Graph CNNs with Motif and Variable Temporal Block for Skeleton-based Action Recognition
THIRTY-THIRD AAAI CONFERENCE ON ARTIFICIAL INTELLIGENCE / THIRTY-FIRST INNOVATIVE APPLICATIONS OF ARTIFICIAL INTELLIGENCE CONFERENCE / NINTH AAAI SYMPOSIUM ON EDUCATIONAL ADVANCES IN ARTIFICIAL INTELLIGENCE(2019)
摘要
Hierarchical structure and different semantic roles of joints in human skeleton convey important information for action recognition. Conventional graph convolution methods for modeling skeleton structure consider only physically connected neighbors of each joint, and the joints of the same type, thus failing to capture high-order information. In this work, we propose a novel model with motif-based graph convolution to encode hierarchical spatial structure, and a variable temporal dense block to exploit local temporal information over different ranges of human skeleton sequences. Moreover, we employ a non-local block to capture global dependencies of temporal domain in an attention mechanism. Our model achieves improvements over the stateof-the-art methods on two large-scale datasets.
更多查看译文
关键词
Skeleton-Based Recognition,Spatiotemporal Features,Silhouette Analysis,Action Recognition,Novelty Detection
AI 理解论文
溯源树
样例
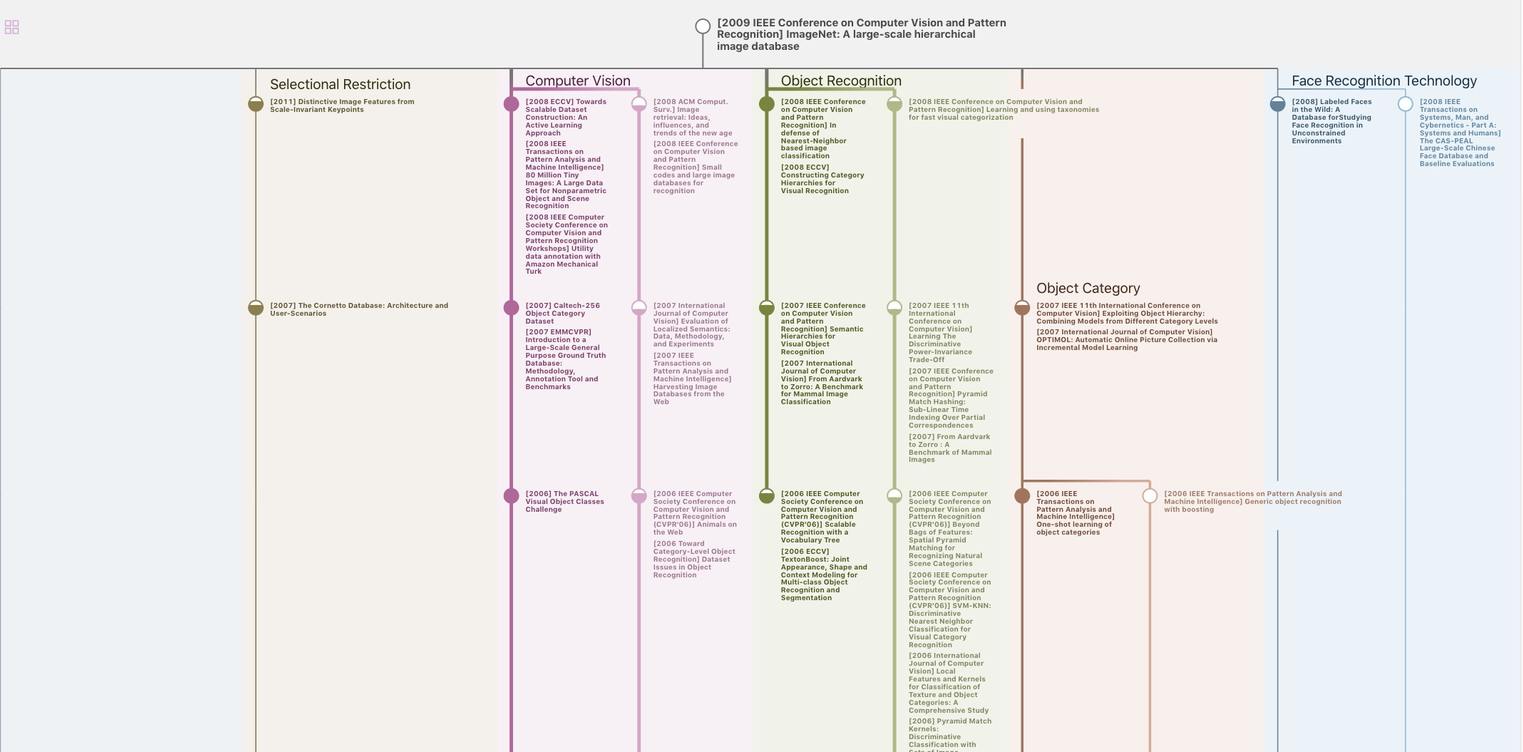
生成溯源树,研究论文发展脉络
Chat Paper
正在生成论文摘要