Learning To Regularized Resource Allocation with Budget Constraints
ICASSP 2023 - 2023 IEEE International Conference on Acoustics, Speech and Signal Processing (ICASSP)(2023)
摘要
Online resource allocation problem with budget constraints has a wide range of applications in network science and operation research. In this problem, the decision maker needs to make actions that consume resources to accumulate rewards. Contrary to prior work, we introduce a non-linear and non-separable regularizer to this problem that acts on the total resource consumption. The motivation for introducing the regularizer is allowing the decision maker to tradeoff the reward maximization and metrics optimization such as load-balancing and fairness that appears frequently in practical needs. Our goal is to simultaneously maximize additively separable rewards and the value of a non-separable regularizer without violating resource budget constraints. We develop a primal-dual-type online algorithm for this problem in the online learning setting and confirm its no-regret guarantee and zero constraint violations for stochastic i.i.d. input models. Furthermore, the general convex resource consumption functions allow our model to be more applicable. Numerical experiments are conducted to demonstrate the theoretical guarantee of our algorithm.
更多查看译文
关键词
additively separable rewards,decision maker,general convex resource consumption functions,metrics optimization,network science,nonseparable regularizer,online learning setting,online resource allocation problem,operation research,regularized resource allocation,resource budget constraints,reward maximization,total resource consumption,zero constraint violations
AI 理解论文
溯源树
样例
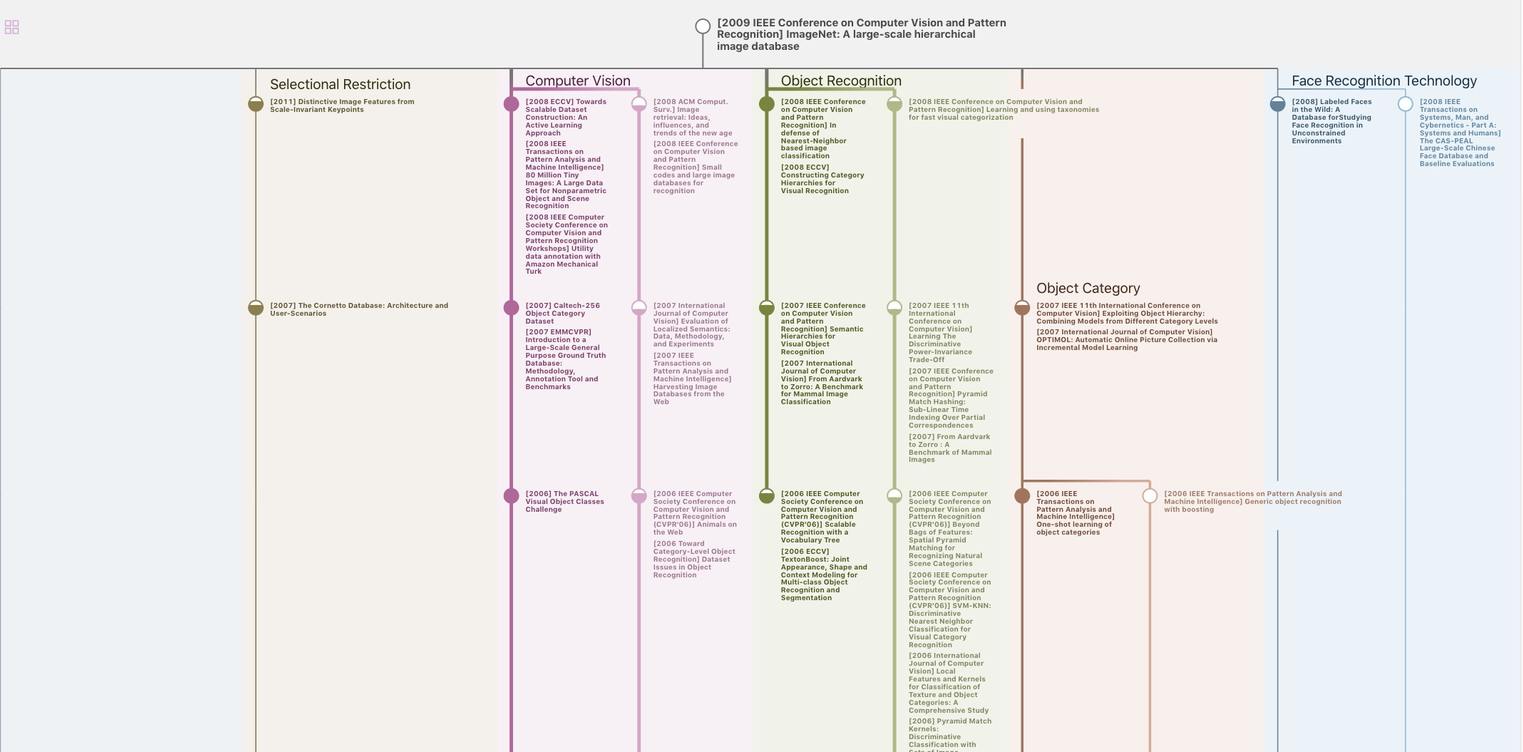
生成溯源树,研究论文发展脉络
Chat Paper
正在生成论文摘要