Deep One-Class Fine-Tuning for Imbalanced Short Text Classification in Transfer Learning.
Advanced Data Mining and Applications: 19th International Conference, ADMA 2023, Shenyang, China, August 21–23, 2023, Proceedings, Part I(2023)
摘要
The abundance of user-generated online content has presented significant challenges in handling big data. One challenge involves analyzing short posts on social media, ranging from sentiment identification to abusive content detection. Despite recent advancements in pre-trained language models and transfer learning for textual data analysis, the classification performance is hindered by imbalanced data, where anomalous data represents only a small portion of the dataset. To address this, we propose Deep One-Class Fine-Tuning (DOCFT), a versatile method for fine-tuning transfer learning-based textual classifiers. DOCFT uses a one-class SVM-style hyperplane to encapsulate anomalous data. This approach involves a two-step fine-tuning process and utilizes an alternating optimization method based on a custom OC-SVM loss function and quantile regression. Through evaluations on four different hate-speech datasets, we observe that significant performance improvements can be achieved by our method.
更多查看译文
关键词
imbalanced short text classification,transfer learning,one-class,fine-tuning
AI 理解论文
溯源树
样例
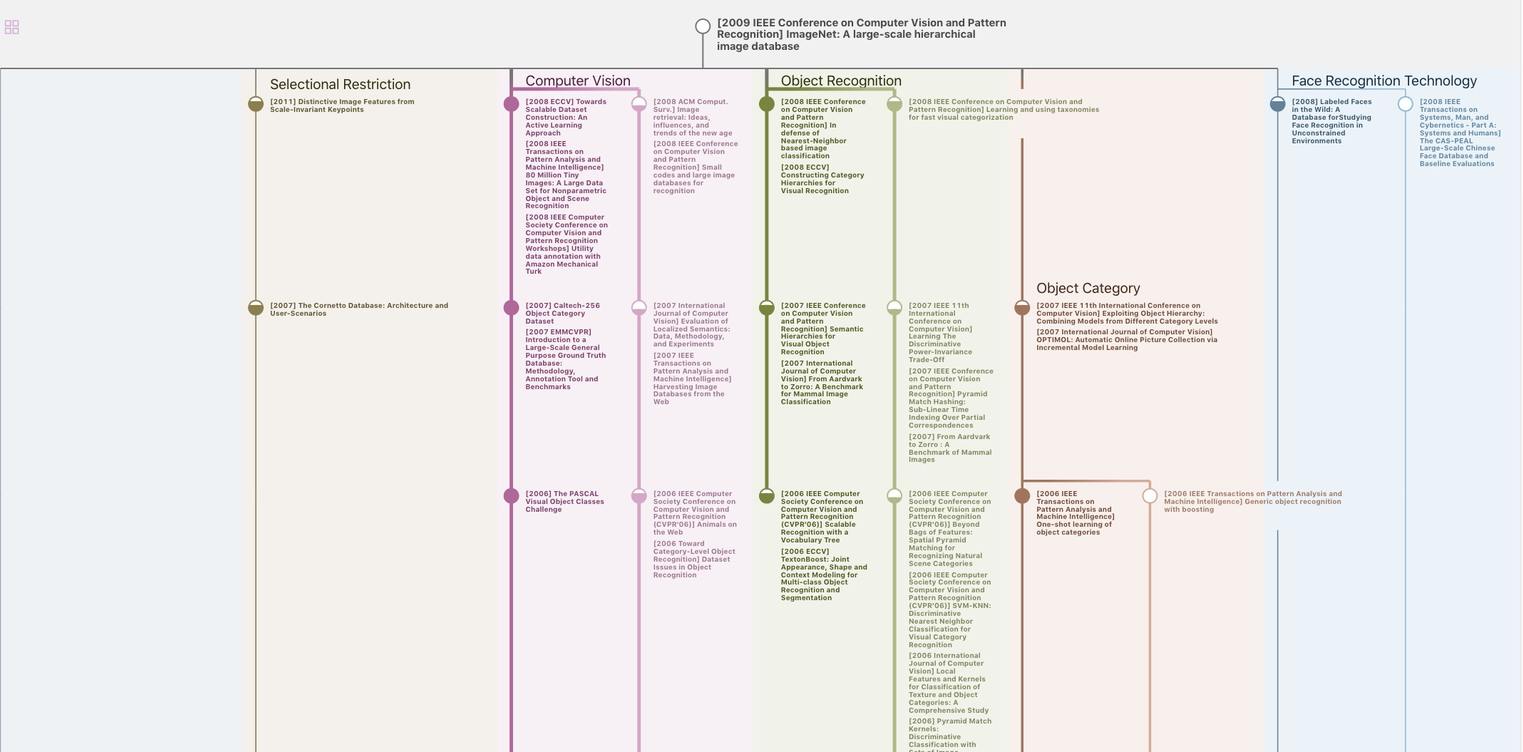
生成溯源树,研究论文发展脉络
Chat Paper
正在生成论文摘要