Optimized Human Drowsiness Detection using Spatial and Temporal features of Eye and Mouth
2023 10th International Conference on Signal Processing and Integrated Networks (SPIN)(2023)
摘要
Drowsy drivers can cause casualties, grave injuries, and fimancial waste; thus, it is essential to have a mechanism that detects drowsiness among drivers. This paper presents a compressed non-intrusive drowsiness monitoring system in three phases. In phase 1, we target the detection of human drowsiness using a set of state-of-the-art machine learning architectures and a custom architecture by using the state of eye and mouth regions from a combination of the MRL Eye and YawDD datasets. Out of VGG-16 (97% test accuracy), Xception (98%), MobileNet (97%), InceptionV3 (98%), and custom architecture (98%), the custom architecture is selected to get carried forward to the subsequent phases because it gives comparable results to deeper models with 100 times fewer parameters. The satisfactory performance of this model was also verified on a selective subset of the NTHU-DDD video dataset. In phase 2, the custom architecture is employed to extract appearance-related features from a set of frames used by an LSTM network to learn motion-related characteristics. The CNN+LSTM model gives 98% test accuracy on the mouth test dataset. In phase 3, to target an embedded board implementation, we used the knowledge distillation technique where the thinned-out version of custom architecture (student network) to get trained under the guidance of Xception (teacher network). Under this experimental phase, the accuracy of the student network got increased from 92% to 96.4% on eye detection and from 97% to 98% on mouth detection.
更多查看译文
关键词
Machine Learning,Deep Learning,CNN,LSTM,Knowledge Distillation
AI 理解论文
溯源树
样例
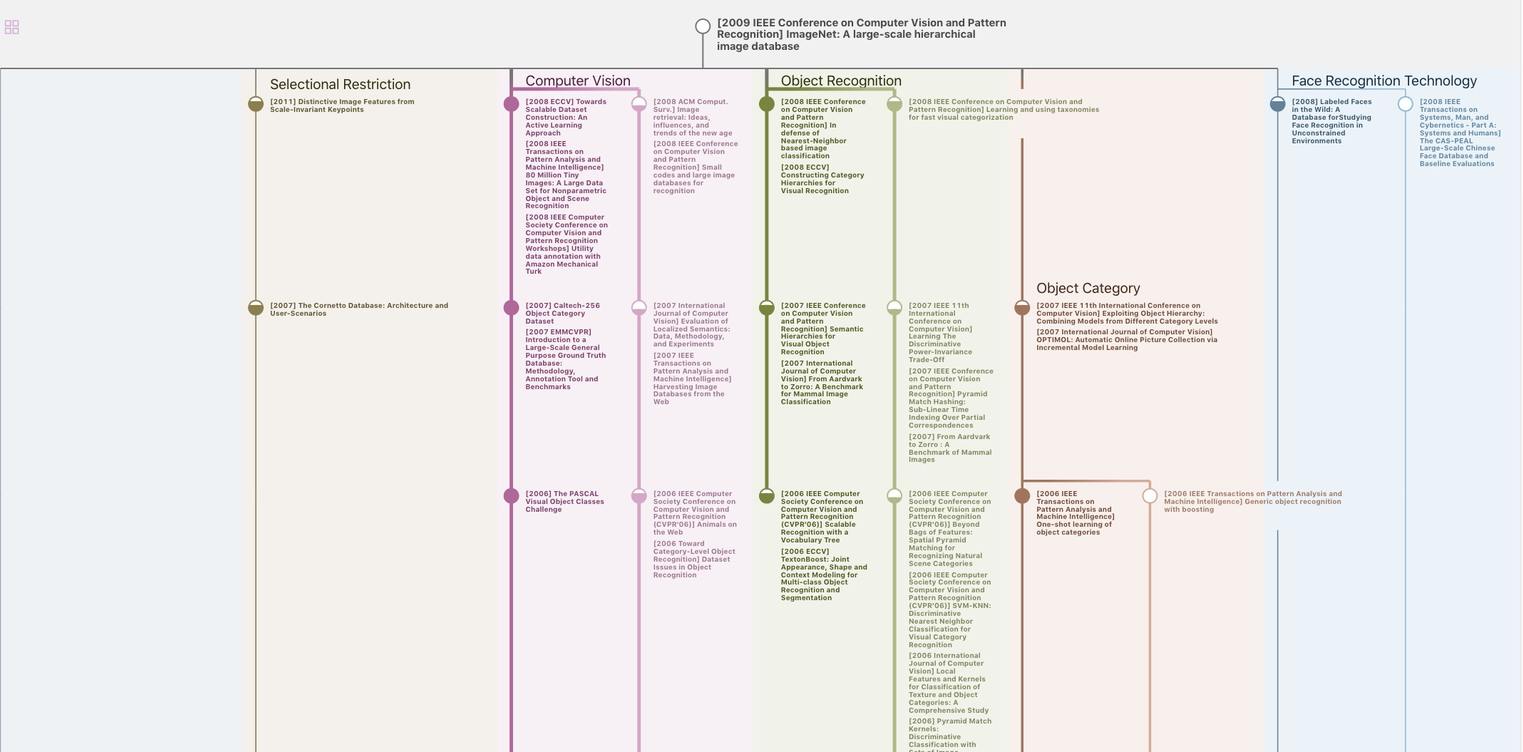
生成溯源树,研究论文发展脉络
Chat Paper
正在生成论文摘要