Using Machine Learning to Predict Patient Health Outcomes
2023 14th International Conference on Computing Communication and Networking Technologies (ICCCNT)(2023)
摘要
Predicting patient health outcomes accurately is a key challenge in modern healthcare, significantly impacting treatment planning, patient management, and healthcare resource allocation. In this study, we propose an innovative approach for predicting patient health outcomes using federated machine learning (FedML), a novel machine learning technique that enables collaborative learning from decentralized data sources while ensuring privacy. This research advances current methodologies by enabling data analysis across diverse health institutions without explicit data sharing. The goal is to develop a robust model capable of capturing a wide spectrum of health factors impacting patient outcomes, thus potentially leading to more accurate predictions. Our approach integrates data from multiple healthcare institutions to train a global predictive model, iterating between local model training and global model aggregation until convergence. We also employ a privacy-preserving mechanism to ensure the sensitive health data remains within the original institution, alleviating privacy and data security concerns inherent in traditional centralized learning methods. We assessed our federated learning strategy in contrast to well-established machine learning techniques such as deep learning, support vector machines, and random forests by using a range of patient datasets. Our technique has been shown to increase prediction performance, generalizability, and scalability while maintaining data privacy, as shown by the results. The study concludes by discussing the potential of federated learning in transforming predictive modeling in healthcare and addressing key challenges in implementing this approach. This research contributes to the body of knowledge in predictive health informatics and provides a roadmap for implementing federated machine learning in healthcare applications.
更多查看译文
关键词
Patient Health Outcomes,Prediction,Health-care,Electronic Health Records,Support Vector Machines,Decision Trees
AI 理解论文
溯源树
样例
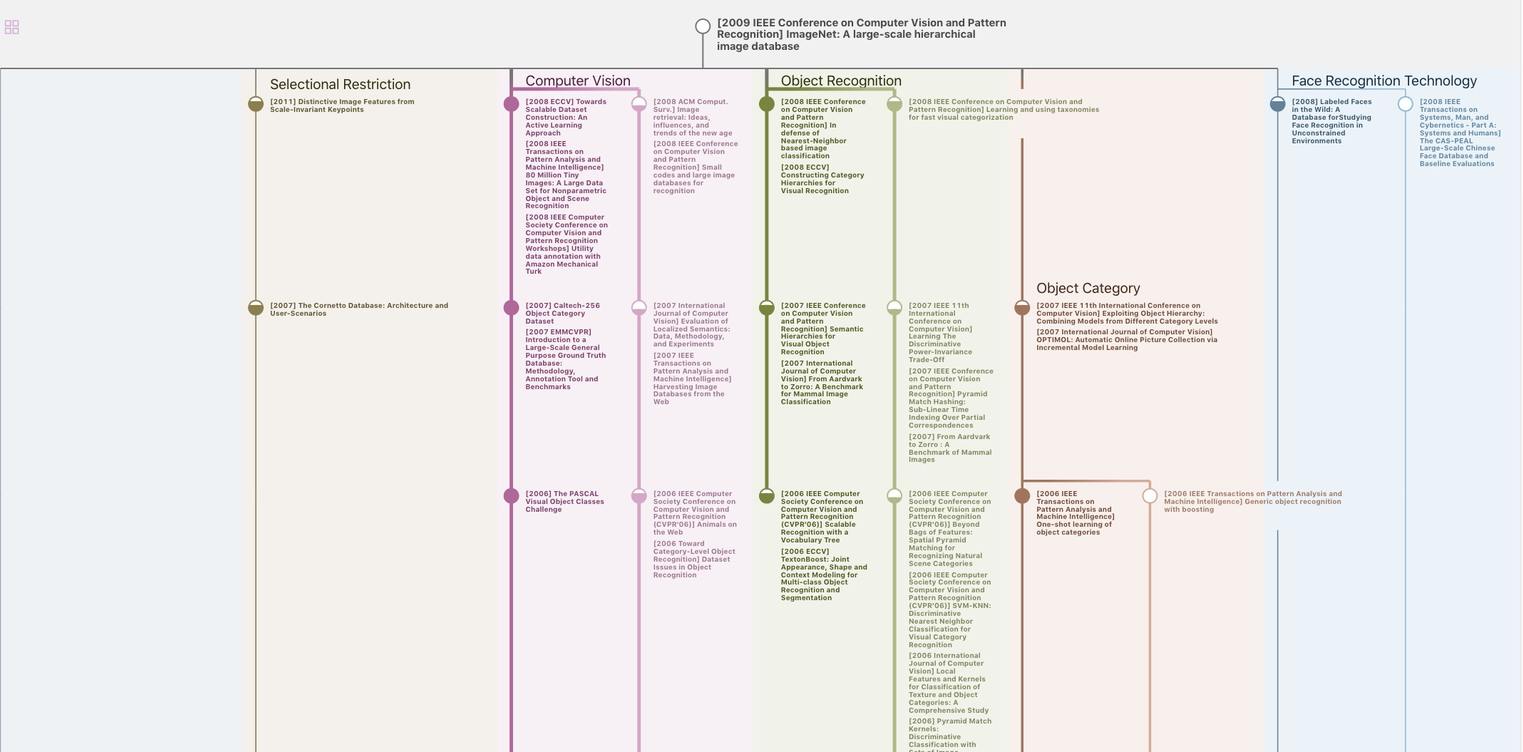
生成溯源树,研究论文发展脉络
Chat Paper
正在生成论文摘要