Matrix Recovery using Deep Generative Priors with Low-Rank Deviations
ICASSP 2023 - 2023 IEEE International Conference on Acoustics, Speech and Signal Processing (ICASSP)(2023)
摘要
In matrix recovery, an unknown matrix can be reconstructed by a small number of limited and noisy measurements. Deep learning-based methods, such as deep generative models, pro-vide stronger priors that can serve to mitigate the pressure of sampling during image recovery. But such methods require that the recovered data be limited to the scope of the generator, otherwise it will lead to large recovery error. To circumvent this problem, in this paper, a framework for matrix recovery from limited measurements is proposed, which employs low rank approximation to characterize the deviation of generator, referred to as Low-Rank-Gen. Theoretically, we propose Matrix Set-Restricted Eigenvalue Condition (M-S-REC), and further prove the existence of decoders and upper bound of reconstruction error using certain number of measurements corresponding to such decoder. Empirically, we observe consistent improvements in reconstruction accuracy, PSNR index over competing approaches.
更多查看译文
关键词
Matrix recovery,deep generative models,Matrix Set-Restricted Eigenvalue Condition,low rank approximation
AI 理解论文
溯源树
样例
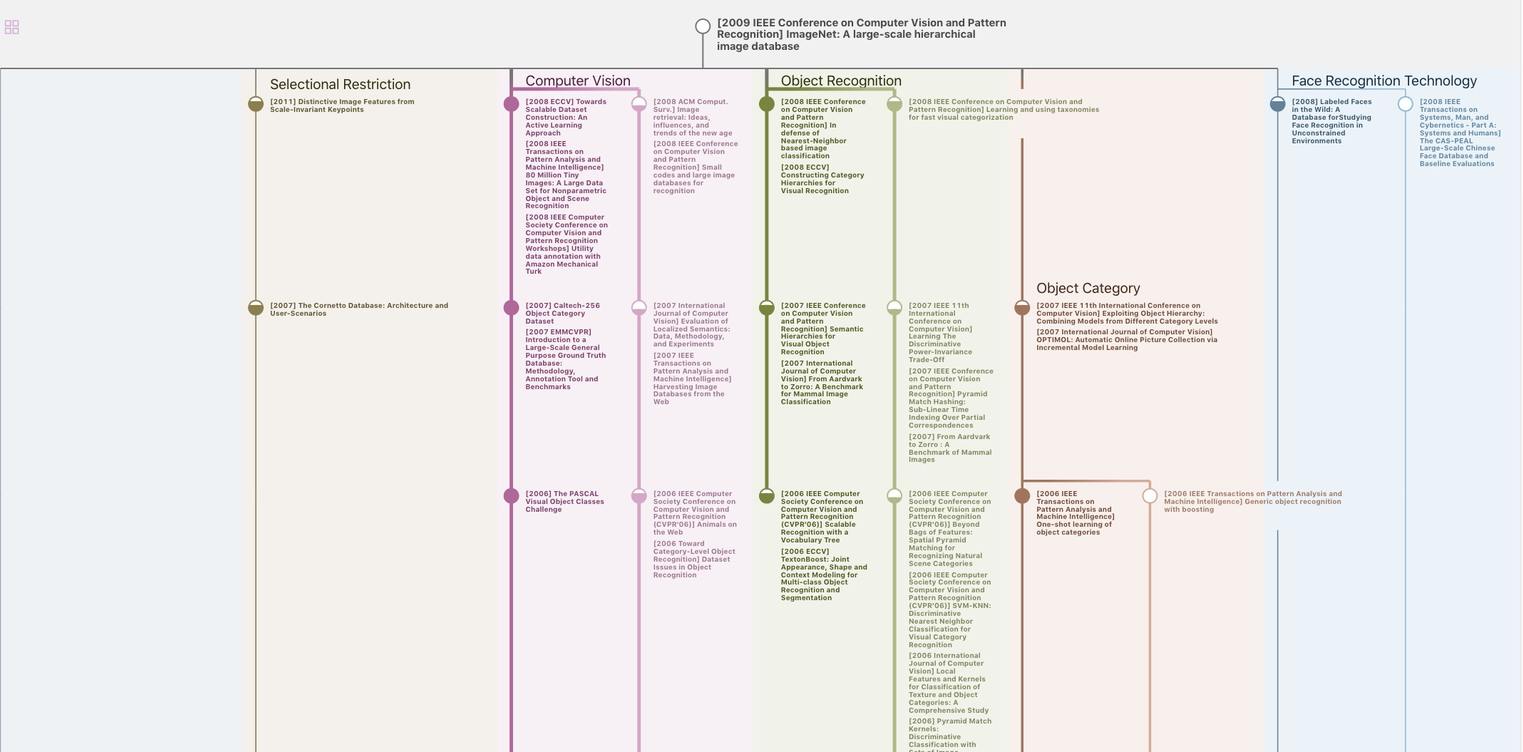
生成溯源树,研究论文发展脉络
Chat Paper
正在生成论文摘要