CMTP-TCE: A Data Augmentation Method of Electronic Tongue Combined With Dot-Product Attention Mechanism and Residual Network for Food Quality Classification
IEEE Sensors Journal(2023)
摘要
The electronic tongue (e-tongue) is a bionic-based taste detection instrument that shows a promising prospect for food quality classification. However, in the current research, food quality classification with the e-tongue is heavily dependent on the labeled training data, which is time-consuming for data collection in practical applications and affects the generalization performance of the models for food quality classification. Data augmentation can increase the diversity of training samples, thus improving the generalization performance of the model. Therefore, in this study, a data augmentation method for e-tongue, namely, a computational model of taste pathways and time-channel expansion (CMTP-TCE), is proposed on nerve conduction mechanisms to improve the performance for food quality classification. To achieve excellent classification, a dot-product attention mechanism (DPAM) and a residual network (ResNet) are designed to process the CMTP-TCE output. The results show that, first, the number and size of taste information samples are expanded using the CMTP-TCE. Second, compared with the identification results of tea and apple samples by traditional taste analysis and other data augmentation methods, the best classification results are obtained using the CMTP-TCE. The applicability and effectiveness of the CMTP-TCE for data augmentation are validated. Finally, compared with the identification results of multiple models and ablation studies, the best accuracy, F1-scores, and kappa coefficients for tea and apple recognition are obtained by the DPAM-ResNet. DPAM-ResNet shows the best classification performance and stability. In conclusion, excellent food quality classification is achieved using the CMTP-TCE and DPAM-ResNet models.
更多查看译文
关键词
electronic tongue combined,data augmentation method,cmtp-tce,dot-product
AI 理解论文
溯源树
样例
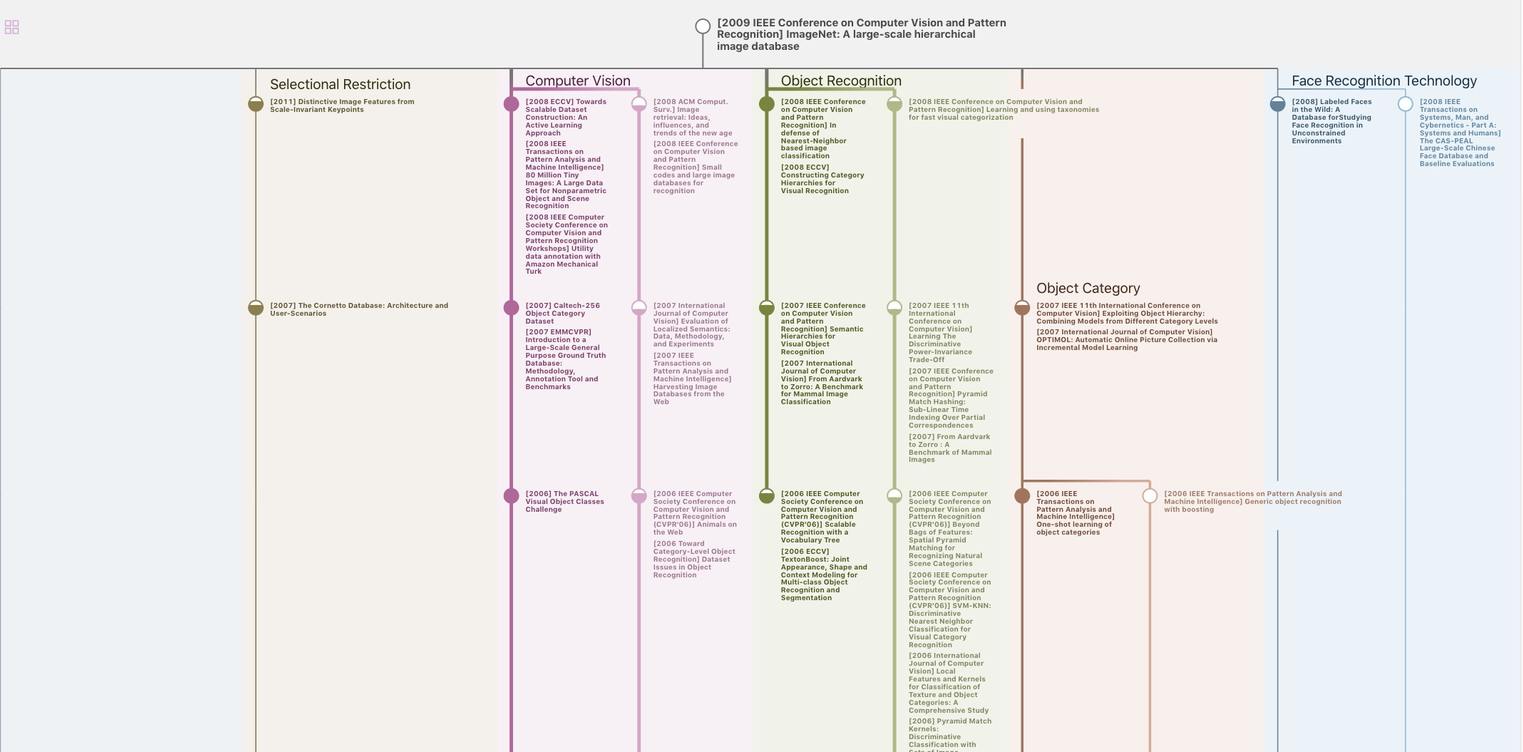
生成溯源树,研究论文发展脉络
Chat Paper
正在生成论文摘要