Generalized Quantum Convolution for Multidimensional Data
Entropy(2023)
摘要
The convolution operation plays a vital role in a wide range of critical algorithms across various domains, such as digital image processing, convolutional neural networks, and quantum machine learning. In existing implementations, particularly in quantum neural networks, convolution operations are usually approximated by the application of filters with data strides that are equal to the filter window sizes. One challenge with these implementations is preserving the spatial and temporal localities of the input features, specifically for data with higher dimensions. In addition, the deep circuits required to perform quantum convolution with a unity stride, especially for multidimensional data, increase the risk of violating decoherence constraints. In this work, we propose depth-optimized circuits for performing generalized multidimensional quantum convolution operations with unity stride targeting applications that process data with high dimensions, such as hyperspectral imagery and remote sensing. We experimentally evaluate and demonstrate the applicability of the proposed techniques by using real-world, high-resolution, multidimensional image data on a state-of-the-art quantum simulator from IBM Quantum.
更多查看译文
关键词
convolution,quantum algorithms,quantum image processing,quantum computing
AI 理解论文
溯源树
样例
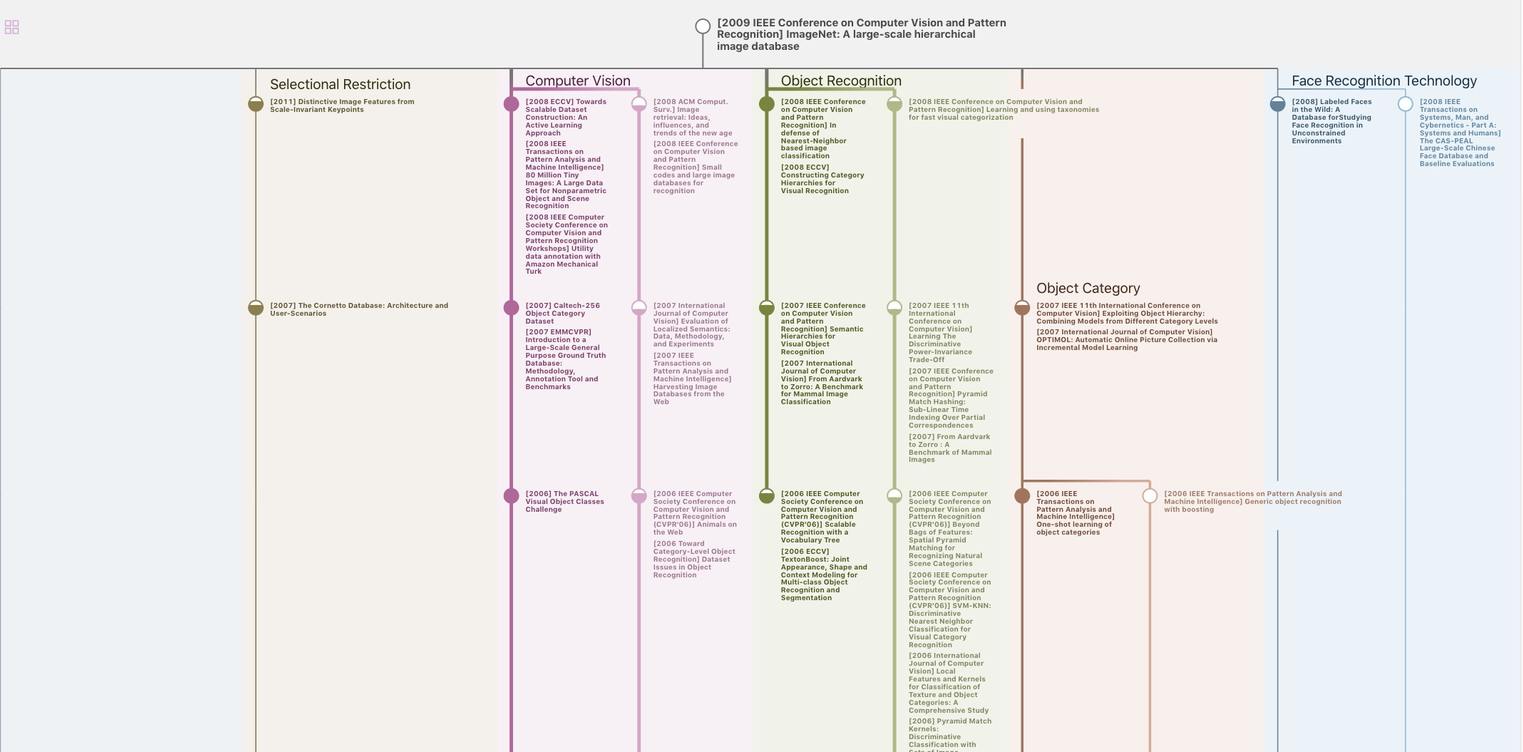
生成溯源树,研究论文发展脉络
Chat Paper
正在生成论文摘要