Multitask Deep Learning for Least-Squares Imaging: Seismic Reflectivity Inversion and Quantitative Error Analysis
IEEE Geoscience and Remote Sensing Letters(2023)
摘要
Least-squares migration (LSM) produces an accurate solution for subsurface reflectivity by solving a linear inverse problem, which can effectively mitigate the unbalanced subsurface illumination, suppress acquisition footprint, and improve image resolution. It can be implemented in the data or image domain. Data-domain LSM requires intensive computations for many times of Born modeling and adjoint migration. By contrast, image-domain LSM requires a large memory storage for calculating and saving the Hessian matrix. Due to the ability to describe sophisticated nonlinear relations, the neural network can be used to approximate the Hessian inverse by using the reverse time migration (RTM) image as the input and the true reflectivity model as the output. However, previous neural network-based LSMs mainly focused on the enhancement of image resolution and amplitude fidelity, but few on error and uncertainty analysis of network predicted images. We present a novel multitask deep learning method for the image-domain LSM to simultaneously estimate the Hessian inverse and evaluate kinematic and dynamic errors of network predicted results. We first use the RTM images along with migration velocity and source illumination as the network input and regard both the true reflectivity model and kinematic or dynamic error between predicted and labeled images as the output. Then, the L2-norm misfits of two terms are incorporated in the loss function to calculate the gradient to optimize the network trainable weights for producing an accurate LSM image. Numerical examples demonstrate that as the epoch of network training increases, the imaging accuracy of subsurface reflectors is gradually enhanced and the kinematic and dynamic errors of network predicted results are reduced, suggesting that the proposed multitask deep learning method can simultaneously predict high-quality LSM image and conduct quantitative error analysis.
更多查看译文
关键词
seismic reflectivity inversion,deep learning,imaging,multi-task,least-squares
AI 理解论文
溯源树
样例
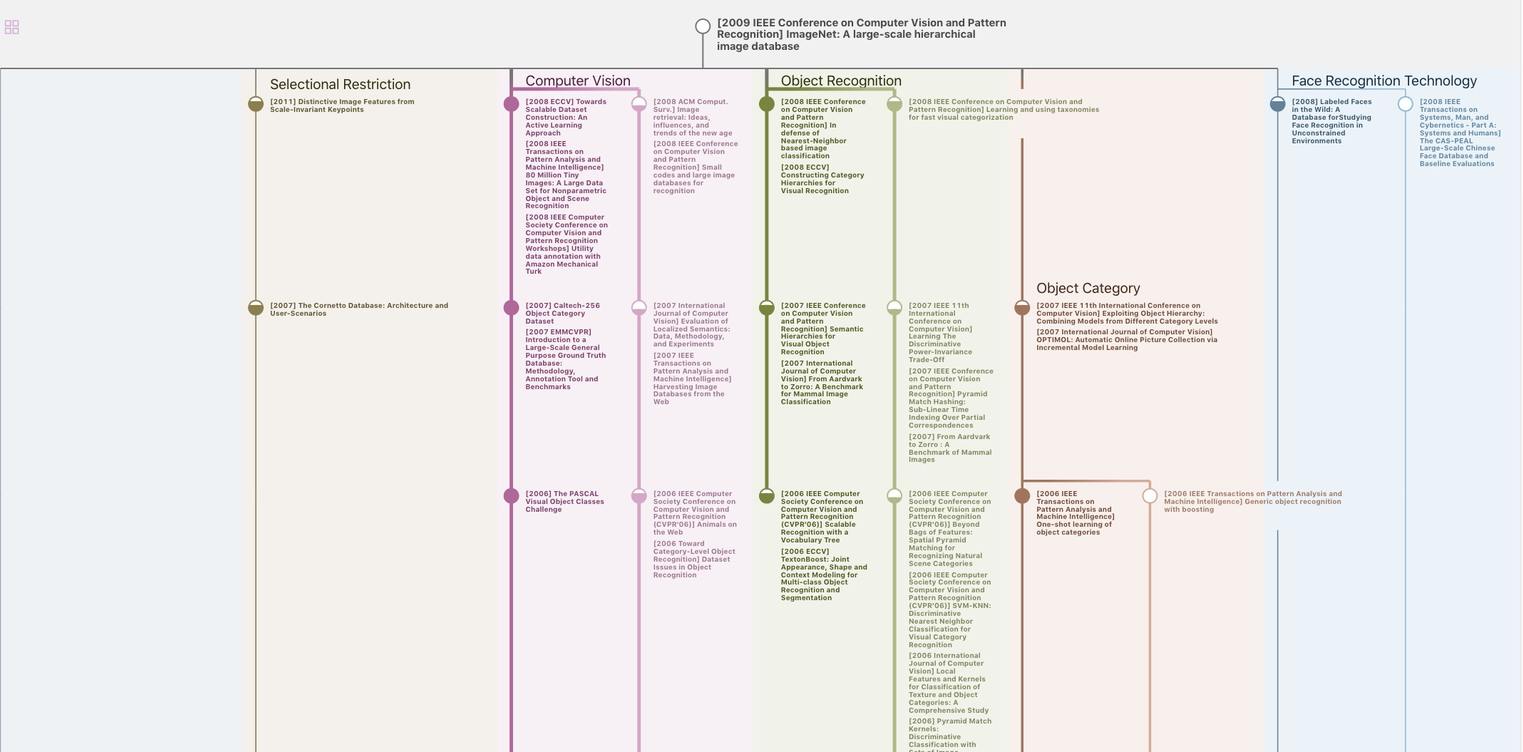
生成溯源树,研究论文发展脉络
Chat Paper
正在生成论文摘要