Improving Disfluency Detection with Multi-Scale Self Attention and Contrastive Learning
ICASSP 2023 - 2023 IEEE International Conference on Acoustics, Speech and Signal Processing (ICASSP)(2023)
摘要
Disfluency detection aims to recognize disfluencies in sentences. Existing works usually adopt a sequence labeling model to tackle this task. They also attempt to integrate into models the feature that the disfluencies are similar to the correct phrase, the so-called "rough copy". However, they heavily rely on hand-craft features or word-to-word match patterns, which are insufficient to precisely capture such rough copy and cause under-tagging and over-tagging problems. To alleviate these problems, we propose a multi-scale self-attention mechanism (MSAT) and design contrastive learning (CL) loss for this task. Specifically, the MSAT leverages token representations to learn representations for different scales of phrases, and then compute similarity among them. The CL adopts the fluent version of the input to build the positive and negative samples and encourages the model to keep the fluent version consistent with the input in semantics. We conduct experiments on a public English dataset Switchboard, and an in-house Chinese dataset Waihu, which is derived from an online conversation bot. Results show that our method outperforms the baselines and achieves superior performance on both datasets.
更多查看译文
关键词
Disfluency detection,multi-scale self-attention,contrastive learning
AI 理解论文
溯源树
样例
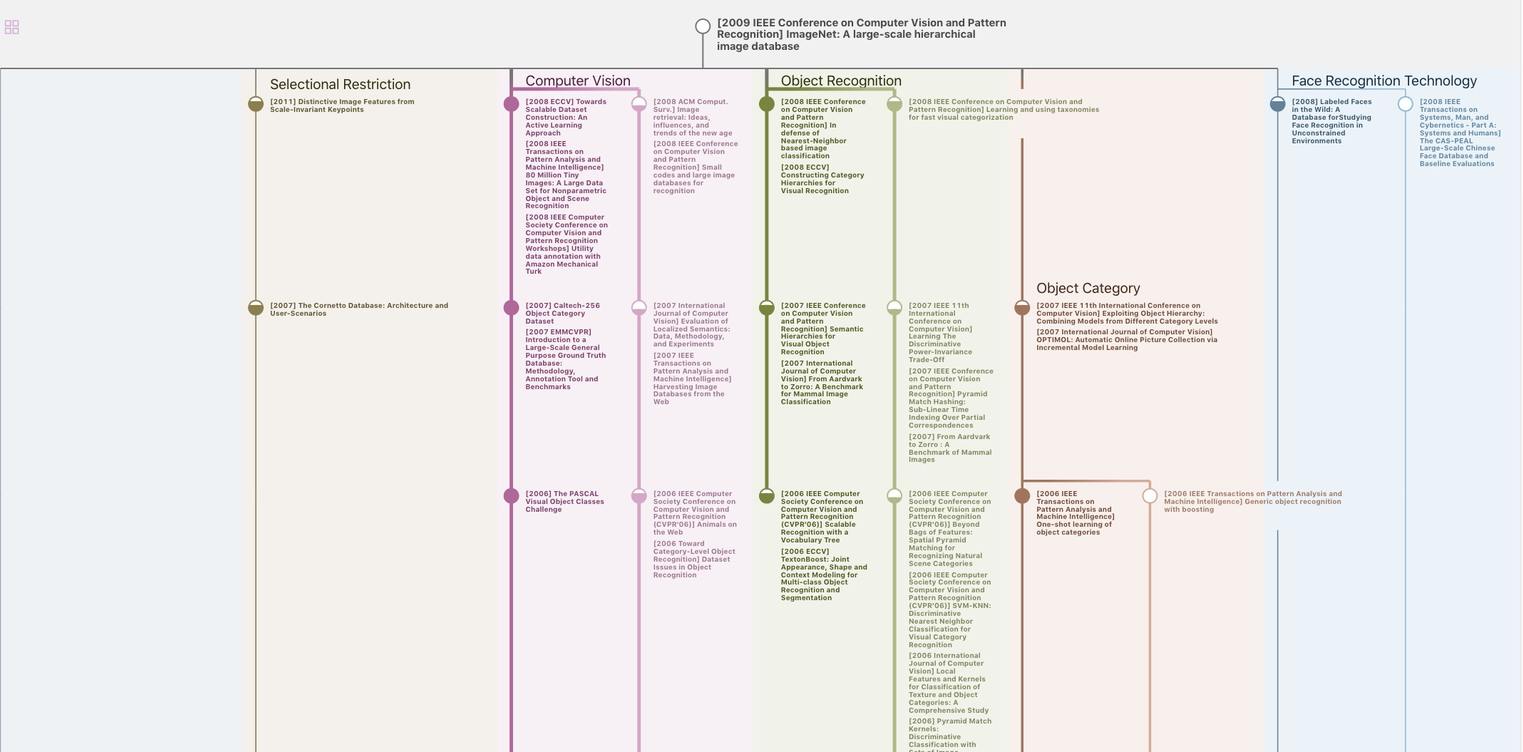
生成溯源树,研究论文发展脉络
Chat Paper
正在生成论文摘要