GAF and CBAM-ResNet: An Efficient Combination for Identifying the Degree of Safety Valve Leakage
2023 CAA Symposium on Fault Detection, Supervision and Safety for Technical Processes (SAFEPROCESS)(2023)
摘要
This study proposes a combined method according to Gramian Angle Field (GAF) image encoding and a deep residual neural network with the Convolution Block Attention Module (CBAM) to identify the leakage degree of safety valves used in pipeline transportation systems. In the proposed method, the raw Acoustic Emission (AE) signal from safety valves is transformed into a two-dimensional image using GAF encoding emthod. The image features related to the leakage degree are extracted and classified by the ResNet-50 model with the CBAM attention mechanism. The study compares the performance differences between Gramian Angular Summation Fields (GASF) and Gramian Angular Difference Fields (GADF) in encoding AE signals and analyzes the effect of adding the CBAM attention mechanism to ResNet-50. The experimental results show that GASF outperforms GADF in highlighting image features of AE signals. The CBAM-based ResNet-50 achieves a stable accuracy of 96.5% in identifying the leakage degree and provides a faster convergence speed.
更多查看译文
关键词
safety valve,acoustic emission,leakage degree identification,Gramian Angle Field,convolution block attention module
AI 理解论文
溯源树
样例
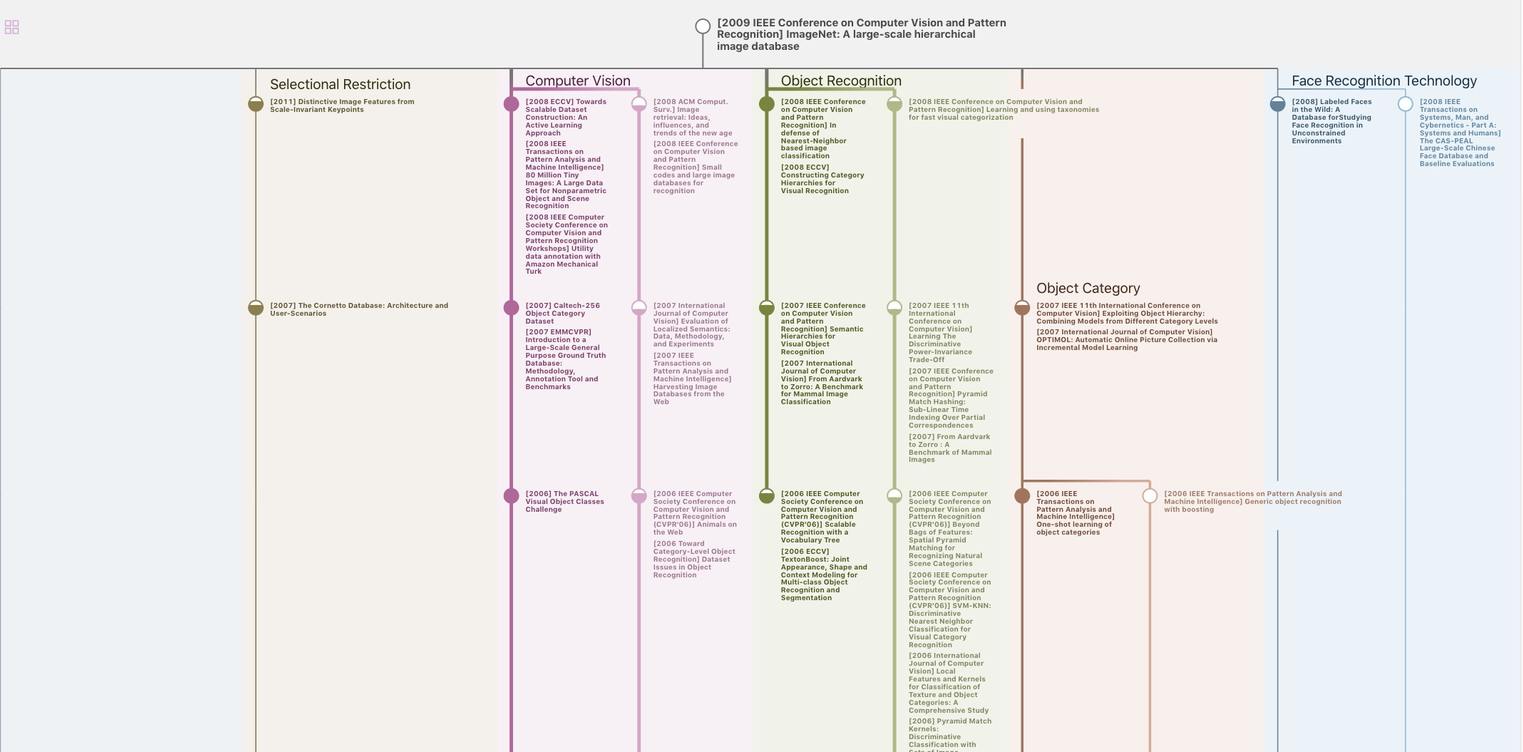
生成溯源树,研究论文发展脉络
Chat Paper
正在生成论文摘要