Spatial and Spectral Structure Preserved Self-Representation for Unsupervised Hyperspectral Band Selection
IEEE TRANSACTIONS ON GEOSCIENCE AND REMOTE SENSING(2023)
摘要
As an effective manner to reduce data redundancy and processing inconvenience, hyperspectral band selection aims to select a subset of informative and discriminative bands from the original data cube. Although a large number of approaches have been proposed and obtained great success, they still face at least two issues. First, most of the previous methods only consider the redundancy between neighbor bands, while the global information has been ignored. Second, each band is often treated as a whole and reshaped to a feature vector without considering the spatial structure of different regions. In this article, in order to address these issues, we propose a spatial and spectral structure preserved self-representation model for unsupervised hyperspectral band selection without using any label information, referred to as S4P briefly. Different from previous methods that stretch each band into a feature vector, the first principal component of the original hyperspectral cube is segmented into different superpixels, which can reflect the spatial structure of homogeneous regions. Then each band can be represented by a superpixel-level feature vector, and the self-representation model is utilized to learn the spectral correlation of different bands. In addition, an adaptive and weighted multiple graph fusion term is designed to generate a unified similarity graph between different superpixels, which is used to capture the spatial structure in the self-representation space. Finally, an l(2, 1)-norm is imposed on the self-representation coefficient matrix to measure the band importance. We design an alternative update scheme to optimize the resultant problem, the self-representation coefficient matrix and the superpixel-wise similarity graph can boost each other during the updating process to obtain optimal results. Extensive experiments with detailed analysis of three public datasets are conducted to validate the superiority of the proposed S4P when compared with other state-of-the-art competitors.
更多查看译文
关键词
Hyperspectral imaging,Feature extraction,Correlation,Adaptation models,Redundancy,Principal component analysis,Image segmentation,Clustering,graph fusion,hyperspectral band selection,similarity graph learning
AI 理解论文
溯源树
样例
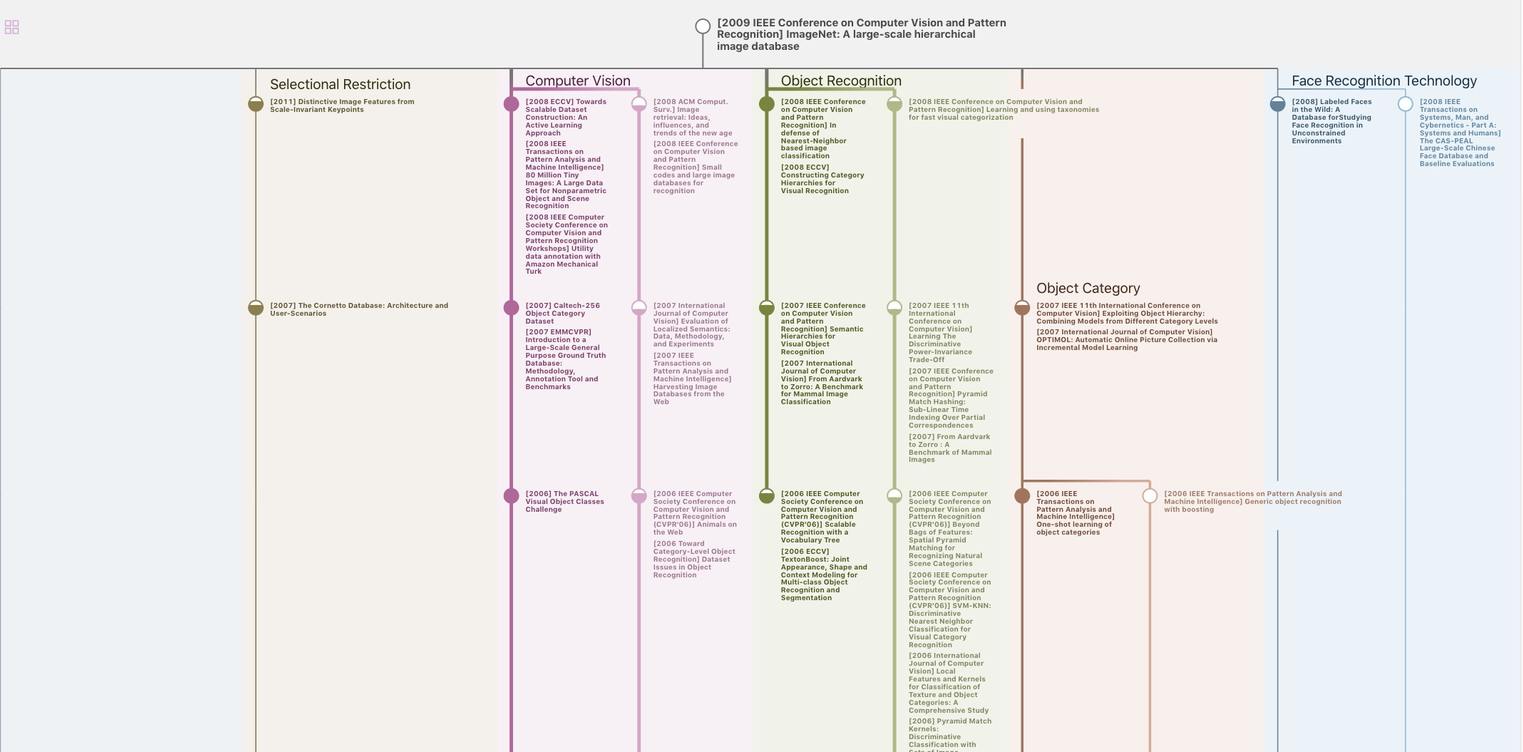
生成溯源树,研究论文发展脉络
Chat Paper
正在生成论文摘要