Ensemble machine-learning model for solar radiation prediction using explainable AI
2023 14th International Conference on Computing Communication and Networking Technologies (ICCCNT)(2023)
摘要
Solar radiation prediction is crucial in many contexts, including solar energy production, climate and weather research, and agricultural planning. Accurate and timely solar radiation estimation opens up opportunities for effective solar energy planning and optimization. Even though a number of machine learning algorithms have been put forth in the literature to predict solar radiation, explainable AI (XAI) has not yet been thoroughly investigated. In this study, we are focusing on filling some research gaps in the performance analysis of ensemble methods for predicting solar radiation based on explainable AI. The research aims to achieve a balance between model interpretability and prediction accuracy by exploring ensemble techniques that go beyond conventional approaches. Additionally, by exploring ensemble-based methods and incorporating explainable AI techniques, we intend to improve prediction accuracy while providing interpretable insights for decision-making in solar energy applications. The results have been reported in terms of R2 Score, mean absolute error (MAE), and root mean squared error (RMSE).
更多查看译文
关键词
Solar radiation prediction,Ensemble methods,Explainable AI,Performance analysis
AI 理解论文
溯源树
样例
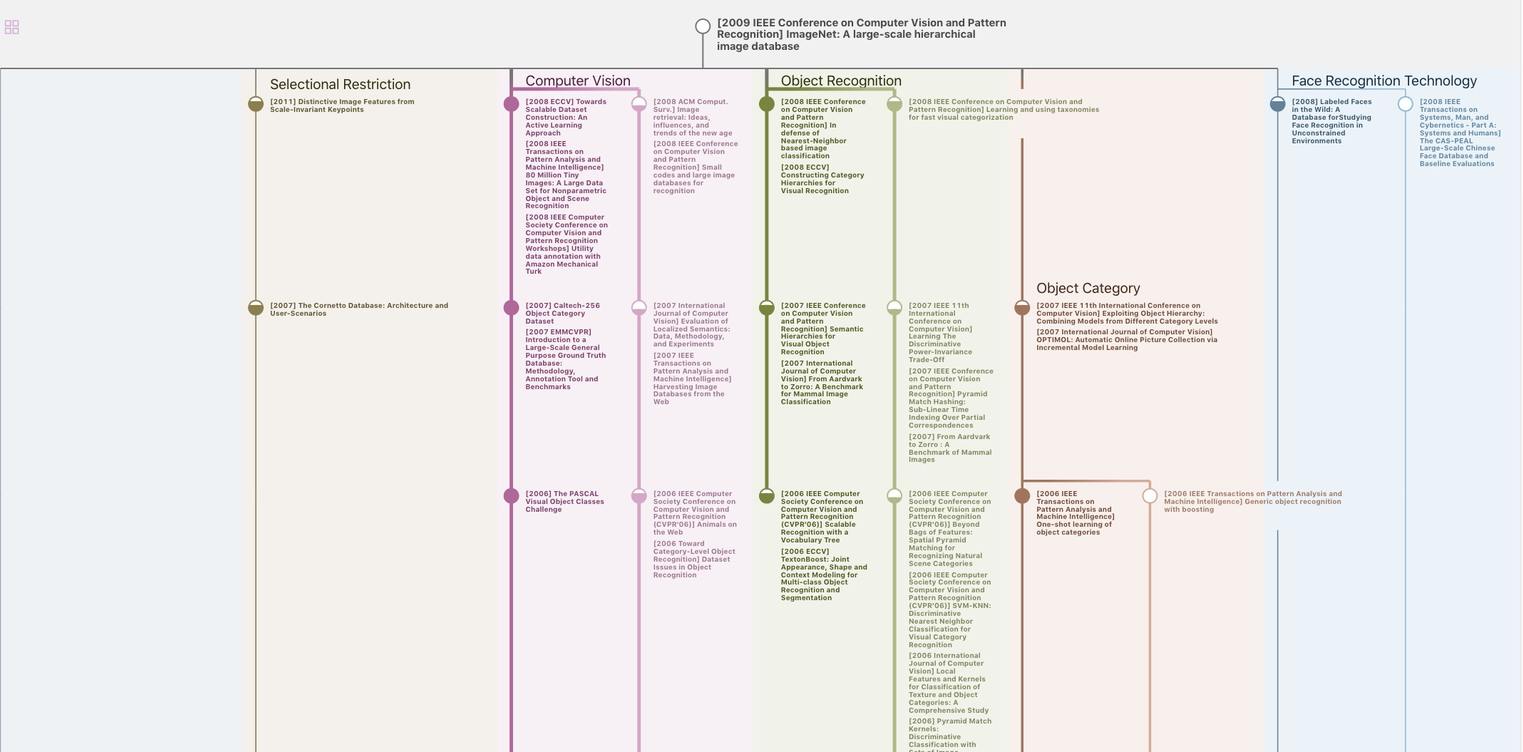
生成溯源树,研究论文发展脉络
Chat Paper
正在生成论文摘要