Magnetic Anomaly Detection Network With Adaptive Time–Frequency Feature Expression
IEEE Sensors Journal(2023)
摘要
Magnetic anomaly detection (MAD) is a method that uses magnetometers to find hidden ferromagnetic objects based on variations in magnetic field signals. Many methods use time–frequency fusion features to detect target signals, but these features are extracted using unlearnable methods in the preprocessing. This article proposes a network with adaptive time–frequency feature expression to detect magnetic anomalies. The network adaptively learns the time–frequency features of magnetic anomaly signal through optimization, and in the feature fusion step, it adaptively selects key features for classification. In experiments, we compared our proposed method with the state-of-the-art (SOTA) time–frequency fusion detection method in simulation, semireal, and real datasets. Results show that our method has better detection performance, with high accuracy (ACC), detection rate (DR), and area under receiver operating characteristic curve (AUC). In real datasets, the proposed method outperforms other methods in low signal-to-noise ratio (SNR) cases and has a low false alarm rate (FAR), demonstrating its good comprehensive detection ability and robustness.
更多查看译文
关键词
Classification based on magnetometer data, feature fusion, magnetic anomaly detection (MAD), neural network
AI 理解论文
溯源树
样例
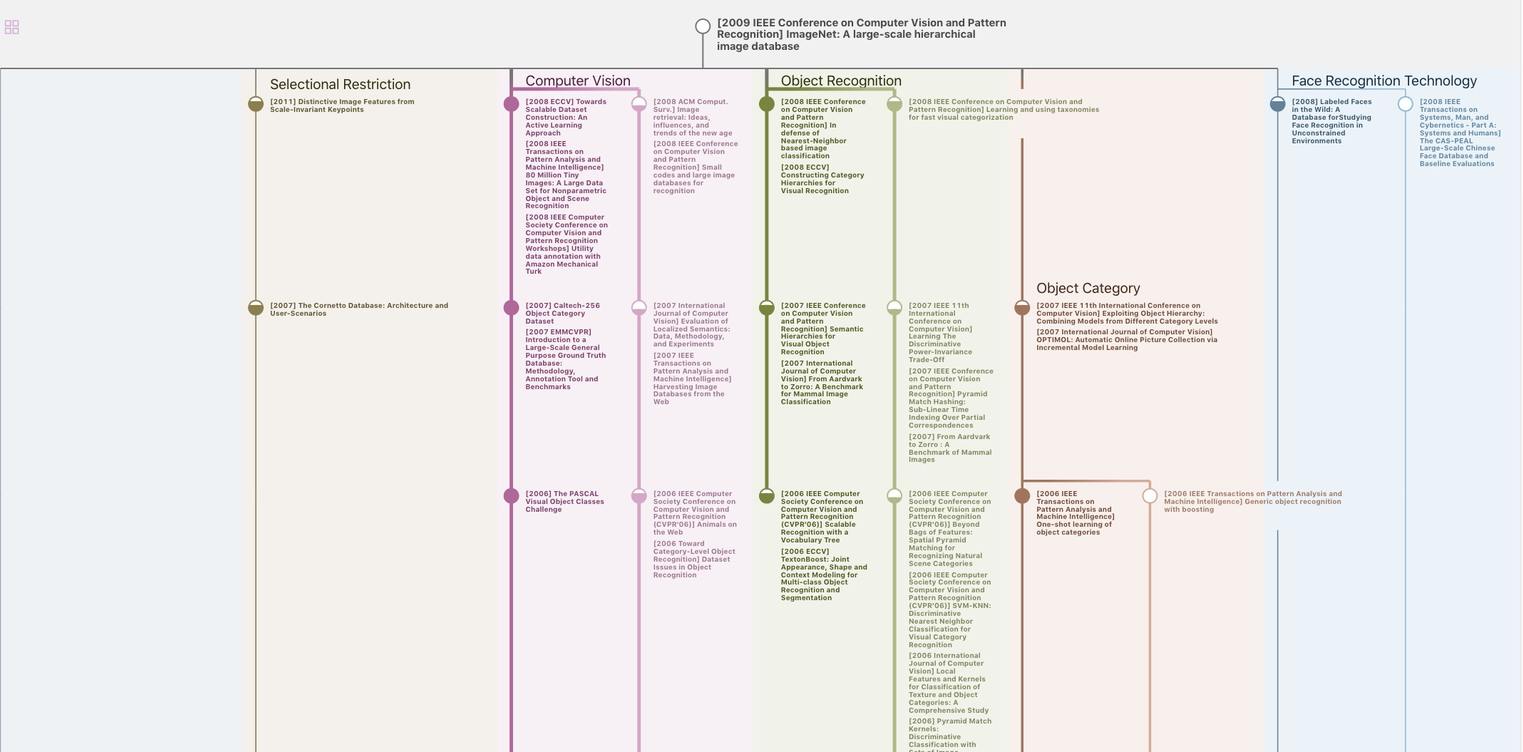
生成溯源树,研究论文发展脉络
Chat Paper
正在生成论文摘要