On the Complexity of Computing Sparse Equilibria and Lower Bounds for No-Regret Learning in Games.
CoRR(2023)
摘要
Characterizing the performance of no-regret dynamics in multi-player games is a foundational problem at the interface of online learning and game theory. Recent results have revealed that when all players adopt specific learning algorithms, it is possible to improve exponentially over what is predicted by the overly pessimistic no-regret framework in the traditional adversarial regime, thereby leading to faster convergence to the set of coarse correlated equilibria (CCE). Yet, despite considerable recent progress, the fundamental complexity barriers for learning in normal- and extensive-form games are poorly understood. In this paper, we make a step towards closing this gap by first showing that -- barring major complexity breakthroughs -- any polynomial-time learning algorithms in extensive-form games need at least $2^{\log^{1/2 - o(1)} |\mathcal{T}|}$ iterations for the average regret to reach below even an absolute constant, where $|\mathcal{T}|$ is the number of nodes in the game. This establishes a superpolynomial separation between no-regret learning in normal- and extensive-form games, as in the former class a logarithmic number of iterations suffices to achieve constant average regret. Furthermore, our results imply that algorithms such as multiplicative weights update, as well as its \emph{optimistic} counterpart, require at least $2^{(\log \log m)^{1/2 - o(1)}}$ iterations to attain an $O(1)$-CCE in $m$-action normal-form games. These are the first non-trivial -- and dimension-dependent -- lower bounds in that setting for the most well-studied algorithms in the literature. From a technical standpoint, we follow a beautiful connection recently made by Foster, Golowich, and Kakade (ICML '23) between sparse CCE and Nash equilibria in the context of Markov games. Consequently, our lower bounds rule out polynomial-time algorithms well beyond the traditional online learning framework.
更多查看译文
AI 理解论文
溯源树
样例
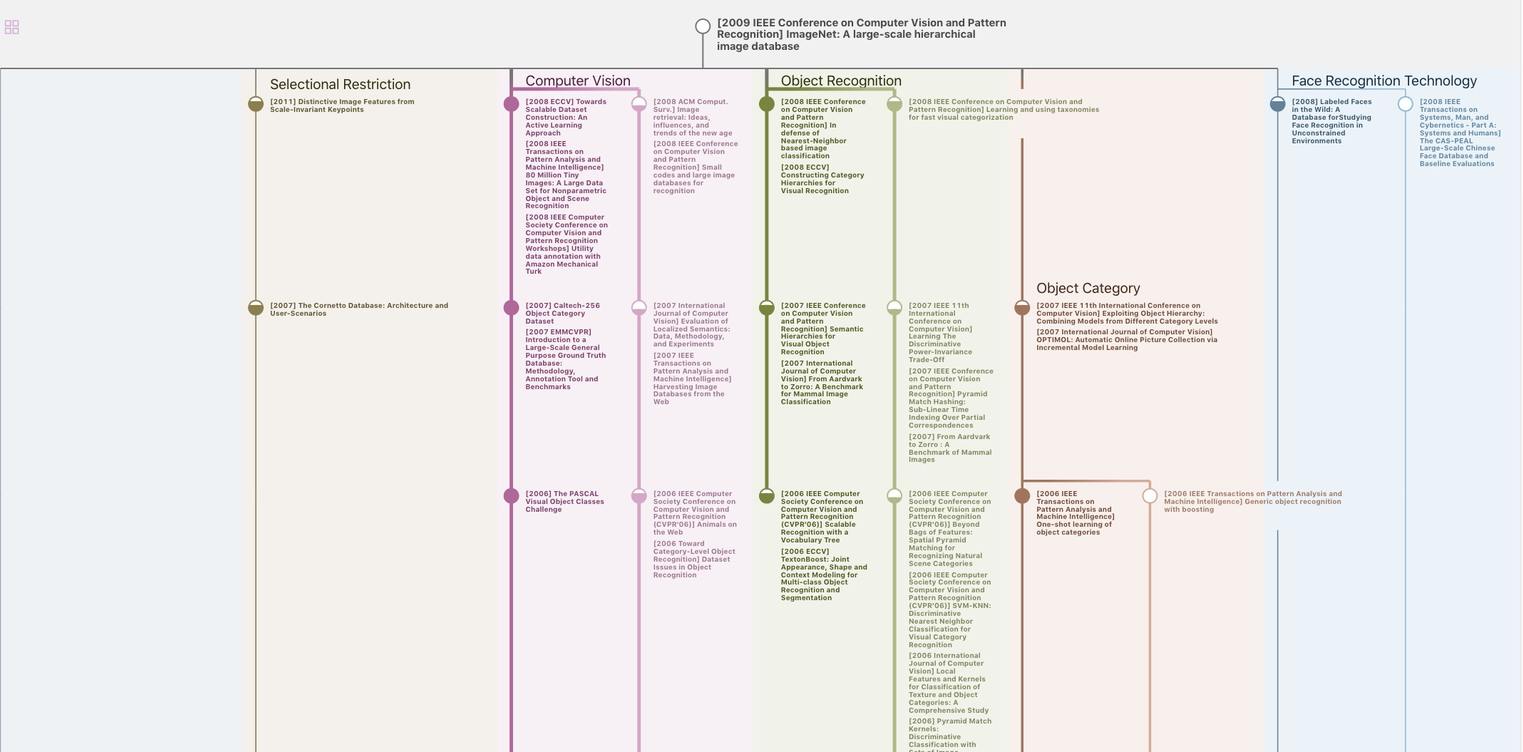
生成溯源树,研究论文发展脉络
Chat Paper
正在生成论文摘要