One-Bit Supervision for Image Classification: Problem, Solution, and Beyond
ACM TRANSACTIONS ON MULTIMEDIA COMPUTING COMMUNICATIONS AND APPLICATIONS(2024)
摘要
This article presents one-bit supervision, a novel setting of learning with fewer labels, for image classification. Instead of the training model using the accurate label of each sample, our setting requires the model to interact with the system by predicting the class label of each sample and learn from the answer whether the guess is correct, which provides one bit (yes or no) of information. An intriguing property of the setting is that the burden of annotation largely is alleviated in comparison to offering the accurate label. There are two keys to one-bit supervision: (i) improving the guess accuracy and (ii) making good use of the incorrect guesses. To achieve these goals, we propose a multi-stage training paradigm and incorporate negative label suppression into an off-the-shelf semi-supervised learning algorithm. Theoretical analysis shows that one-bit annotation is more efficient than full-bit annotation in most cases and gives the conditions of combining our approach with active learning. Inspired by this, we further integrate the one-bit supervision framework into the self-supervised learning algorithm, which yields an even more efficient training schedule. Different from training from scratch, when self-supervised learning is used for initialization, both hard example mining and class balance are verified to be effective in boosting the learning performance. However, these two frameworks still need full-bit labels in the initial stage. To cast off this burden, we utilize unsupervised domain adaptation to train the initial model and conduct pure one-bit annotations on the target dataset. In multiple benchmarks, the learning efficiency of the proposed approach surpasses that using full-bit, semi-supervised supervision.
更多查看译文
关键词
One-bit supervision,semi-supervised learning,active learning,self-supervised learning,unsupervised domain adaptation
AI 理解论文
溯源树
样例
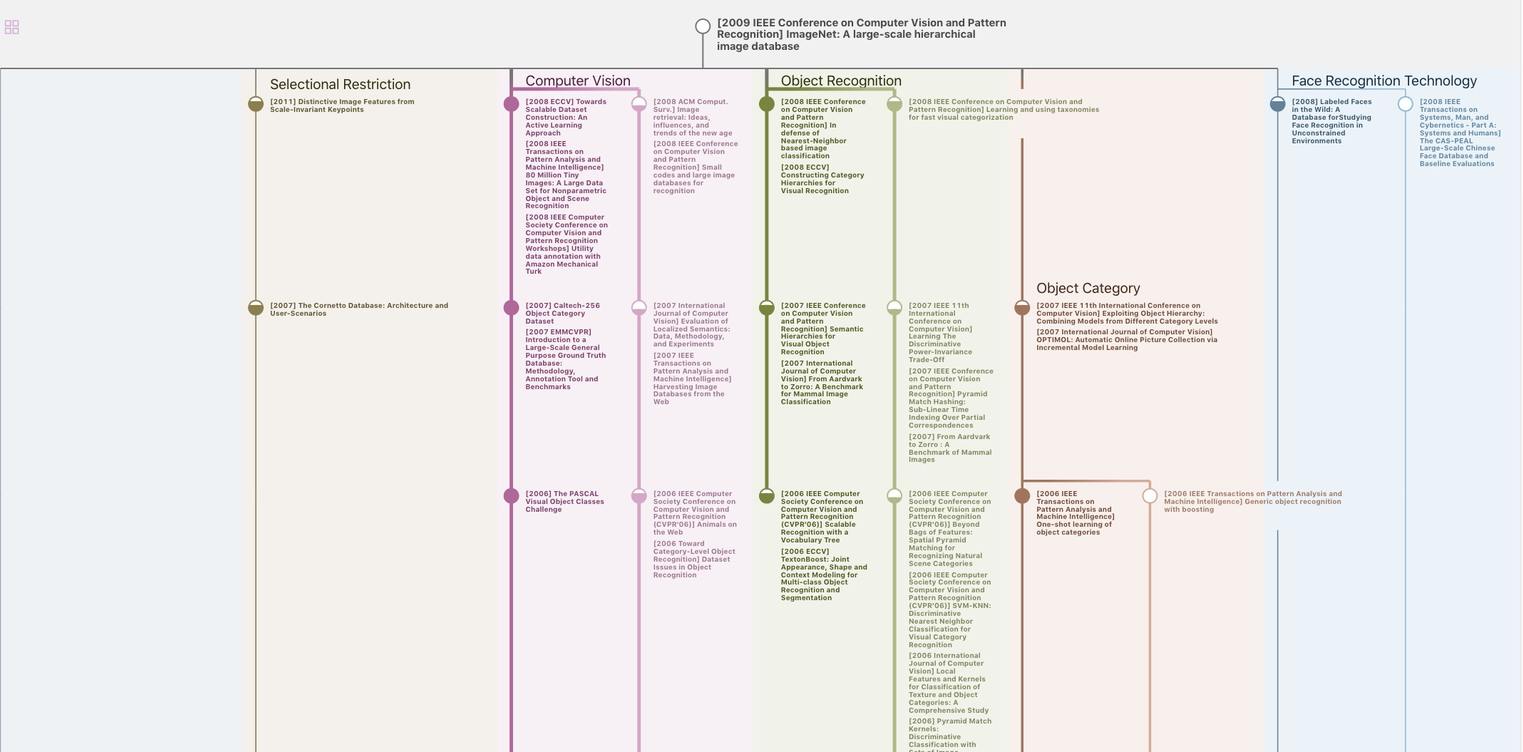
生成溯源树,研究论文发展脉络
Chat Paper
正在生成论文摘要