eSVD-DE: cohort-wide differential expression in single-cell RNA-seq data using exponential-family embeddings
BMC Bioinformatics(2024)
摘要
Single-cell RNA-sequencing (scRNA) datasets are becoming increasingly popular in clinical and cohort studies, but there is a lack of methods to investigate differentially expressed (DE) genes among such datasets with numerous individuals. While numerous methods exist to find DE genes for scRNA data from limited individuals, differential-expression testing for large cohorts of case and control individuals using scRNA data poses unique challenges due to substantial effects of human variation, i.e., individual-level confounding covariates that are difficult to account for in the presence of sparsely-observed genes. We develop the eSVD-DE, a matrix factorization that pools information across genes and removes confounding covariate effects, followed by a novel two-sample test in mean expression between case and control individuals. In general, differential testing after dimension reduction yields an inflation of Type-1 errors. However, we overcome this by testing for differences between the case and control individuals’ posterior mean distributions via a hierarchical model. In previously published datasets of various biological systems, eSVD-DE has more accuracy and power compared to other DE methods typically repurposed for analyzing cohort-wide differential expression. eSVD-DE proposes a novel and powerful way to test for DE genes among cohorts after performing a dimension reduction. Accurate identification of differential expression on the individual level, instead of the cell level, is important for linking scRNA-seq studies to our understanding of the human population.
更多查看译文
关键词
Case–control subjects,Gamma–Poisson distribution,Matrix factorization,Multi-individual data
AI 理解论文
溯源树
样例
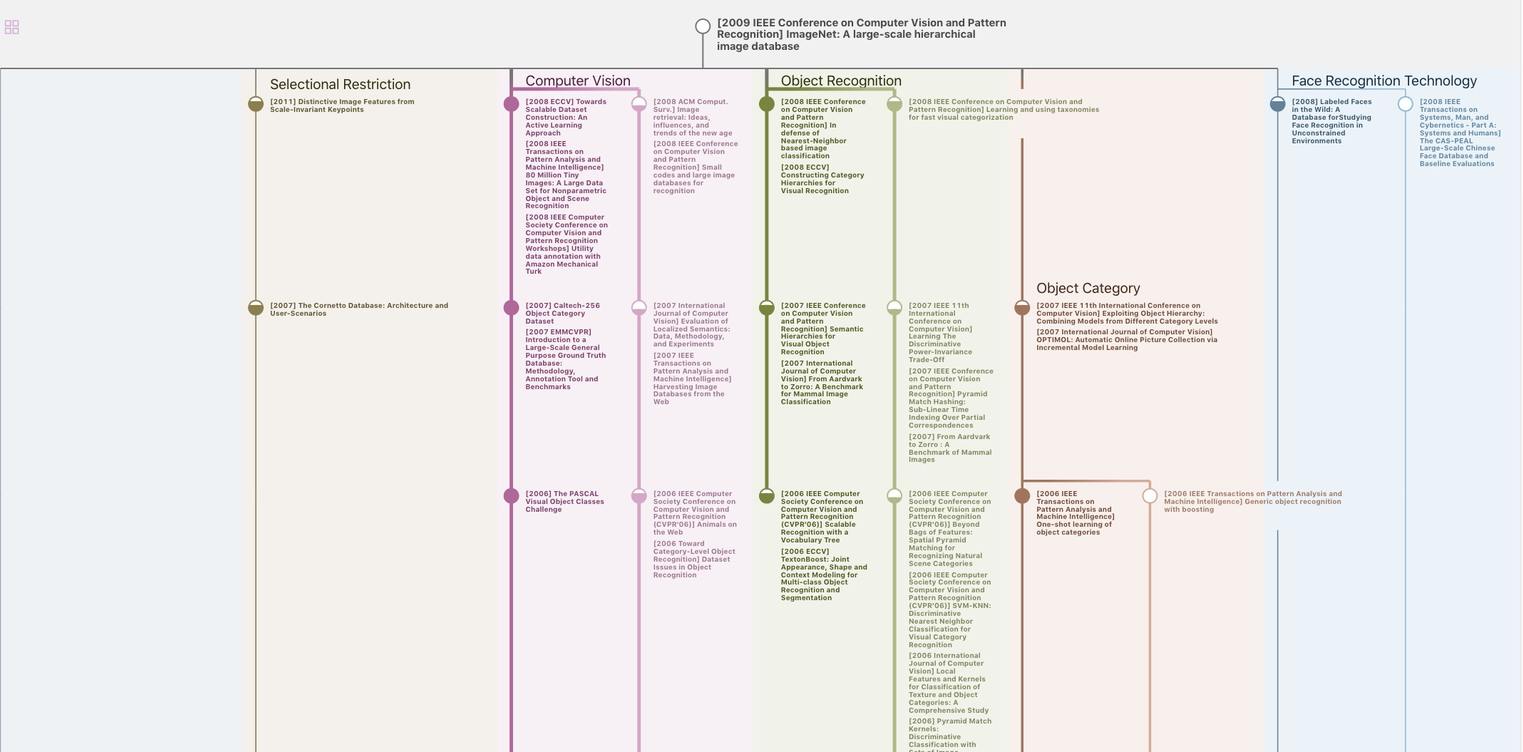
生成溯源树,研究论文发展脉络
Chat Paper
正在生成论文摘要