Stress Detection Through Single Channel Real-Time EEG Signal from Temporal Lobe Using Machine Learning Model
2023 14th International Conference on Computing Communication and Networking Technologies (ICCCNT)(2023)
摘要
Electroencephalography (EEG) is a prompt method for brain signal recording with good temporal resolution, being comparatively cheaper and portable. Stress detection to help facilitate psychological analysis will increase its certainty and accelerate it. The aim of the research is to validate a real-time dataset built with the objective of simplicity and instantaneous detection of stress through recording of EEG signal. In light of that, a dataset was built using only a single channel device of 200 Hz sampling frequency and recording from temporal lobe (T3 and T5) was taken for a short time (30-50 seconds). The EEG data was pre-processed using a bandpass filter and used as a whole to extract features. As FFT is not able to comprehend the non-stationary nature of the data, FFT along with Power Spectral Density (PSD) as features acquires an accuracy of 72.7%. A better feature to properly incorporate the non-stationary characteristics of EEG data, Wavelet Transform was used to acquire an accuracy of 86.96% in Random Forest machine learning classifier. Finally, an accuracy of 97.7% is achieved by incorporating all three features at a time in Random Forest classifier.
更多查看译文
关键词
EEG,Power Spectral Density,Wavelet Transform,Feature Extraction,Machine Learning
AI 理解论文
溯源树
样例
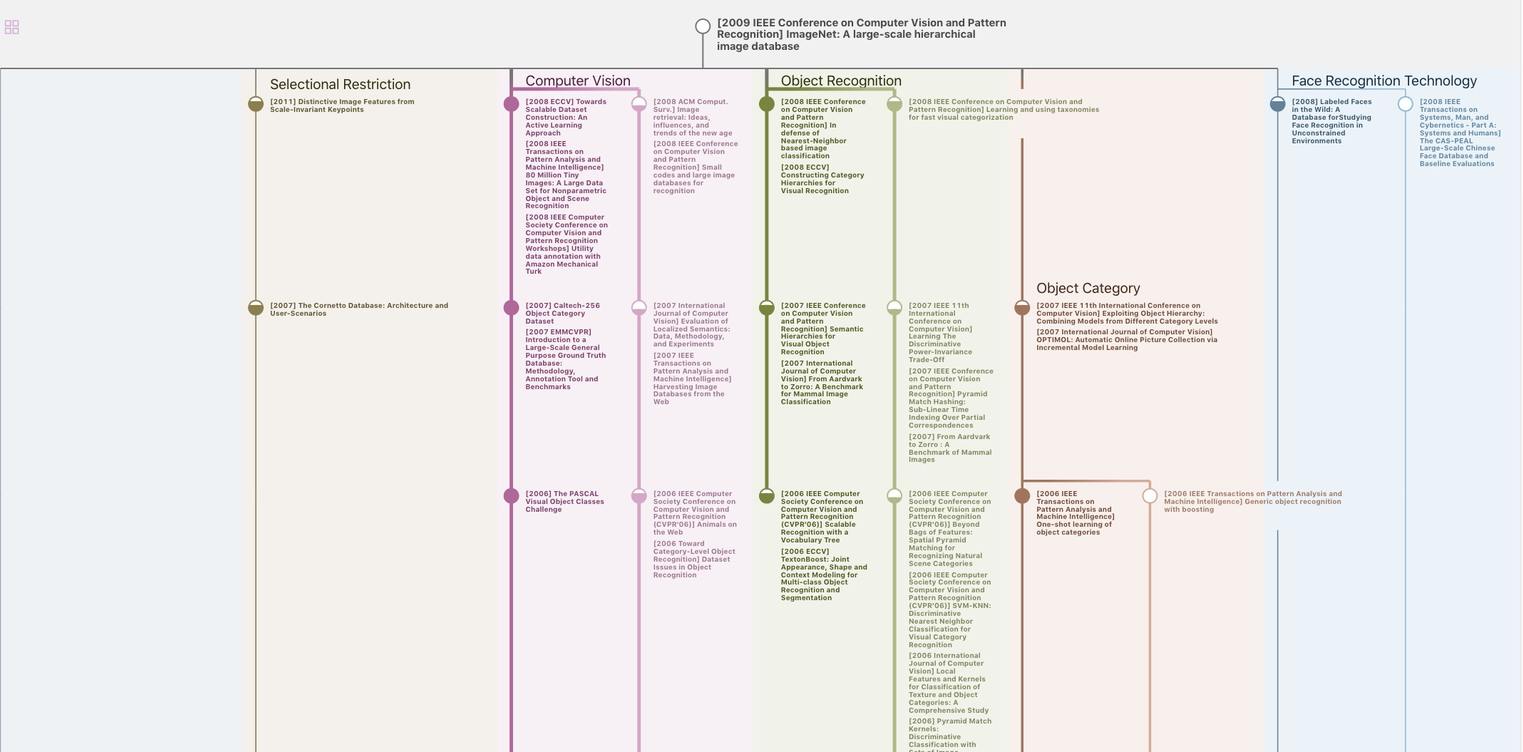
生成溯源树,研究论文发展脉络
Chat Paper
正在生成论文摘要