Label-Efficient and Robust Learning from Multiple Experts
ICASSP 2023 - 2023 IEEE International Conference on Acoustics, Speech and Signal Processing (ICASSP)(2023)
摘要
Learning from multiple sources of labeled data presents unique challenges, including the cost of using many annotators in the training, test, and production time and their limited reliability. In this paper, we analyze the problem of label-efficient learning and propose a method for training a classification system from data labeled by multiple annotators, where only a small subset of them is chosen adaptively to reduce later communication effort and the effect of malicious annotators, especially in the validation and test phase. We define an iterative optimization procedure where the annotation weight vector’s sparsity is enforced while reducing the overall classification error. Using real-world data, we show that our approach can pertain to the classification performance on the complete set of annotators while reducing the need for annotator labels by over 50% on several different tasks. Furthermore, we demonstrate that label sparsity reduces label flip attacks’ effect on performance.
更多查看译文
关键词
annotation weight vector sparsity,classification system,iterative optimization procedure,label-efficient learning,malicious annotators,multiple labeled data sources,robust learning
AI 理解论文
溯源树
样例
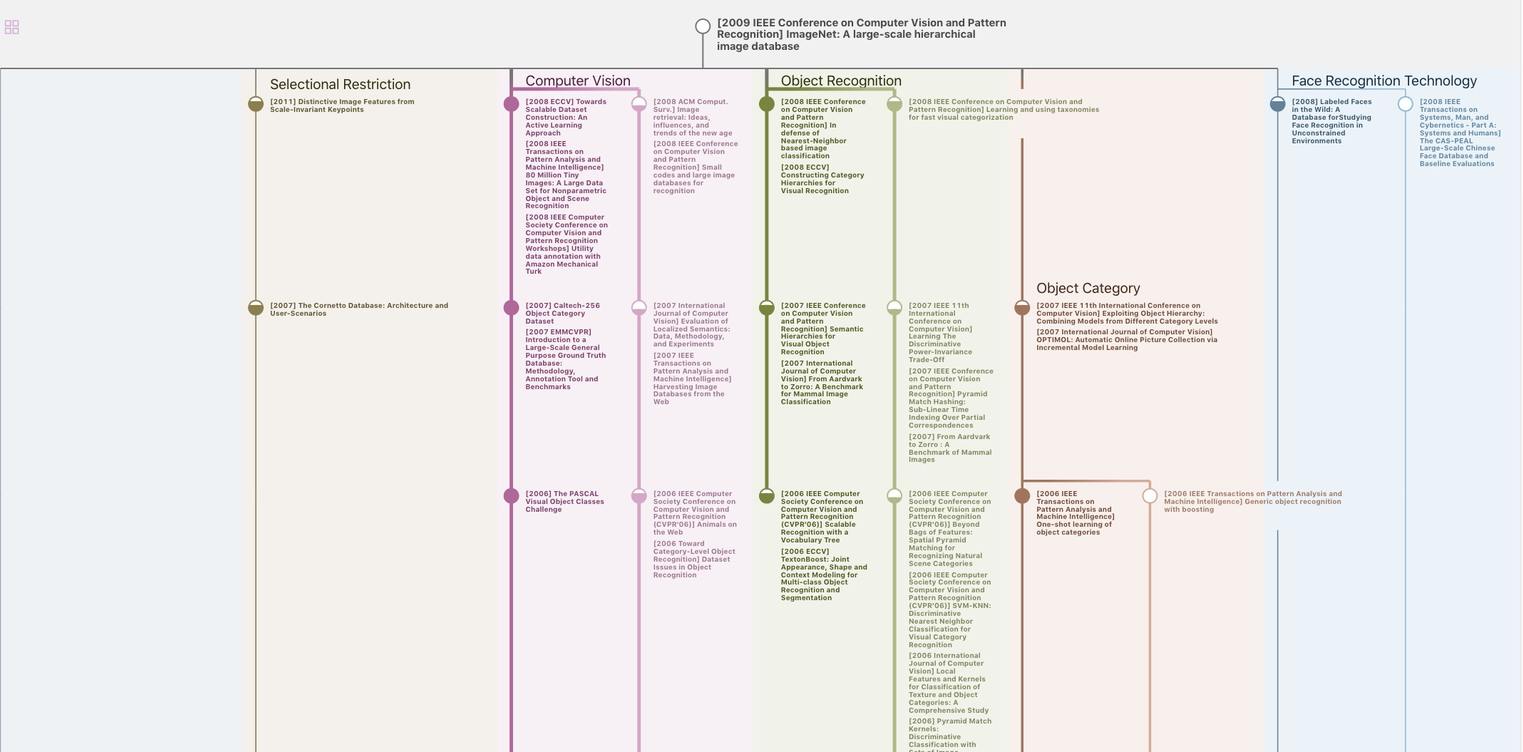
生成溯源树,研究论文发展脉络
Chat Paper
正在生成论文摘要