EFP-Net: A Novel Building Change Detection Method Based on Efficient Feature Fusion and Foreground Perception
REMOTE SENSING(2023)
摘要
Over the past decade, deep learning techniques have significantly advanced the field of building change detection in remote sensing imagery. However, existing deep learning-based approaches often encounter limitations in complex remote sensing scenarios, resulting in false detections and detail loss. This paper introduces EFP-Net, a novel building change detection approach that resolves the mentioned issues by utilizing effective feature fusion and foreground perception. EFP-Net comprises three main modules, the feature extraction module (FEM), the spatial-temporal correlation module (STCM), and the residual guidance module (RGM), which jointly enhance the fusion of bi-temporal features and hierarchical features. Specifically, the STCM utilizes the temporal change duality prior and multi-scale perception to augment the 3D convolution modeling capability for bi-temporal feature variations. Additionally, the RGM employs the higher-layer prediction map to guide shallow layer features, reducing the introduction of noise during the hierarchical feature fusion process. Furthermore, a dynamic Focal loss with foreground awareness is developed to mitigate the class imbalance problem. Extensive experiments on the widely adopted WHU-BCD, LEVIR-CD, and CDD datasets demonstrate that the proposed EFP-Net is capable of significantly improving accuracy in building change detection.
更多查看译文
关键词
building change detection,deep learning,feature fusion,remote sensing imagery
AI 理解论文
溯源树
样例
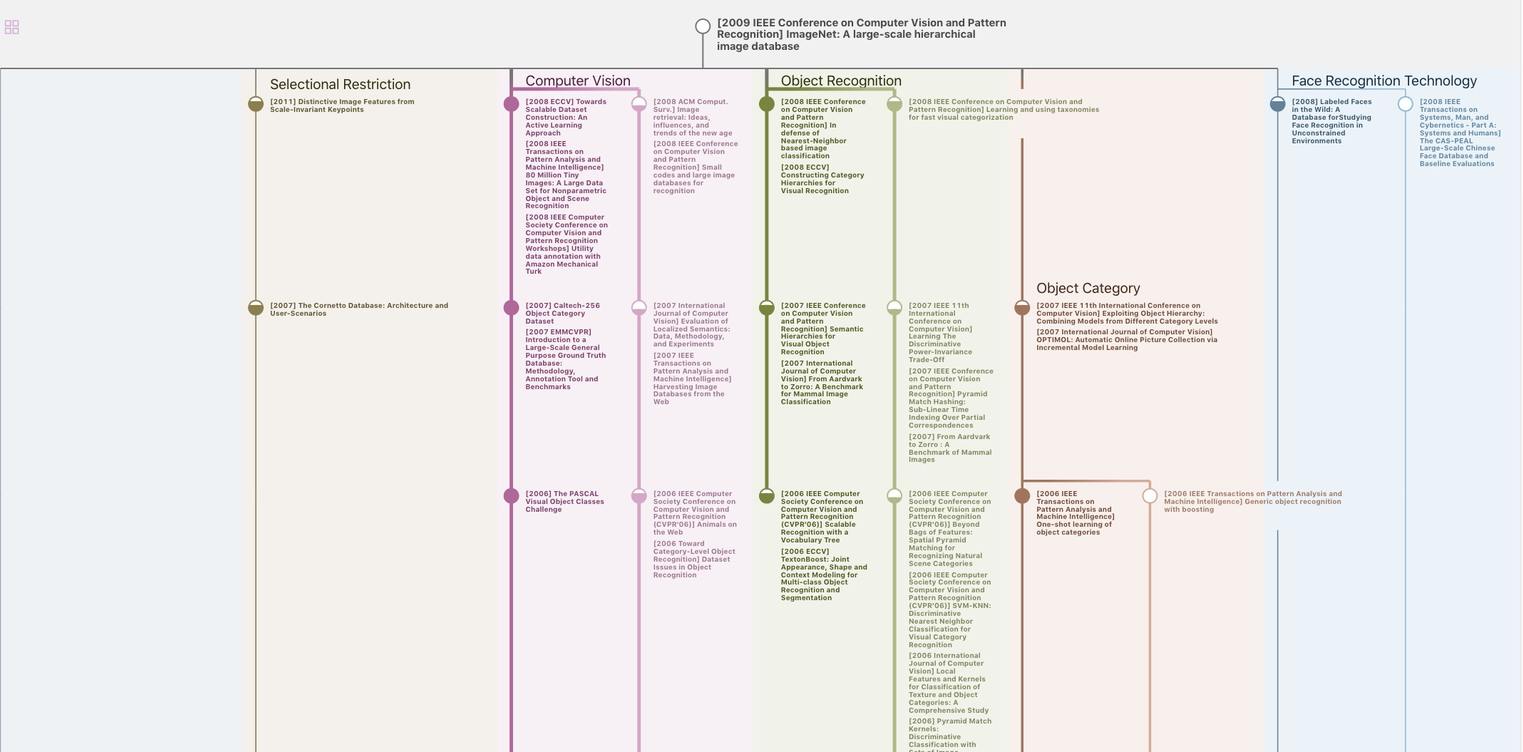
生成溯源树,研究论文发展脉络
Chat Paper
正在生成论文摘要