Enhancing Robustness in Multi-modal CT Image Segmentation with a Clustering-based Deep Active Learning Approach
2023 CAA Symposium on Fault Detection, Supervision and Safety for Technical Processes (SAFEPROCESS)(2023)
Key words
multi-modality,deep active learning,lesion segmentation
AI Read Science
Must-Reading Tree
Example
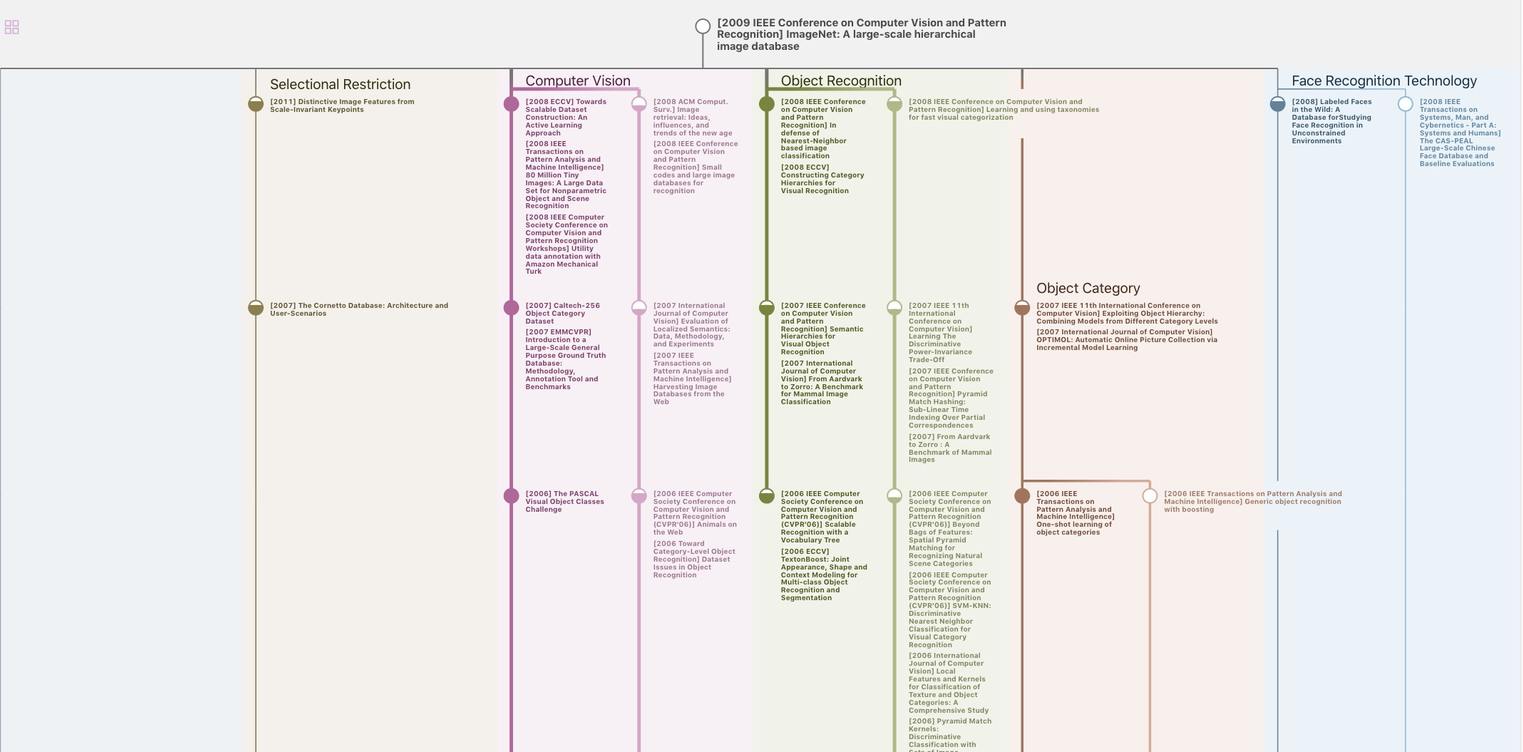
Generate MRT to find the research sequence of this paper
Chat Paper
Summary is being generated by the instructions you defined