Affinity Learning With Blind-Spot Self-Supervision for Image Denoising
ICASSP 2023 - 2023 IEEE International Conference on Acoustics, Speech and Signal Processing (ICASSP)(2023)
摘要
In this paper, we extend the blind-spot based self-supervised denoising by using affinity learning to remove noise from affected pixels. Inspired by inpainting, we introduce a novel Mask Guided Residual Convolution (MGRConv) to learn a neighboring image pixel affinity map that gradually removes noise and refines blind-spot denoising process. We show that mask convolution plays an important role in blind-spot denoising since it is theoretically aligned with $\mathcal{J} - invariance$, which blind-spot based self-supervised denoising frameworks are built upon. The theoretical analysis further shows the motivation behind using more adaptive mask convolutions. Our MGRConv not only enables dynamic mask learning without external trainable parameters, but also preserves appropriate mask constraints by sigmoid activation and residual summation. Our MGRConv is a balance between partial convolution and learnable attention maps, and boosts denoising performance better than other inpainting convolutions with similar or even less parameters, memory, and training/inference time. Extensive experiments show that our proposed plug-and-play MGRConv can assist blind-spot based denoising networks to reach promising results on both existing single-image based and dataset based benchmarks.
更多查看译文
关键词
image denoising,affinity learning,blindspot denoising
AI 理解论文
溯源树
样例
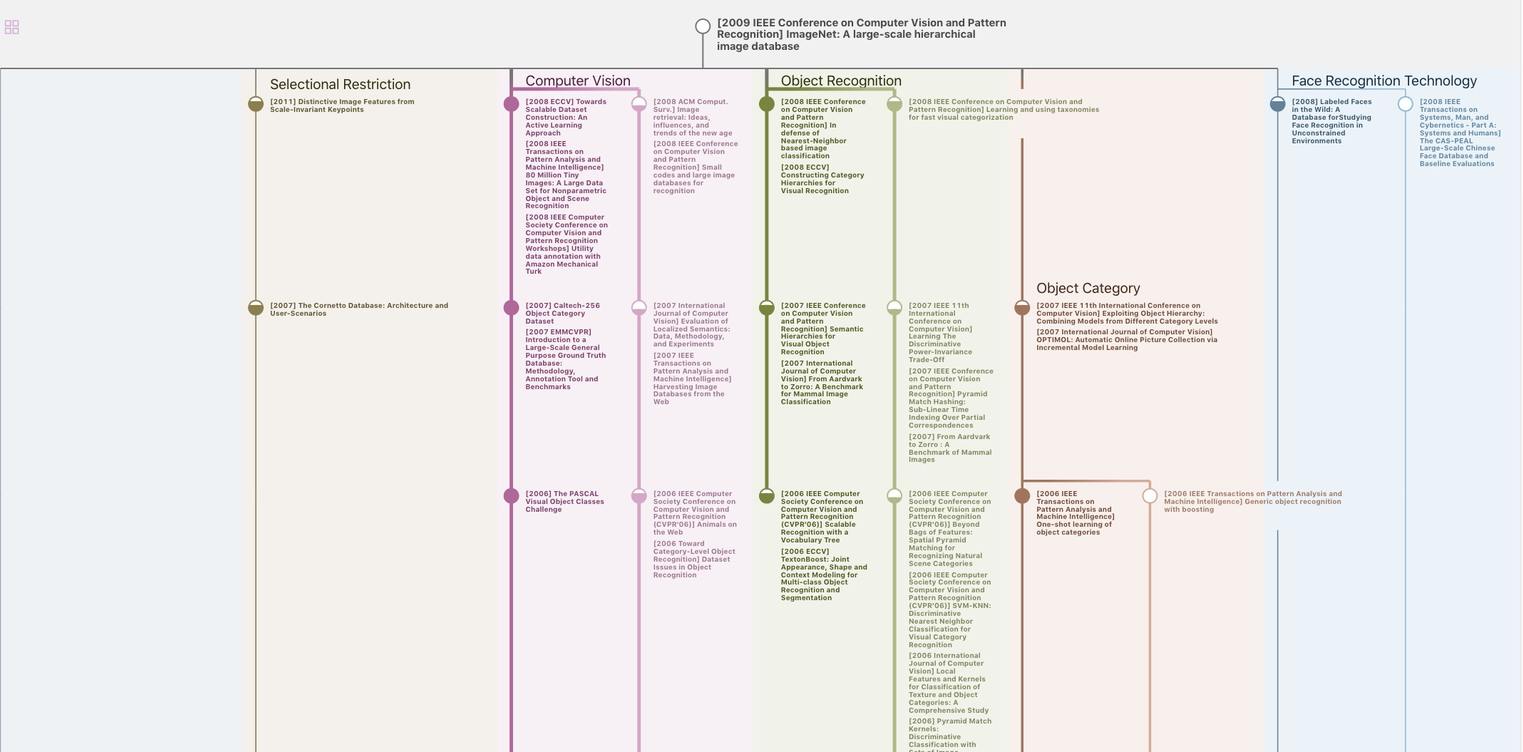
生成溯源树,研究论文发展脉络
Chat Paper
正在生成论文摘要