Semi-Supervised Learning with Per-Class Adaptive Confidence Scores for Acoustic Environment Classification with Imbalanced Data
ICASSP 2023 - 2023 IEEE International Conference on Acoustics, Speech and Signal Processing (ICASSP)(2023)
摘要
In this paper, we concentrate on the per-class accuracy of neural network-based classification in the context of identifying acoustic environments. Even a fully supervised learning framework with an equal amount of data for each class can lead to significant differences in class accuracies. This is then amplified by semi-supervised learning using naturally imbalanced data. To address this problem, we propose an adaptive method for pseudo-label selection via a straightforward optimization of the validation accuracy per class, aimed specifically at reducing the variance between different classes. The proposed method is general and can be applied for both maximum probability and entropy-based confidence criteria. Compared to fully supervised learning as well as state-of-the-art methods for pseudo-labeling, it achieves the lowest variances of per-class accuracy and the highest accuracies of the minority classes when tested on common publicly available environment sound databases.
更多查看译文
关键词
Semi-supervised learning,deep learning,neural networks,pseudo-labeling,sound classification
AI 理解论文
溯源树
样例
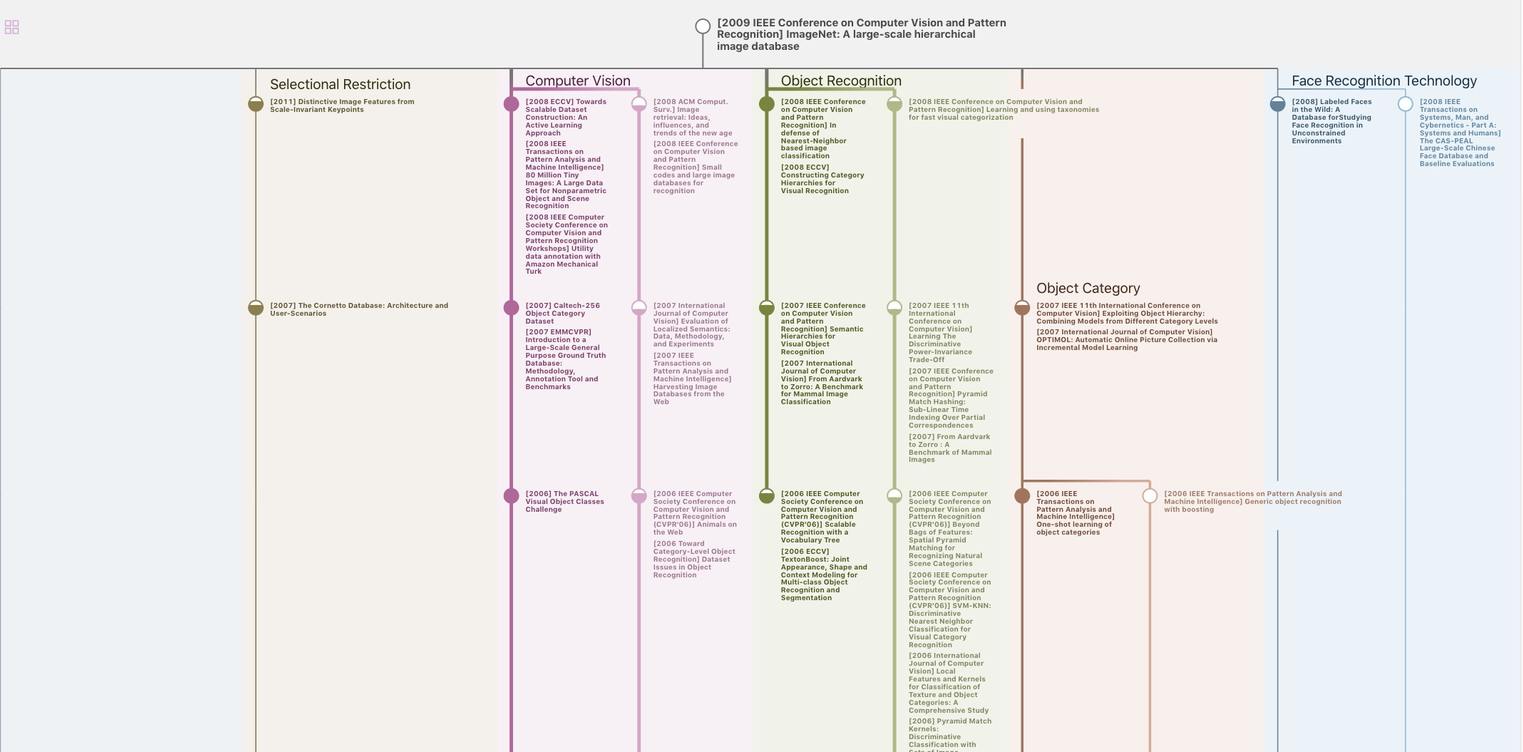
生成溯源树,研究论文发展脉络
Chat Paper
正在生成论文摘要