On Adversarial Robustness of Audio Classifiers
ICASSP 2023 - 2023 IEEE International Conference on Acoustics, Speech and Signal Processing (ICASSP)(2023)
摘要
We make three contributions to improve adversarial robustness of audio classifiers. First, most existing works focus on ℓ
p
-norm bounded adversarial perturbations. Instead, we consider signal-to-noise ratio (SNR) as a more natural measure of adversarial perturbations for audio data. We show that perturbed examples with a particular SNR can be generated using a corresponding ℓ
2
-norm perturbation, and establish the equivalence of these two metrics in assessing adversarial perturbations. This connection enables direct control of the SNR quality of perturbed examples and allows comparison using perturbations with different ℓ
p
-norm constraints. Second, we are among the first to introduce APGD attack for adversarial training on audio data. In our experiments, APGD adversarial training is robust to adversarial attacks without compromising clean accuracy. Last, we improve adversarial robustness by adapting CutMix to audio - cutting and mixing two audio clips together - in conjunction with adversarial training, and observe improvements in robustness on US8K.
更多查看译文
关键词
sound classification,adversarial robustness,data augmentation
AI 理解论文
溯源树
样例
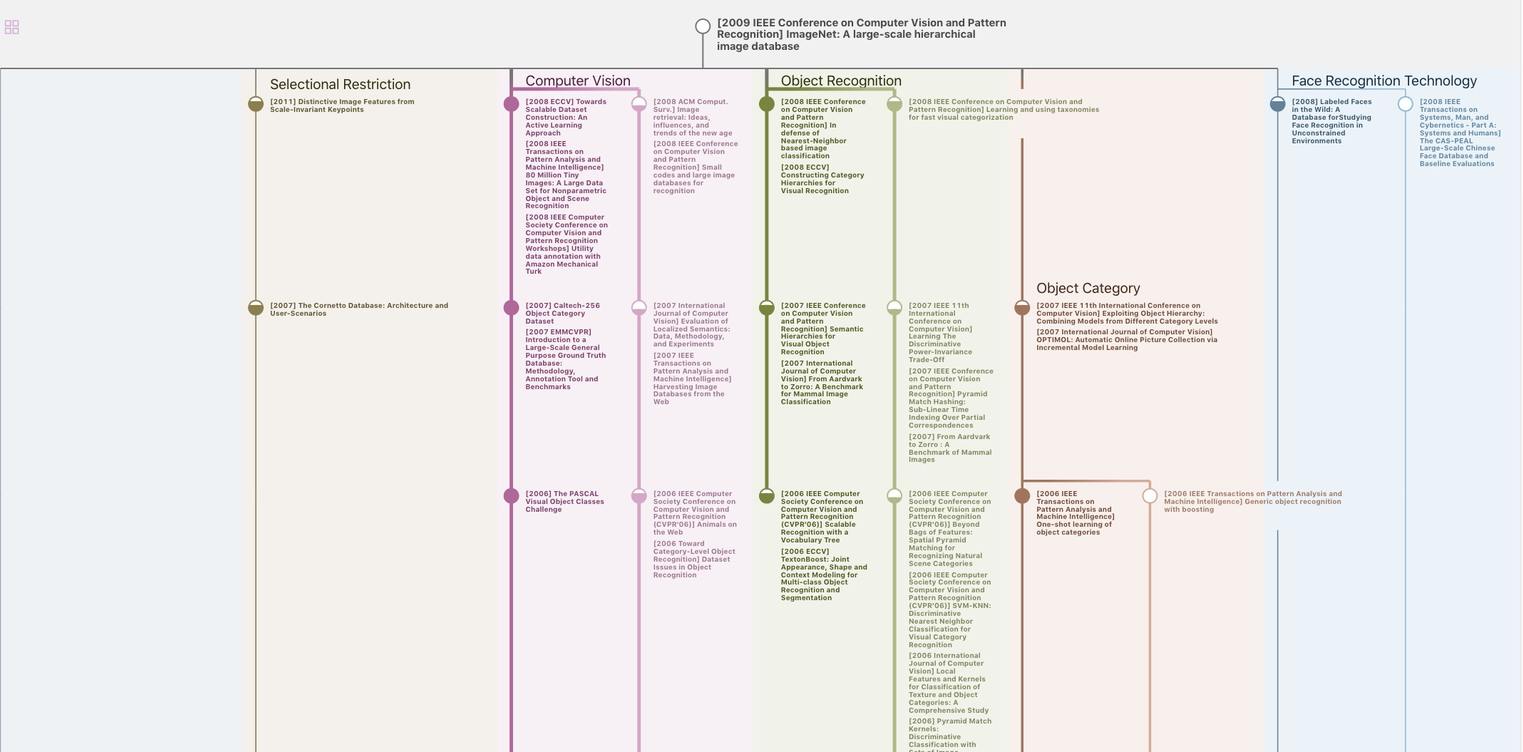
生成溯源树,研究论文发展脉络
Chat Paper
正在生成论文摘要