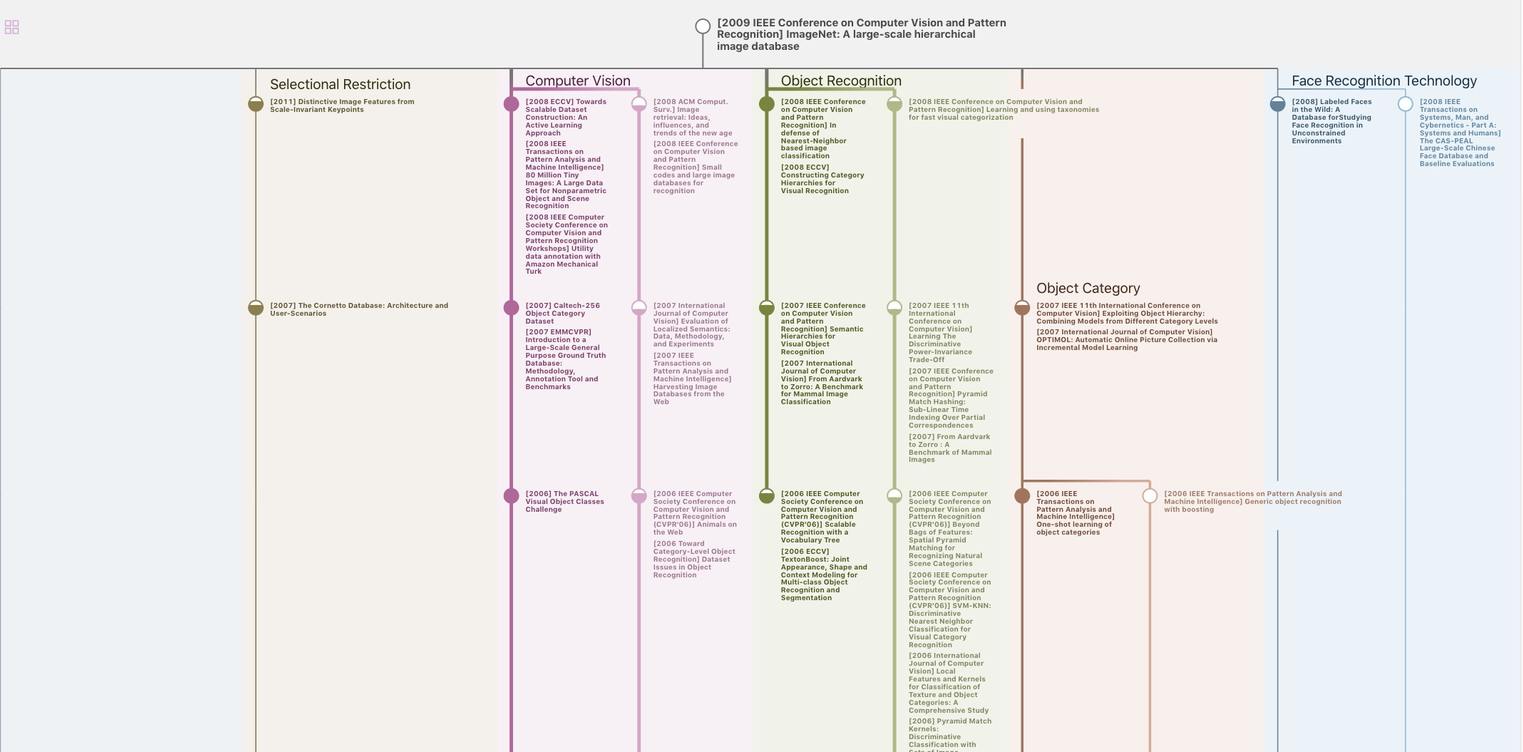
生成溯源树,研究论文发展脉络
Chat Paper
正在生成论文摘要